Novel ceramic plate defect detection using YOLO- R
2022 14th International Conference on Electronics, Computers and Artificial Intelligence (ECAI)(2022)
摘要
Deep learning based visual inspection solution have provided great results in the last decade warranting further investments in this area. The amount of data available is the sole bottleneck of these systems. Enough data and the model can confidently replace human workers. Not enough data and the project will implode. For stoneware and ceramics manufacturers, visual inspection is a critical task for the quality of the products. Being high volume, a fast inspection system is needed. Accuracy is also a critical threshold. It is mandatory to be at least as accurate as a human quality inspector. In this work we propose a fast, accurate and medium cost defect detection solution based on the YOLOR model. The version derived from it for the task of defect detection is trained on ceramic plate defects, achieves up to 0.354 validation mAP at an intersection of union(IoU) of 0.5 at 100 epochs. Judging by speed, the model comfortably processes a frame in 33ms on a consumer RTX3070 GPU. Furthermore, the critical aspect of the development process is building a large dataset of more than 100.000 samples and constructing a data engine that is capable of handling all this data. Other important tasks are the: model development process, single or multi-GPU training, deployment via a pilot line, testing and validation.
更多查看译文
关键词
convolutional neural networks,defect detection,automated inspection,artificial intelligence,industry
AI 理解论文
溯源树
样例
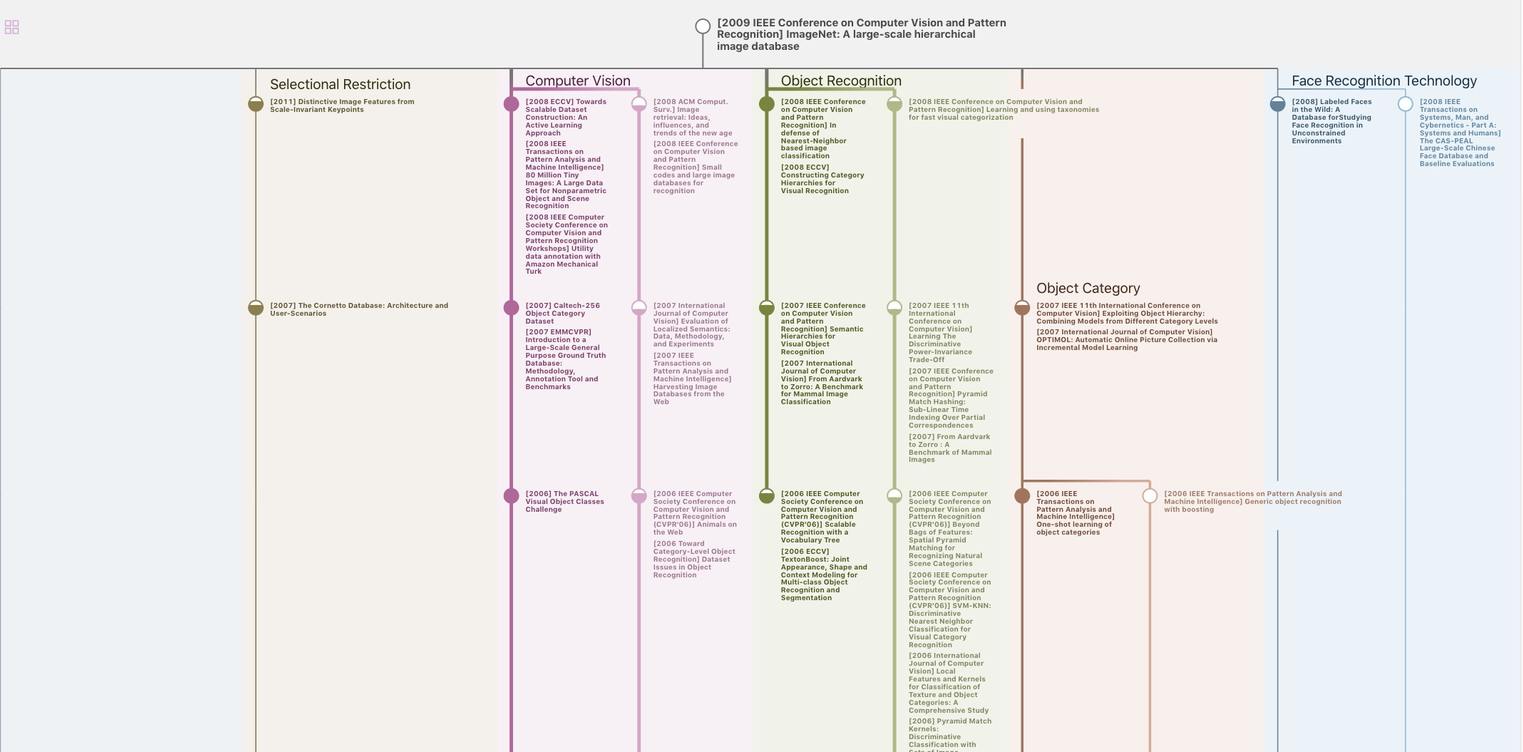
生成溯源树,研究论文发展脉络
Chat Paper
正在生成论文摘要