Group Residual Dense Block for Key-Point Detector with One-Level Feature.
Pacific Rim International Conference on Artificial Intelligence (PRICAI)(2022)
摘要
In this paper, we propose a novel key-point detector with only one-level feature with the stride of 8, which is 75.0% less than methods with the stride of 4. Due to the reduction of the feature layers, firstly we adopt a new key-point labeling method, which can make full use of the detection points on the feature map. Secondly, we propose a U-shaped feature fusion module with group residual dense blocks, which works together with grouped convolutional and re-parameterization methods to bring significant improvements while reducing parameters. Thirdly, we use a soft non-key-point branch to re-weight the classification score. Using NVIDIA GeForce 3060 GPU and based on the VOC dataset, the proposed model with RepVGG-A0 runs about 51.4% faster than CenterNet with ResNet-18, runs 261.3% faster, and achieves higher accuracy than CenterNet with ResNet-101 under the resolution of 512 x 512.
更多查看译文
关键词
Object detection,Key-point detector,One-level feature,Residual dense block,Re-parameterization
AI 理解论文
溯源树
样例
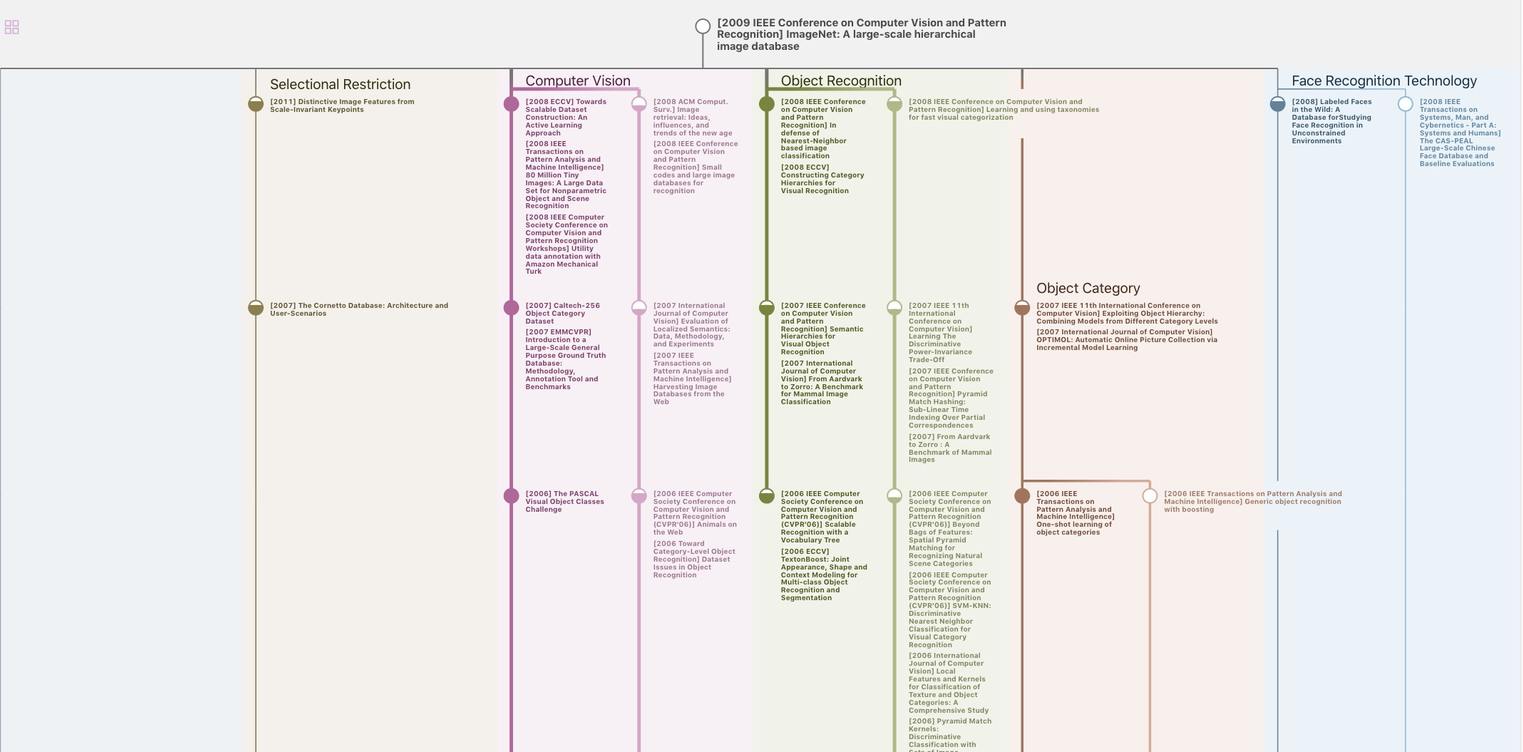
生成溯源树,研究论文发展脉络
Chat Paper
正在生成论文摘要