Segmentation Mask Resampling for MRI Prostate Localization Improvement
2022 International Conference on Electrical, Computer and Energy Technologies (ICECET)(2022)
摘要
This study aimed to examine the effect of segmentation mask resampling on segmentation performance and to confirm whether attempt to convert anisotropic data to isotropic can improve results of the current state of the art segmentation technique. A total of 50 prostate cancer cases gathered at 4 different institutions were examined. Magnetic resonance images of different resolutions and spacing were input into both 2D and 3D nnU-Net networks. Dice similarity coefficient was used as a metric to compare segmentation results between different models validated with the use of k-fold cross validation. Models trained included 2D and 3D models on original data and 3D models on resampled data. Image resampling was performed by applying third order spline interpolation. Two techniques were used for binary segmentation mask resampling: nearest neighbor interpolation followed by morphological opening and closing and calculation of average shape to be inserted between two adjacent slices. The mean dice similarity coefficients were 0.8736±0.0480 and 0.8680±0.0552 for 2D and 3D models with original data respectively. The use of both resampling techniques resulted in better segmentation results than no resampling with 0.8787±0.0299 and 0.8965±0.0165 for models with segmentation masks resampled with morphological operations and average shape calculation respectively. Thus, the study confirmed that converting anisotropic data to isotropic can improve segmentation results as well as reduce room for error.
更多查看译文
关键词
Segmentation mask resampling,isotropic data,prostate localization,nnUNet model,MRI
AI 理解论文
溯源树
样例
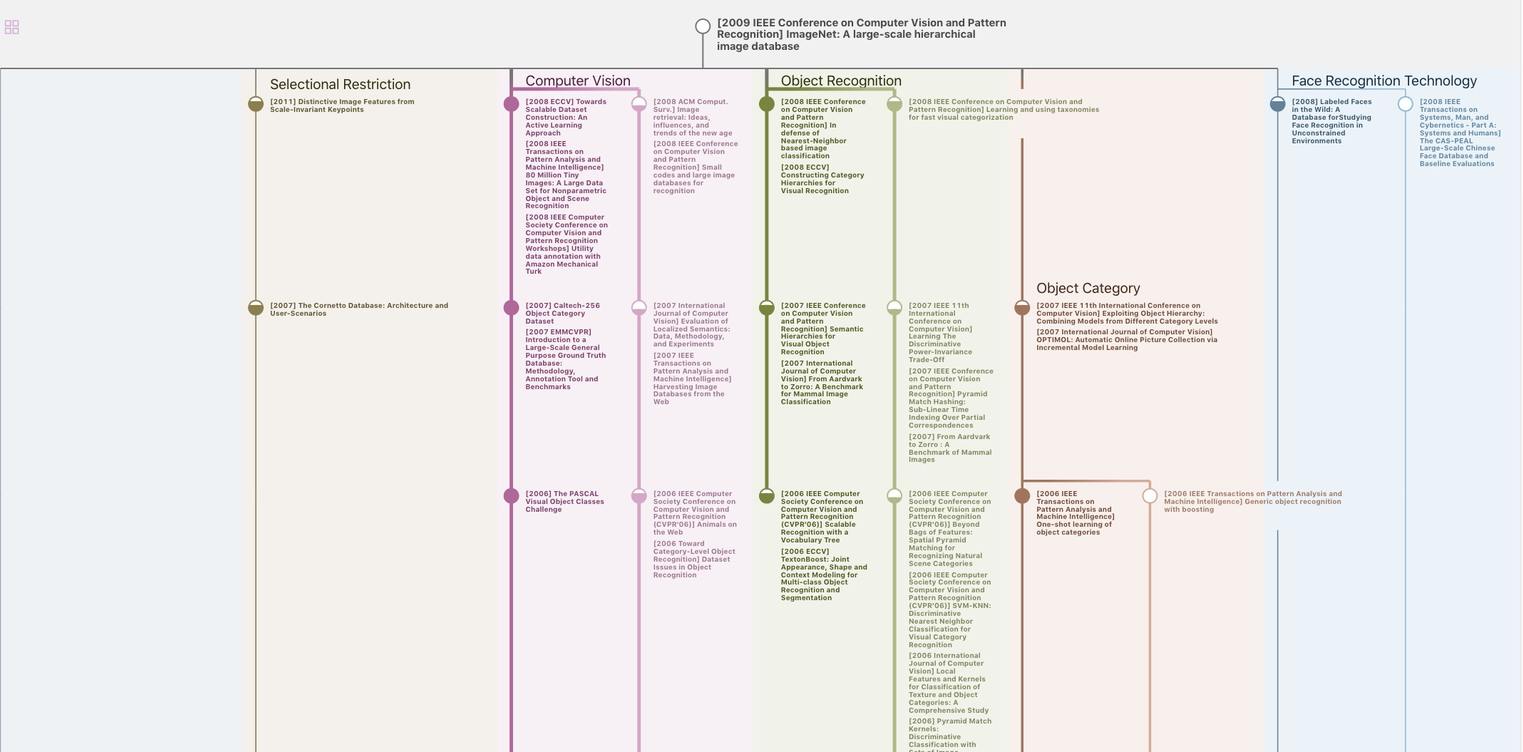
生成溯源树,研究论文发展脉络
Chat Paper
正在生成论文摘要