Faber: A Hardware/SoftWare Toolchain for Image Registration
IEEE Transactions on Parallel and Distributed Systems (TPDS)(2023)CCF ASCI 2区
Politecn Milan
Abstract
Image registration is a well-defined computation paradigm widely applied to align one or more images to a target image. This paradigm, which builds upon three main components, is particularly compute-intensive and represents many image processing pipelines’ bottlenecks. State-of-the-art solutions leverage hardware acceleration to speed up image registration, but they are usually limited to implementing a single component. We present Faber, an open-source HW/SW CAD toolchain tailored to image registration. The Faber toolchain comprises HW/SW highly-tunable registration components, supports users with different expertise in building custom pipelines, and automates the design process. In this direction, Faber provides both default settings for entry-level users and latency and resource models to guide HW experts in customizing the different components. Finally, Faber achieves from 1.5× to 54× in speedup and from 2× to 177× in energy efficiency against state-of-the-art tools on a Xeon Gold.
MoreTranslated text
Key words
Measurement,Pipelines,Image registration,Field programmable gate arrays,Optimization,Graphics processing units,Registers,FPGAs,HW,SW design automation,image registration
PDF
View via Publisher
AI Read Science
AI Summary
AI Summary is the key point extracted automatically understanding the full text of the paper, including the background, methods, results, conclusions, icons and other key content, so that you can get the outline of the paper at a glance.
Example
Background
Key content
Introduction
Methods
Results
Related work
Fund
Key content
- Pretraining has recently greatly promoted the development of natural language processing (NLP)
- We show that M6 outperforms the baselines in multimodal downstream tasks, and the large M6 with 10 parameters can reach a better performance
- We propose a method called M6 that is able to process information of multiple modalities and perform both single-modal and cross-modal understanding and generation
- The model is scaled to large model with 10 billion parameters with sophisticated deployment, and the 10 -parameter M6-large is the largest pretrained model in Chinese
- Experimental results show that our proposed M6 outperforms the baseline in a number of downstream tasks concerning both single modality and multiple modalities We will continue the pretraining of extremely large models by increasing data to explore the limit of its performance
Try using models to generate summary,it takes about 60s
Must-Reading Tree
Example
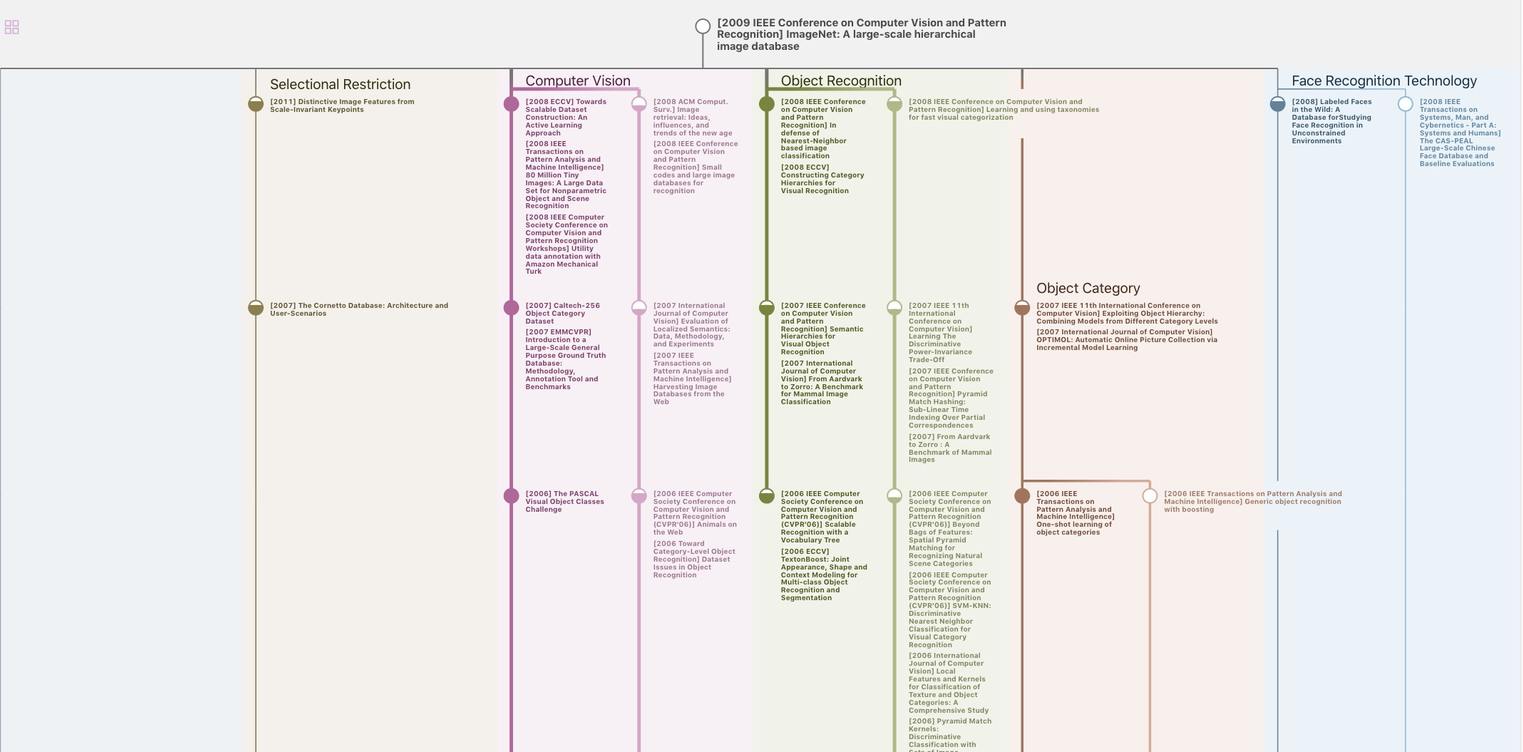
Generate MRT to find the research sequence of this paper
Related Papers
Hephaestus: Codesigning and Automating 3D Image Registration on Reconfigurable Architectures
ACM TRANSACTIONS ON EMBEDDED COMPUTING SYSTEMS 2023
被引用2
Starlight: A Kernel Optimizer for GPU Processing
JOURNAL OF PARALLEL AND DISTRIBUTED COMPUTING 2024
被引用0
Data Disclaimer
The page data are from open Internet sources, cooperative publishers and automatic analysis results through AI technology. We do not make any commitments and guarantees for the validity, accuracy, correctness, reliability, completeness and timeliness of the page data. If you have any questions, please contact us by email: report@aminer.cn
Chat Paper
GPU is busy, summary generation fails
Rerequest