CaPUF: Cascaded PUF Structure for Machine Learning Resiliency
IEEE Transactions on Computer-Aided Design of Integrated Circuits and Systems(2022)
摘要
With the rise of the Internet of Things (IoT), resource-constrained and power-constrained devices attract more attention. The need for lightweight solutions as alternatives to resource-intensive applications became more urgent. Moreover, as the number of connected devices grew, authenticating them became more challenging. Traditionally, this would be performed by using hash functions and secure memory to store a key, which both come at a high cost. physical unclonable functions (PUFs) emerged as a suitable lightweight alternative to hash functions to authenticate the devices. Using the inherent minute differences between integrated circuits (ICs), they can generate IC-specific responses for input challenges coming from a so-called verifier. Through the years, machine learning (ML) has been used to attack PUFs by modeling them and accurately predicting their response to a given challenge. This stimulated research on ML-resilient PUFs. This resilience came with the significant area and challenge-to-response delay overheads. In this work, we introduce the novel cascaded PUF (CaPUF) and show that it is resilient against state-of-the-art ML-based attacks, i.e., logistic regression (LR) and support vector machines (SVMs). These attacks could not achieve accuracy better than 52% against our CaPUF, which is only as good as flipping a coin. Additionally, our CaPUF requires 89% less area compared to state-of-the-art ML-resilient PUFs.
更多查看译文
关键词
Hardware security,modeling attacks,physical unclonable function (PUF)
AI 理解论文
溯源树
样例
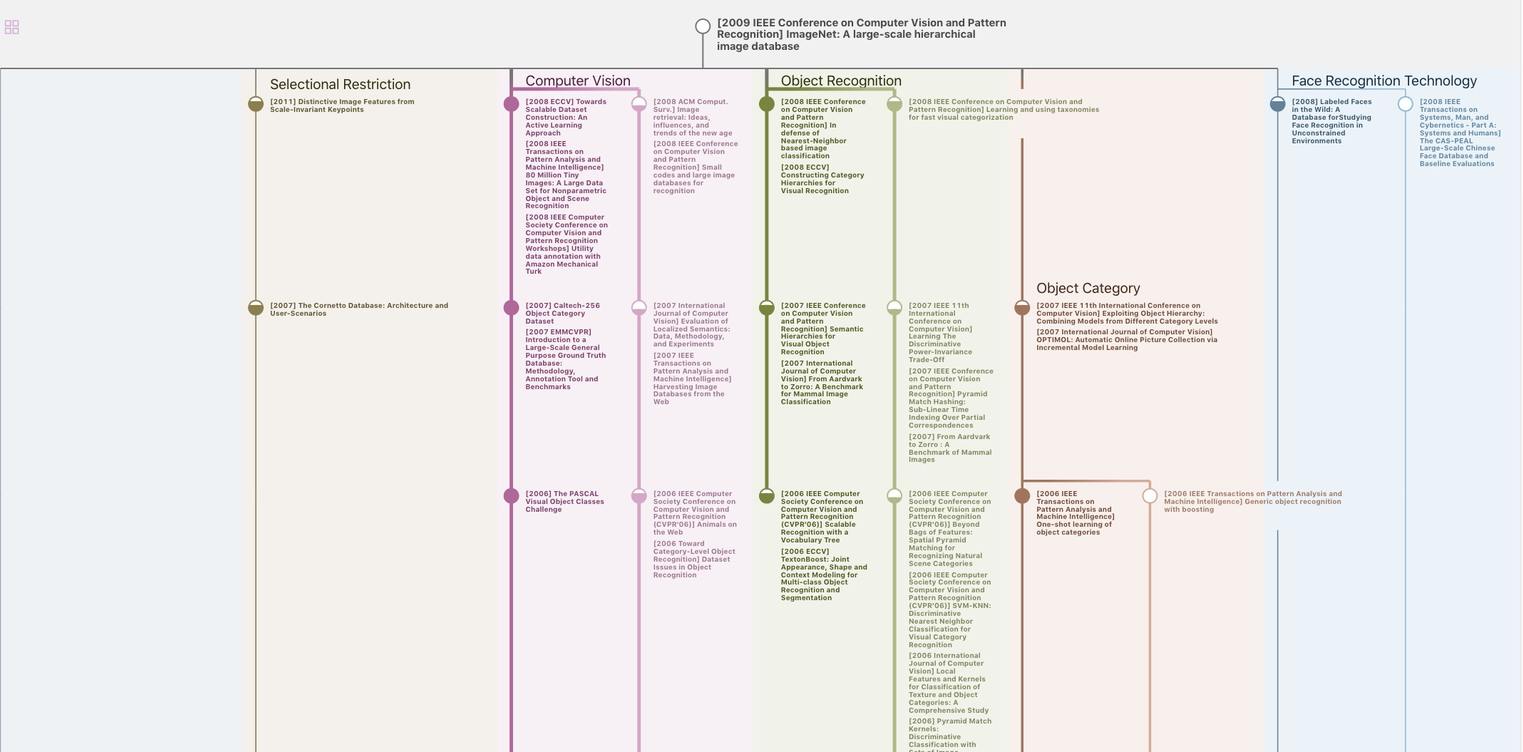
生成溯源树,研究论文发展脉络
Chat Paper
正在生成论文摘要