Cutting Through the Noise: An Empirical Comparison of Psychoacoustic and Envelope-based Features for Machinery Fault Detection
arxiv(2023)
摘要
Acoustic-based fault detection has a high potential to monitor the health condition of mechanical parts. However, the background noise of an industrial environment may negatively influence the performance of fault detection. Limited attention has been paid to improving the robustness of fault detection against industrial environmental noise. Therefore, we present the Lenze production background-noise (LPBN) real-world dataset and an automated and noise-robust auditory inspection (ARAI) system for the end-of-line inspection of geared motors. An acoustic array is used to acquire data from motors with a minor fault, major fault, or which are healthy. A benchmark is provided to compare the psychoacoustic features with different types of envelope features based on expert knowledge of the gearbox. To the best of our knowledge, we are the first to apply time-varying psychoacoustic features for fault detection. We train a state-of-the-art one-class-classifier, on samples from healthy motors and separate the faulty ones for fault detection using a threshold. The best-performing approaches achieve an area under curve of 0.87 (logarithm envelope), 0.86 (time-varying psychoacoustics), and 0.91 (combination of both).
更多查看译文
关键词
machinery fault detection,noise,features,psycho-acoustic,envelope-based
AI 理解论文
溯源树
样例
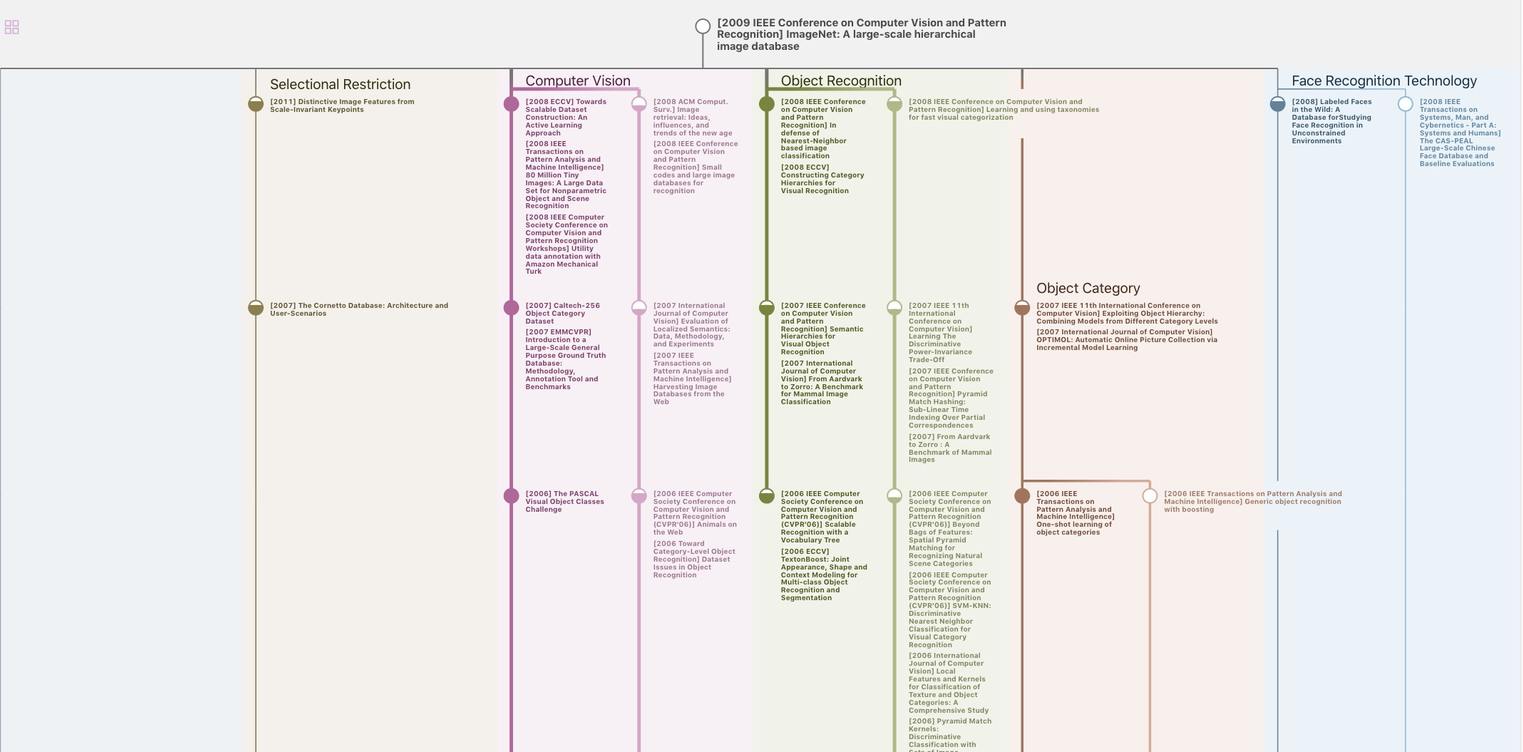
生成溯源树,研究论文发展脉络
Chat Paper
正在生成论文摘要