Condition Management of Low-Voltage Distribution Cables
2022 IEEE Electrical Insulation Conference (EIC)(2022)
Budapest Univ Technol & Econ
Abstract
Distribution grid operators face various challenges today. In general, many of the assets in the current grid are approaching the end of their design lifetime and it is feared that they will soon be unable to perform their tasks safely and reliably. Furthermore, there are major risks associated with the increasing penetration of electric vehicles and residential solar generation. This phenomenon is changing the load patterns seen so far, presumably causing the accelerated aging of cables. At the same time, as the cables are subjected to different mechanical, thermal and electrical stresses during their operation, the condition of each device may differ. Low-voltage cables are in most cases weakly documented and have been operated in the run-to-failure strategy due to the lack of interest and sufficient diagnostic methods. Though in the future, the available methods may be broader, in the current situation, the condition management needs to be solved based on scattered and inaccurate information. This paper describes the development of a condition management strategy to support maintaining a more reliable low-voltage power grid and to identify the most important degrading factors that require investigation.
MoreTranslated text
Key words
cables,asset management,aging,distribution,lack of data
求助PDF
上传PDF
View via Publisher
AI Read Science
AI Summary
AI Summary is the key point extracted automatically understanding the full text of the paper, including the background, methods, results, conclusions, icons and other key content, so that you can get the outline of the paper at a glance.
Example
Background
Key content
Introduction
Methods
Results
Related work
Fund
Key content
- Pretraining has recently greatly promoted the development of natural language processing (NLP)
- We show that M6 outperforms the baselines in multimodal downstream tasks, and the large M6 with 10 parameters can reach a better performance
- We propose a method called M6 that is able to process information of multiple modalities and perform both single-modal and cross-modal understanding and generation
- The model is scaled to large model with 10 billion parameters with sophisticated deployment, and the 10 -parameter M6-large is the largest pretrained model in Chinese
- Experimental results show that our proposed M6 outperforms the baseline in a number of downstream tasks concerning both single modality and multiple modalities We will continue the pretraining of extremely large models by increasing data to explore the limit of its performance
Upload PDF to Generate Summary
Must-Reading Tree
Example
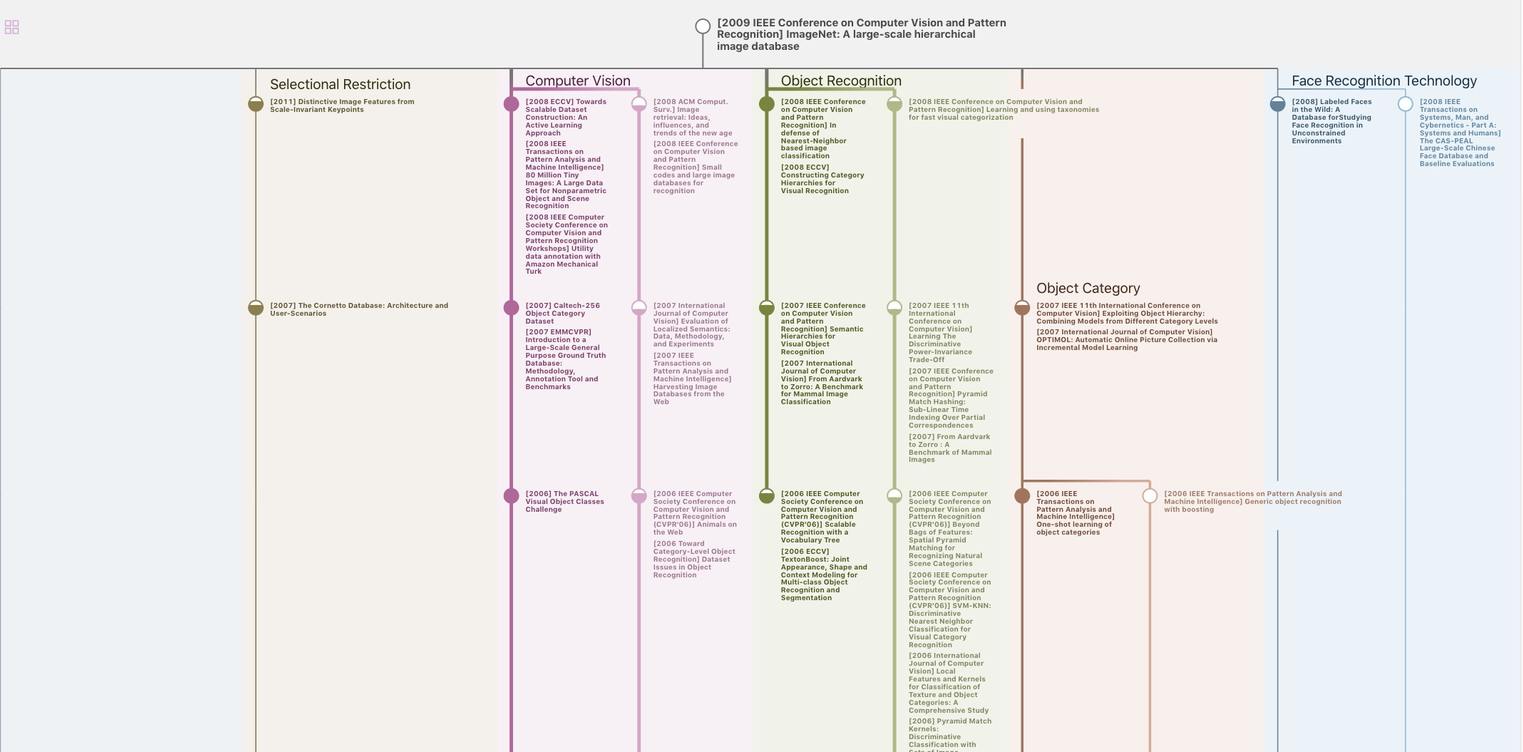
Generate MRT to find the research sequence of this paper
Related Papers
2008
被引用60 | 浏览
Data Disclaimer
The page data are from open Internet sources, cooperative publishers and automatic analysis results through AI technology. We do not make any commitments and guarantees for the validity, accuracy, correctness, reliability, completeness and timeliness of the page data. If you have any questions, please contact us by email: report@aminer.cn
Chat Paper
GPU is busy, summary generation fails
Rerequest