Computer-aided detection and prognosis of colorectal cancer on whole slide images using dual resolution deep learning
Journal of cancer research and clinical oncology(2022)
摘要
Purpose Rapid diagnosis and risk stratification can provide timely treatment for colorectal cancer (CRC) patients. Deep learning (DL) is not only used to identify tumor regions in histopathological images, but also applied to predict survival and achieve risk stratification. Whereas, most of methods dependent on regions of interest annotated by pathologist and ignore the global information in the image. Methods A dual resolution DL network based on weakly supervised learning (WDRNet) was proposed for CRC identification and prognosis. The proposed method was trained and validated on the dataset from The Cancer Genome Atlas (TCGA) and tested on the external dataset from Affiliated Cancer Hospital and Institute of Guangzhou Medical University (ACHIGMU). Results In identification task, WDRNet accurately identified tumor images with an accuracy of 0.977 in slide level and 0.953 in patch level. Besides, in prognosis task, WDRNet showed an excellent prediction performance in both datasets with the concordance index (C-index) of 0.716 ± 0.037 and 0.598 ± 0.024 respectively. Moreover, the results of risk stratification were statistically significant in univariate analysis ( p < 0.001, HR = 7.892 in TCGA-CRC, and p = 0.009, HR = 1.718 in ACHIGMU) and multivariate analysis ( p < 0.001, HR = 5.914 in TCGA-CRC, and p = 0.025, HR = 1.674 in ACHIGMU). Conclusions We developed a weakly supervised resolution DL network to achieve precise identification and prognosis of CRC patients, which will assist doctors in diagnosis on histopathological images and stratify patients to select appropriate therapeutic schedule.
更多查看译文
关键词
Colorectal cancer,Deep learning,Identification,Prognosis,Whole-slide Images
AI 理解论文
溯源树
样例
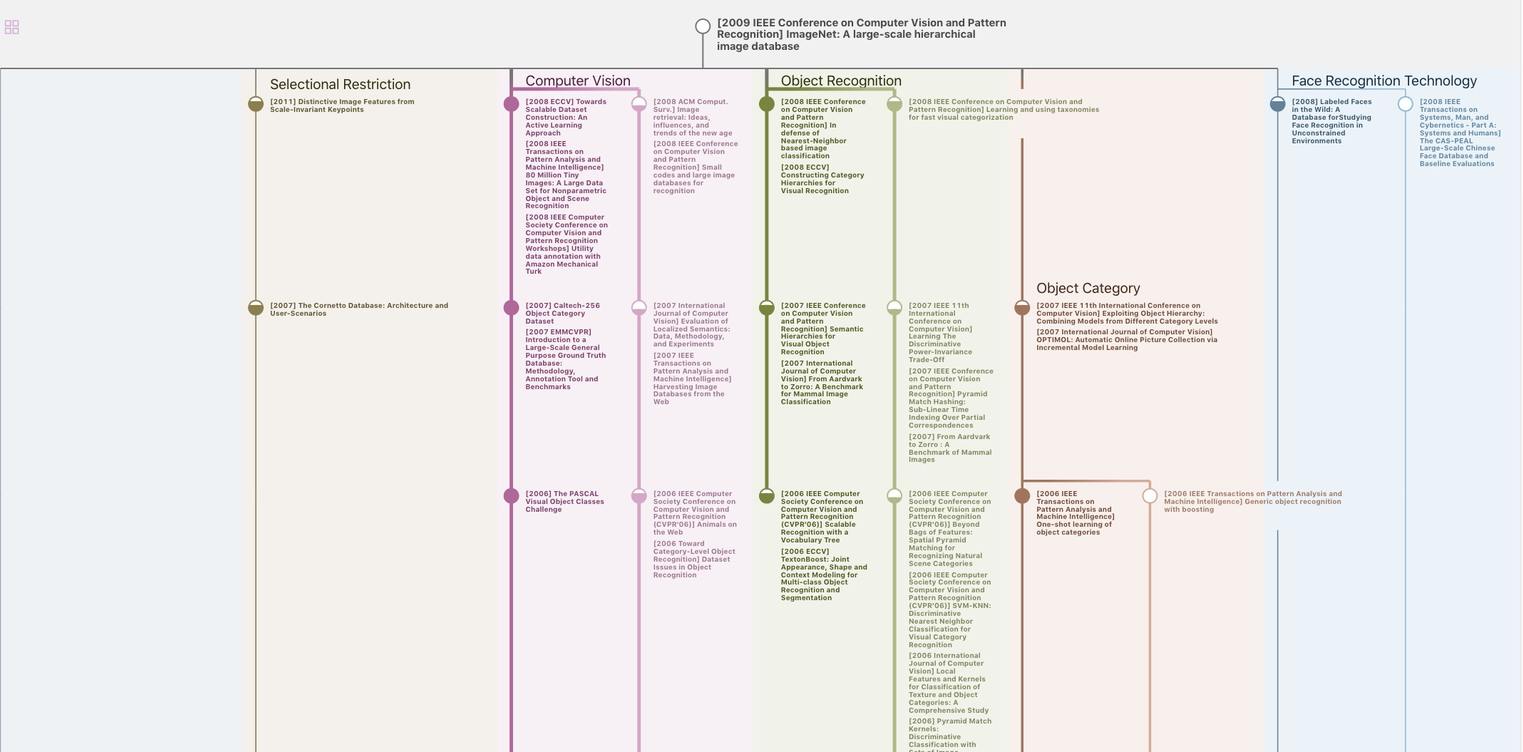
生成溯源树,研究论文发展脉络
Chat Paper
正在生成论文摘要