Efficient deep steering control method for self-driving cars through feature density metric
Neurocomputing(2023)
摘要
In this paper, a low cost method for input size reduction without sacrificing accuracy is proposed, which reduces required computation resources for both training and inference of deep convolutional neural network (DCNN) in the steering control of self-driving cars. Efficient processing of DCNNs is becoming prominent challenge due to its huge computation cost, number of parameters and also inadequate computation resources on power efficient hardware devices and the proposed method alleviates the problem comparing the state of the art. The proposed method introduces feature density metric (FDM) as criterion to mask and filter out regions of input image that do not contain adequate amount of features. This filtering method prevents DCNN from useless calculations belongs to feature-free regions. Compared to PilotNet, the proposed method accelerates overall training and inference phases of end-to-end (ETE) deep steering control of self-driving cars up to 1.3× and 2.0× respectively.
更多查看译文
关键词
Deep convolutional neural network,Computation reduction,Feature density metric,Self-driving car steering control
AI 理解论文
溯源树
样例
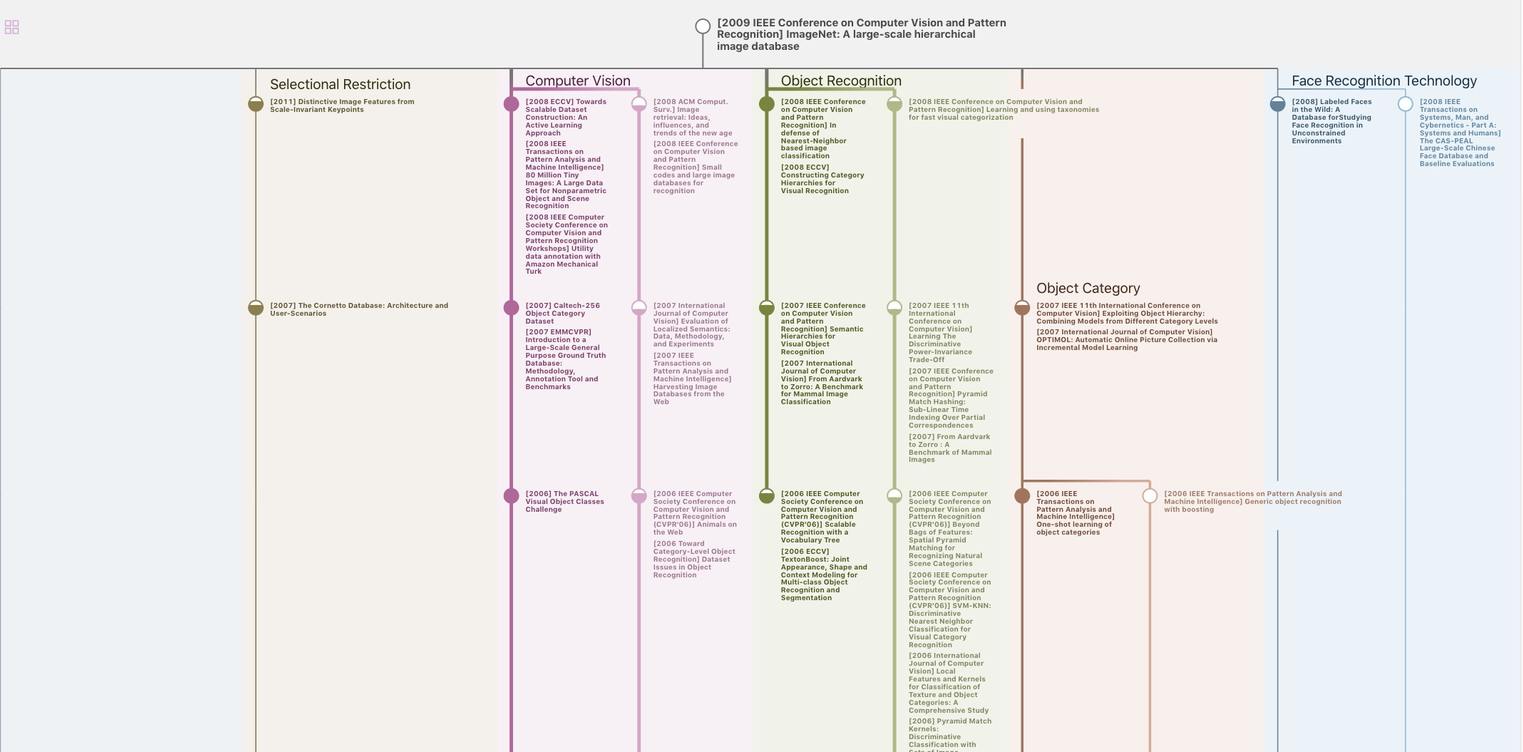
生成溯源树,研究论文发展脉络
Chat Paper
正在生成论文摘要