An Overview of the Latest Machine Learning Trends in Short-Term Load Forecasting
2022 IEEE International Conference on Environment and Electrical Engineering and 2022 IEEE Industrial and Commercial Power Systems Europe (EEEIC / I&CPS Europe)(2022)
摘要
The role of electricity in our daily lives has been steadily growing, and the socioeconomic data shows an indisputable link between the flourishing of modern societies and the expansion of its electric power systems infrastructure. The 21st century brought an increased awareness of global warming as a serious threat, which is deeply rooted in the prolonged greenhouse emissions. This prompted policy makers and societies to adopt several transformations that, in turn, result in a continuously challenging environment. In face of this scenario, short-term load forecasting (STLF) continues to be a crucial task for a better decision-making, not only for the System Operator, but also for market participants, distributors, and retailers. In addition, the proposal of more disaggregated load forecasting has been a new spark to this field, much due to the progress of smart-grids projects, where the implementation of self-consumption, load-scheduling and demand-response requires an estimated knowledge of the load consumption. The STLF literature offers a comprehensive variety of different methods to perform the prediction task. Currently, machine-learning (ML) based methods are the predominant choice to build forecasters, primarily due to their capability to deal with complex load patterns. This study aims to characterize this rich framework, by briefly delving into the working principle, architecture, and other performance issues, that help contextualize the cherry-picked influential contributions. These comprise the main ML-based STLF methods. A discussion of the strengths and weaknesses of each method is provided in the end, resulting in a useful overview and guidance for future research work.
更多查看译文
关键词
Load Forecasting,Short-Term Load Forecasting,Prediction Models,Neural Networks,Machine Learning and Deep Learning
AI 理解论文
溯源树
样例
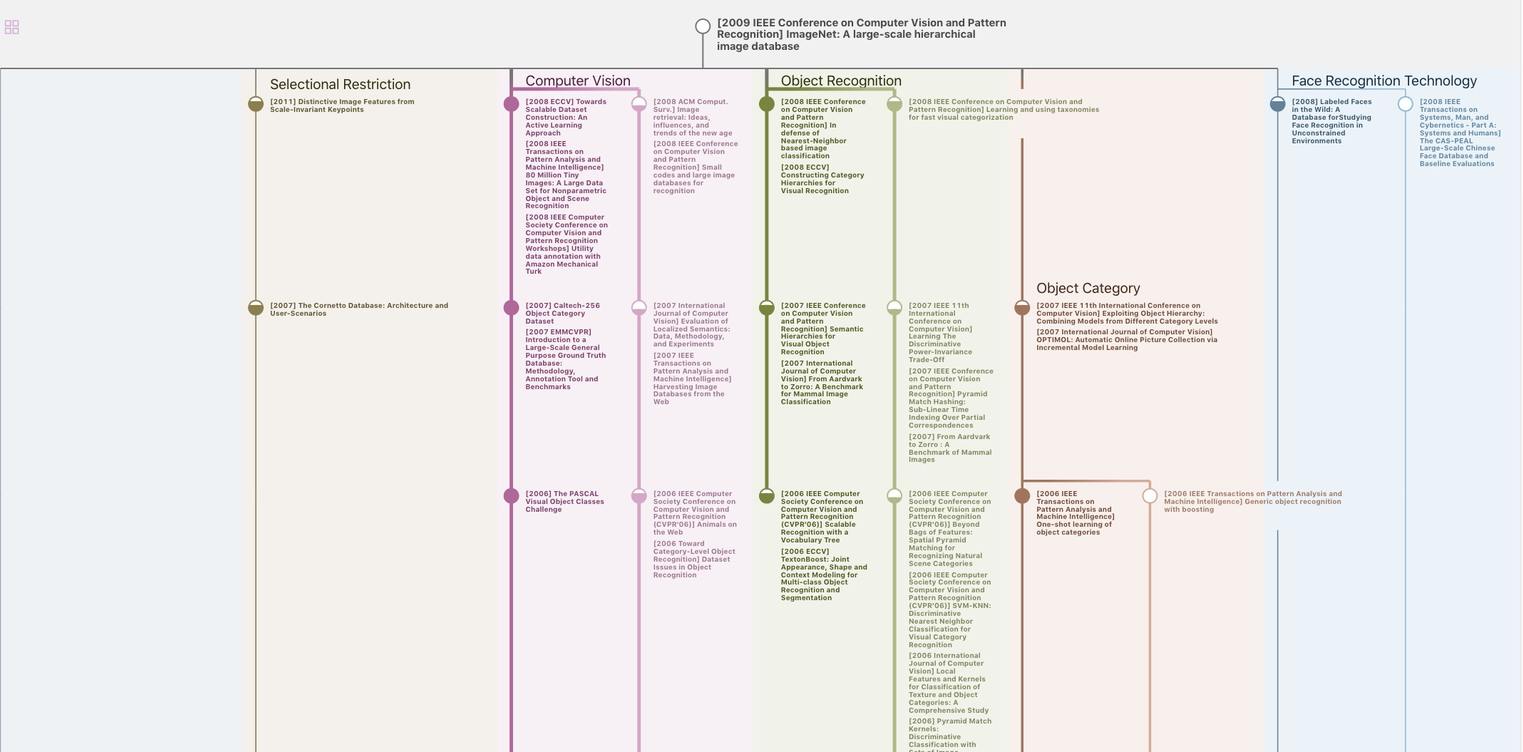
生成溯源树,研究论文发展脉络
Chat Paper
正在生成论文摘要