Impact of Own Brand Product Introduction on Optimal Pricing Models for Platform and Incumbent Sellers
Information Systems Research(2022)
Key words
own brand product,sell-on contract,sell-to contract,e-commerce platform,antitrust regulation
AI Read Science
Must-Reading Tree
Example
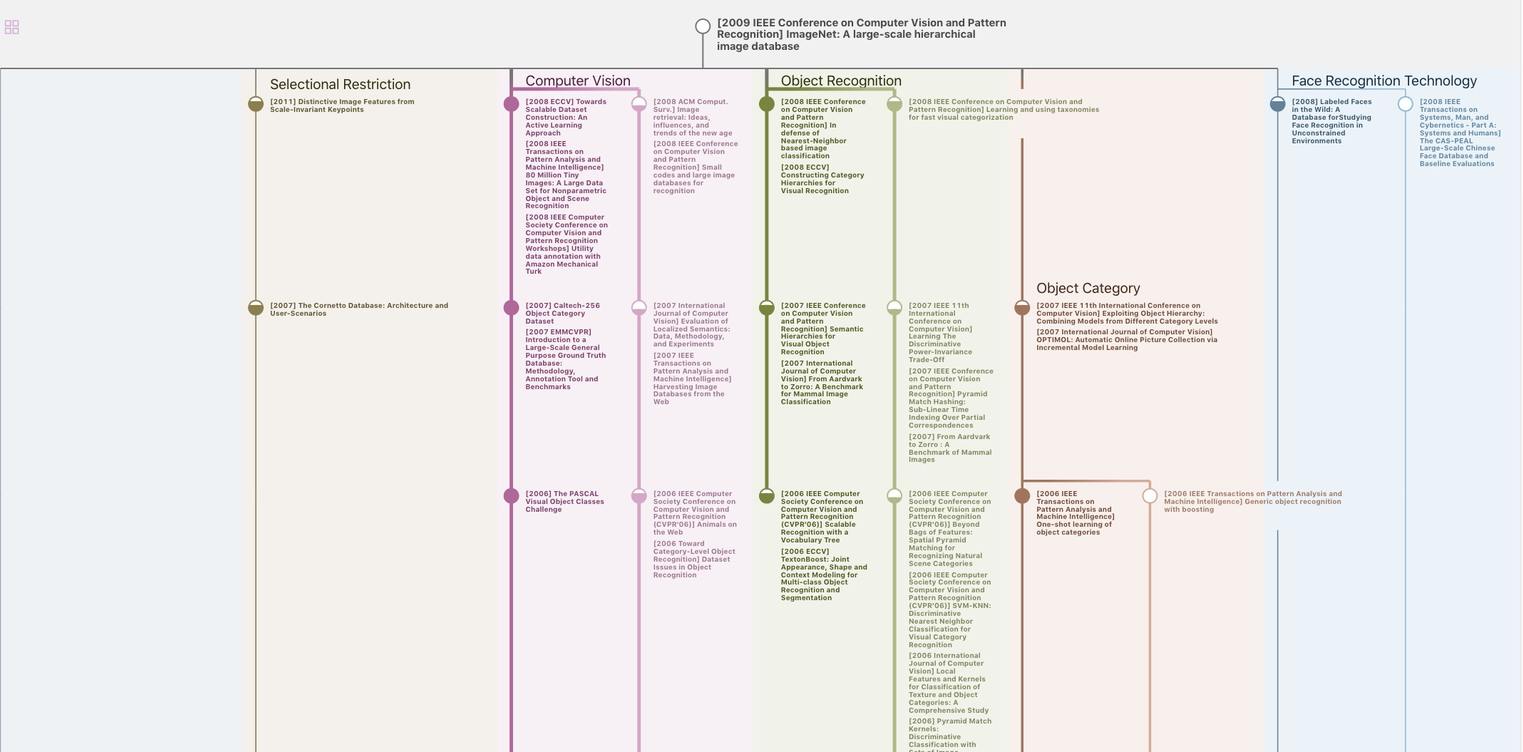
Generate MRT to find the research sequence of this paper
Chat Paper
Summary is being generated by the instructions you defined