Recursive Estimation of Multichannel Autoregressive Processes in Correlated Noise With Joint Instrumental Variable and Bias Compensation
IEEE TRANSACTIONS ON INSTRUMENTATION AND MEASUREMENT(2022)
摘要
This article proposes a new recursive algorithm for estimating multichannel autoregressive (AR) processes in spatially correlated noise using joint extended instrumental variable (EIV) and bias compensation (BC). A weighted least squares (WLS) solution for solving the Yule-Walker (YW) equations from EIV and BC is proposed, while the noise covariance parameters is estimated via the least-squares method. A splitting approach is proposed for solving this WLS problem based on matrix splitting, and it is further extended to a recursive algorithm, which can be solved via the variable forgetting factor (VFF)-EIV algorithm. A robust smoothing method for estimating the noise parameters and an adaptive model selection method for controlling the BC contribution are proposed for reducing the estimation variance. The validity of the proposed algorithm is evaluated using both synthetic and real data. The experimental results show that the proposed VFF-EIV-BC (EBC) algorithm gives higher accuracy than conventional recursive least squares (RLS) algorithm and the VFF-EIV method. It also provides faster tracking speed under sudden changes. The proposed method is applied to the brain-computer interface (BCI) competition IV-2b EEG dataset on motor imagery tasks for spectrum and effective connectivity estimation. The high-alpha and beta event-related desynchronization associated with the tasks can be clearly revealed. It was also applied to an auditory odd-ball data from the Open-fMRI database.
更多查看译文
关键词
Bias compensation (BC), effective connectivity, electroencephalogram, extended instrumental variable (EIV), time-varying multichannel autoregressive (AR) model
AI 理解论文
溯源树
样例
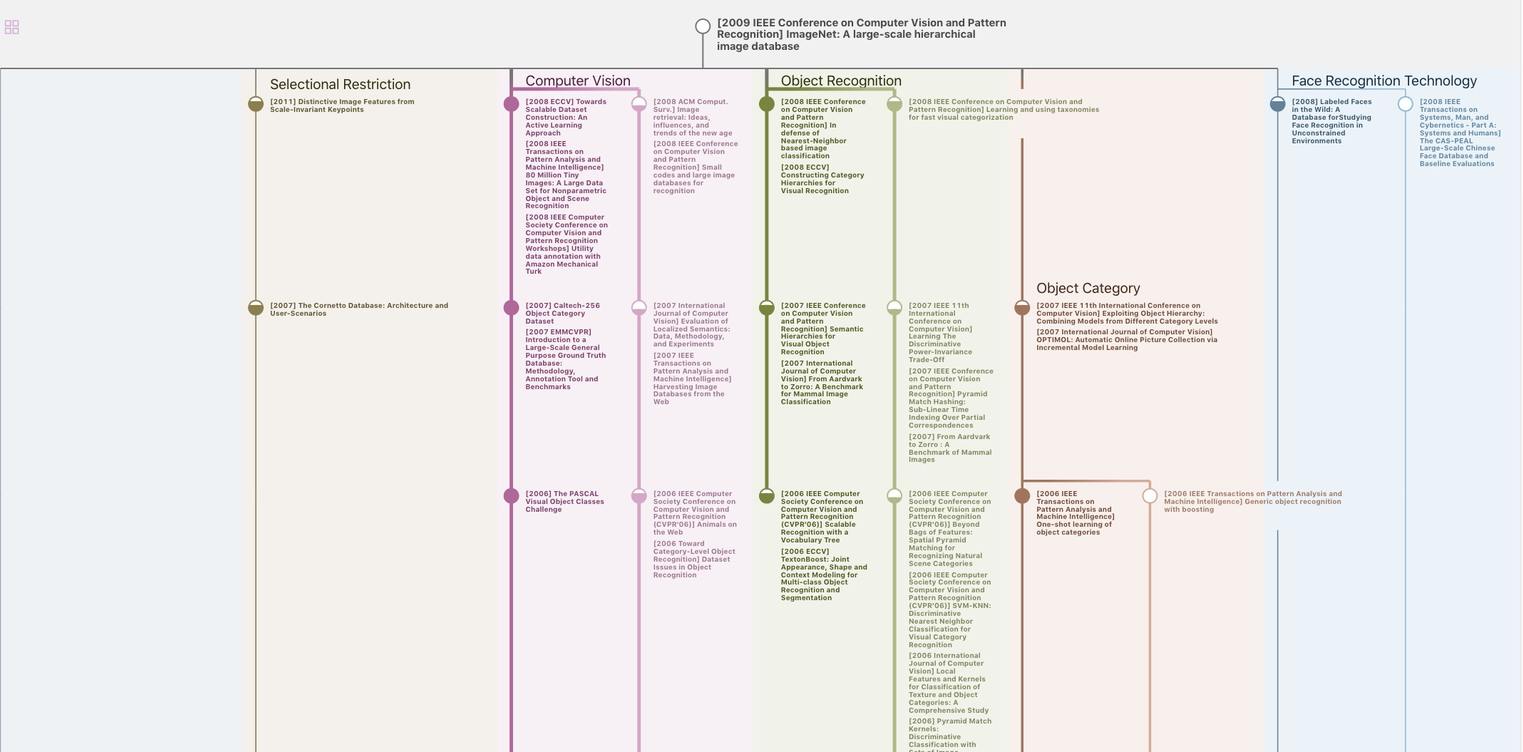
生成溯源树,研究论文发展脉络
Chat Paper
正在生成论文摘要