Inverse Scaling Can Become U-Shaped.
arxiv(2023)
摘要
Scaling up language models has been empirically shown to improve performance and unlock emergent abilities. Conversely, observing worse performance as a function of scale ("inverse scaling") would indicate that scaling encourages behaviors that are misaligned with human preferences. The Inverse Scaling Prize (McKenzie et al. 2022) identified eleven such inverse scaling tasks, evaluated on models of up to 280B parameters and up to 500 zettaFLOPs of training compute. This paper takes a closer look at these inverse scaling tasks. We evaluate models of up to 540B parameters, trained on five times more compute than those evaluated in the Inverse Scaling Prize. With this increased range of model sizes and training compute, only four out of the eleven tasks remain inverse scaling. Six out of the eleven tasks exhibit what we call "U-shaped scaling" -- performance decreases up to a certain model size, and then increases again up to the largest model evaluated (the one remaining task displays positive scaling). U-shaped scaling suggests that the inverse scaling trend observed in McKenzie et al. (2022) may not continue to hold for larger models, and adds further support to the claim that sufficiently large models unlock emergent abilities.
更多查看译文
关键词
inverse scaling,u-shaped
AI 理解论文
溯源树
样例
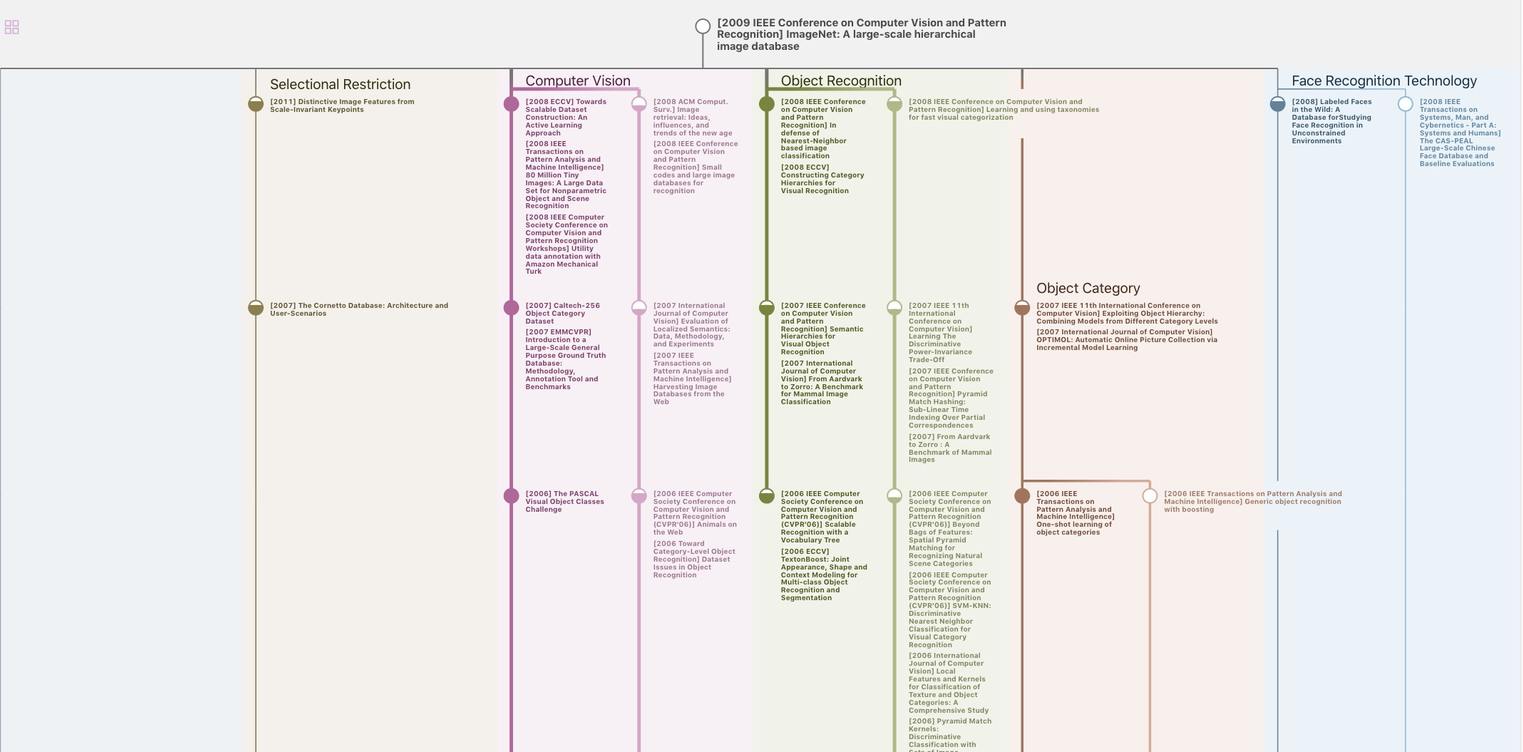
生成溯源树,研究论文发展脉络
Chat Paper
正在生成论文摘要