CERAD-NAB and flexible battery based neuropsychological differentiation of Alzheimer's dementia and depression using machine learning approaches
AGING NEUROPSYCHOLOGY AND COGNITION(2024)
摘要
Depression (DEP) and dementia of the Alzheimer's type (DAT) represent the most common neuropsychiatric disorders in elderly patients. Accurate differential diagnosis is indispensable to ensure appropriate treatment. However, DEP can yet mimic cognitive symptoms of DAT and patients with DAT often also present with depressive symptoms, impeding correct diagnosis. Machine learning (ML) approaches could eventually improve this discrimination using neuropsychological test data, but evidence is still missing. We therefore employed Support Vector Machine (SVM), Naive Bayes (NB), Random Forest (RF) and conventional Logistic Regression (LR) to retrospectively predict the diagnoses of 189 elderly patients (68 DEP and 121 DAT) based on either the well-established Consortium to Establish a Registry for Alzheimer's Disease neuropsychological assessment battery (CERAD-NAB) or a flexible battery approach (FLEXBAT). The best performing combination consisted of FLEXBAT and NB, correctly classifying 87.0% of patients as either DAT or DEP. However, all accuracies were similar across algorithms and test batteries (83.0% - 87.0%). Accordingly, our study is the first to show that common ML algorithms with their default parameters can accurately differentiate between patients clinically diagnosed with DAT or DEP using neuropsychological test data, but do not necessarily outperform conventional LR.
更多查看译文
关键词
Alzheimer's dementia,depression,neuropsychological assessment,machine learning,differential diagnosis,CERAD-NAB,flexible battery approach
AI 理解论文
溯源树
样例
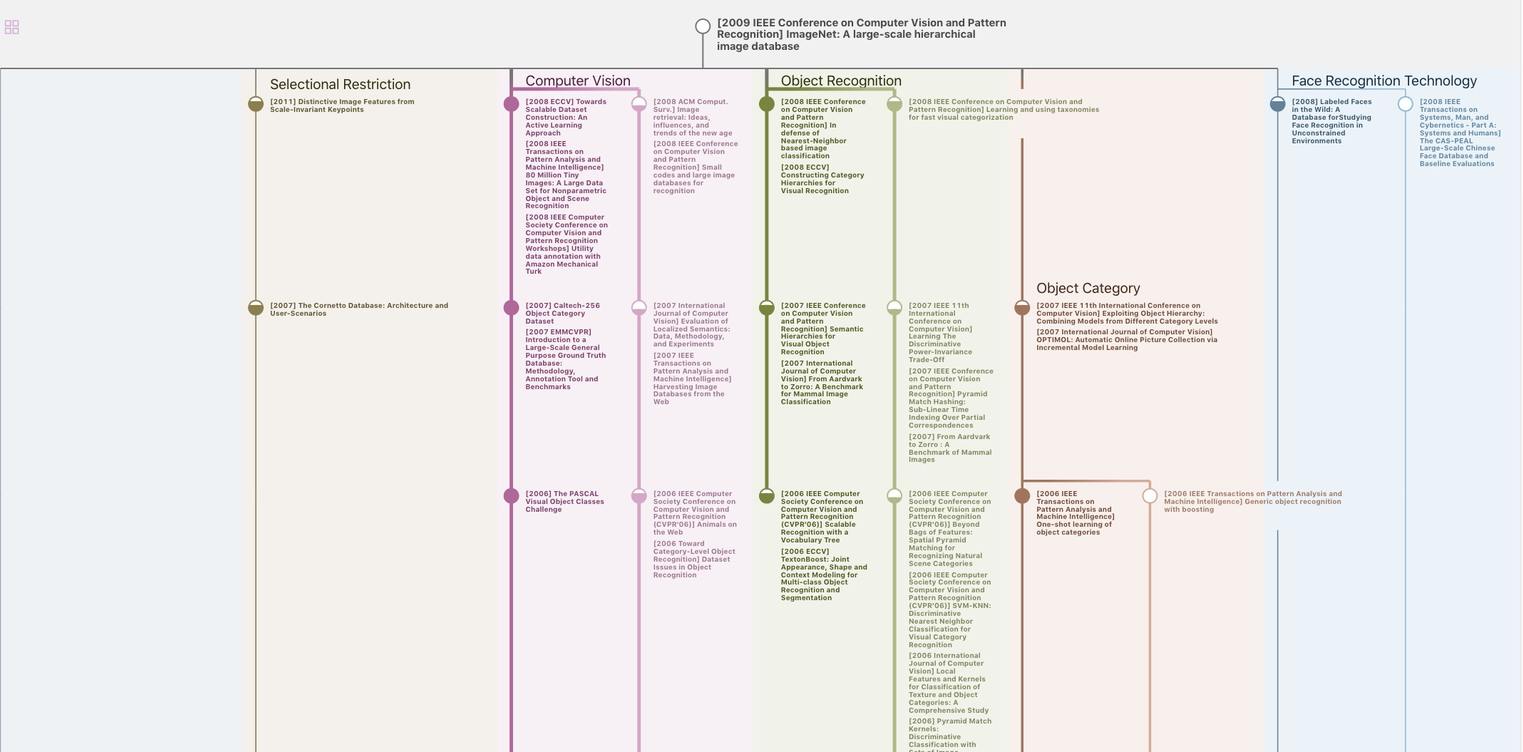
生成溯源树,研究论文发展脉络
Chat Paper
正在生成论文摘要