Improved Fine-Tuning of In-Domain Transformer Model for Inferring COVID-19 Presence in Multi-Institutional Radiology Reports
Journal of digital imaging(2022)
摘要
Building a document-level classifier for COVID-19 on radiology reports could help assist providers in their daily clinical routine, as well as create large numbers of labels for computer vision models. We have developed such a classifier by fine-tuning a BERT-like model initialized from RadBERT, its continuous pre-training on radiology reports that can be used on all radiology-related tasks. RadBERT outperforms all biomedical pre-trainings on this COVID-19 task ( P <0.01) and helps our fine-tuned model achieve an 88.9 macro-averaged F1-score, when evaluated on both X-ray and CT reports. To build this model, we rely on a multi-institutional dataset re-sampled and enriched with concurrent lung diseases, helping the model to resist to distribution shifts. In addition, we explore a variety of fine-tuning and hyperparameter optimization techniques that accelerate fine-tuning convergence, stabilize performance, and improve accuracy, especially when data or computational resources are limited. Finally, we provide a set of visualization tools and explainability methods to better understand the performance of the model, and support its practical use in the clinical setting. Our approach offers a ready-to-use COVID-19 classifier and can be applied similarly to other radiology report classification tasks.
更多查看译文
关键词
Radiology,COVID-19,Classification,Natural language processing (NLP),Transformer,BERT
AI 理解论文
溯源树
样例
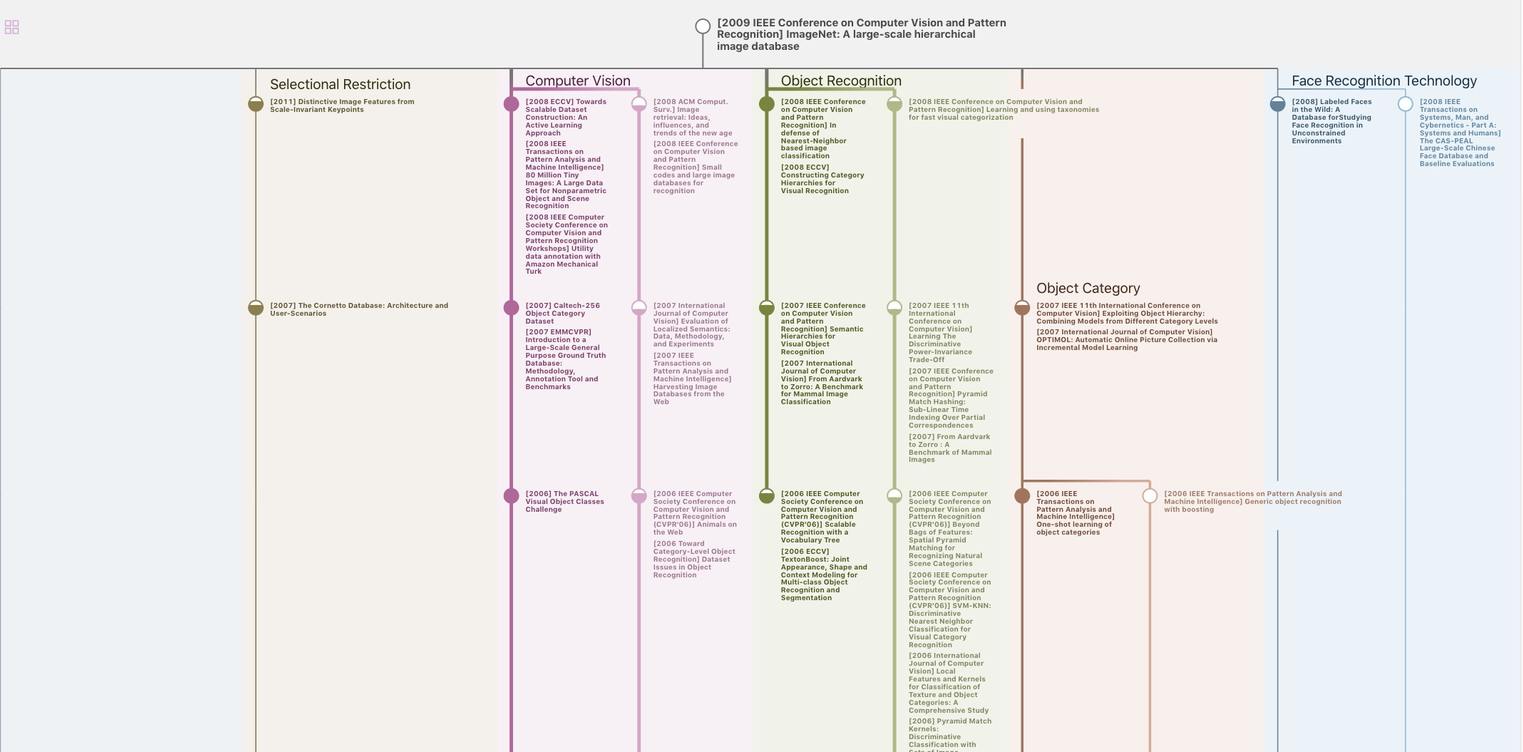
生成溯源树,研究论文发展脉络
Chat Paper
正在生成论文摘要