Towards Zero-Shot Code-Switched Speech Recognition
arxiv(2022)
摘要
In this work, we seek to build effective code-switched (CS) automatic speech recognition systems (ASR) under the zero-shot setting where no transcribed CS speech data is available for training. Previously proposed frameworks which conditionally factorize the bilingual task into its constituent monolingual parts are a promising starting point for leveraging monolingual data efficiently. However, these methods require the monolingual modules to perform language segmentation. That is, each monolingual module has to simultaneously detect CS points and transcribe speech segments of one language while ignoring those of other languages -- not a trivial task. We propose to simplify each monolingual module by allowing them to transcribe all speech segments indiscriminately with a monolingual script (i.e. transliteration). This simple modification passes the responsibility of CS point detection to subsequent bilingual modules which determine the final output by considering multiple monolingual transliterations along with external language model information. We apply this transliteration-based approach in an end-to-end differentiable neural network and demonstrate its efficacy for zero-shot CS ASR on Mandarin-English SEAME test sets.
更多查看译文
关键词
code-switched ASR,zero-shot ASR,CTC
AI 理解论文
溯源树
样例
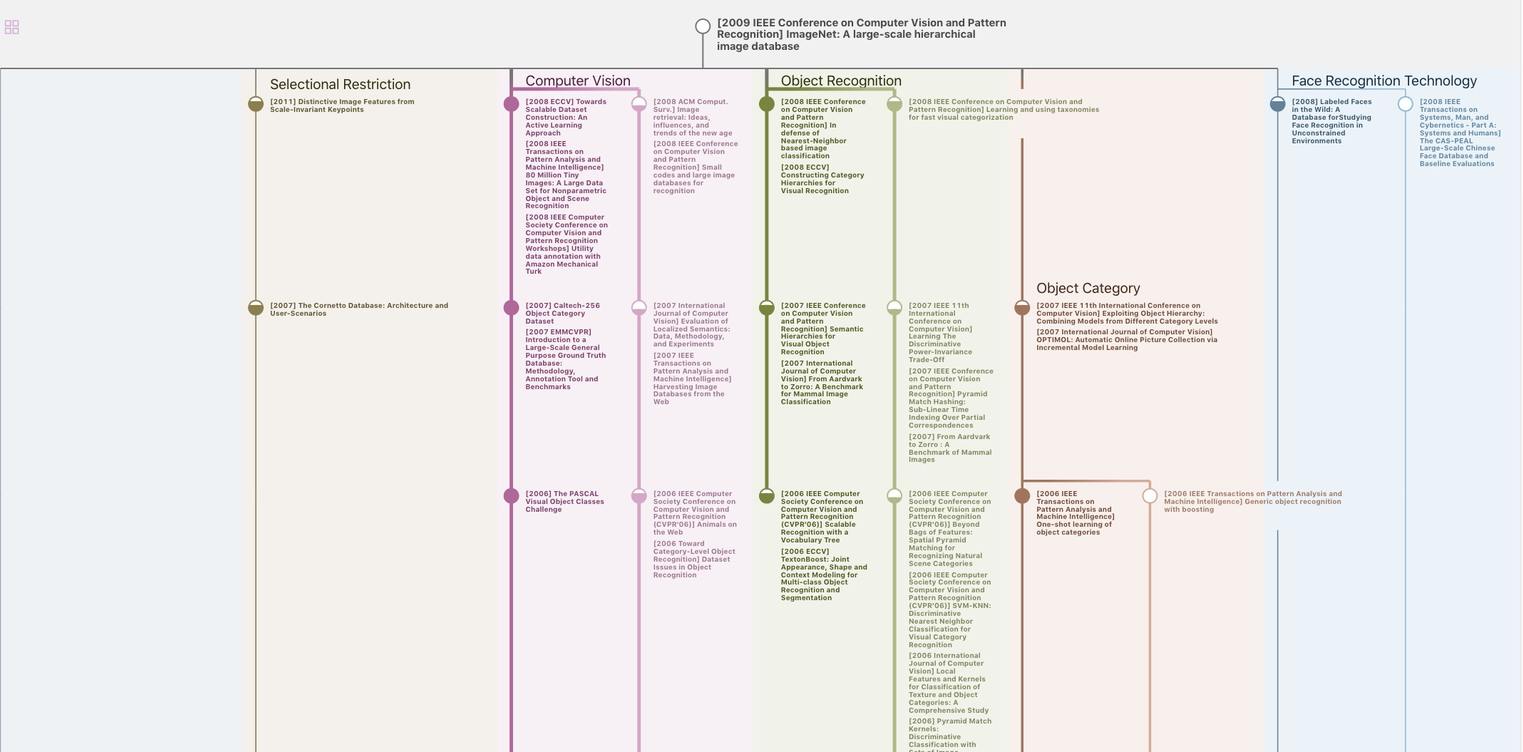
生成溯源树,研究论文发展脉络
Chat Paper
正在生成论文摘要