MorphoAST: A Machine Learning Workflow for Sub-Doubling Time Single Cell Antimicrobial Susceptibility Testing
biorxiv(2022)
摘要
Background: Multidrug-resistant bacteria are among the most urgent global public health threats. Rapid determination of antimicrobial resistance in a single bacterium is a major clinical unmet need in the diagnosis of bacterial infections. Methods: By capturing dynamic single-cell morphological features of over twenty-eight thousand cells, we evaluated strategies based on time and concentration differentials for classifying the susceptibility of Klebsiella pneumoniae to meropenem and predicting their minimum inhibitory concentrations (MIC). Findings: The classifiers achieved as high as 97% accuracy in 20 minutes (two-fifths of the doubling time) and reached over 99% accuracy within 50 minutes (one doubling time) in predicting the antimicrobial response. A regression model based on the concentration differential of individual cells predicted the MIC with >97% categorical agreement and 100% essential agreement. When tested against cells from an unseen strain, the regressor achieved a categorical agreement of 91.9% with a very major error of 0.1%. Interpretation: We report morphometric antimicrobial susceptibility testing (MorphoAST), an image-based machine learning workflow, for rapid determination of antimicrobial susceptibility by single-cell morphological analysis in a sub-doubling time. Our approach has the ability to predict bacterial antimicrobial responsiveness in a fraction of the organisms doubling time. This innovation will have significant implications for the future management of bacterial infections. Funding: This work was supported in part by NIH NIAID (R01AI153133).
### Competing Interest Statement
The authors have declared no competing interest.
更多查看译文
AI 理解论文
溯源树
样例
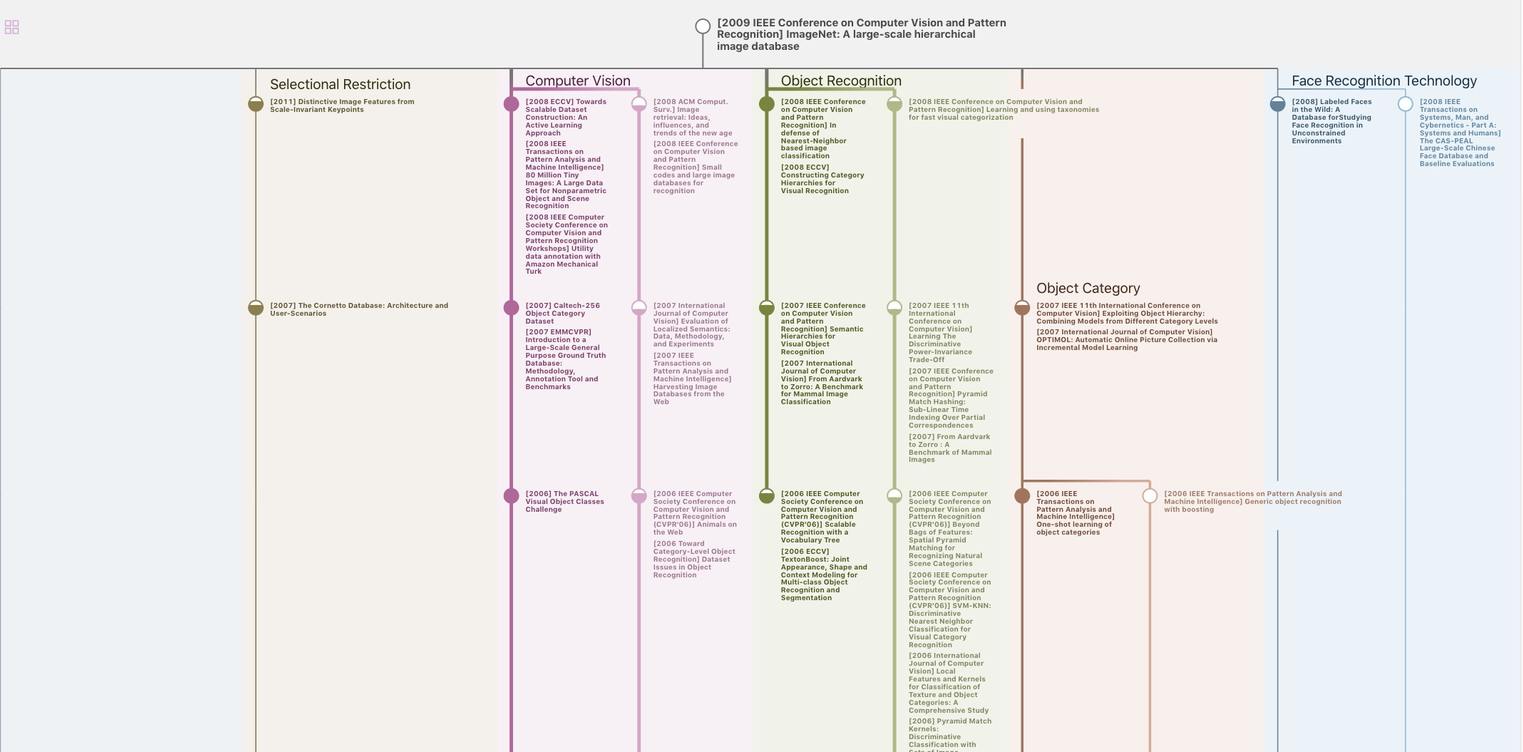
生成溯源树,研究论文发展脉络
Chat Paper
正在生成论文摘要