A Comparative Study of Misclassification Cost Assignment Strategies for Cost-sensitive AdaBoost in Imbalance Data Classification
2022 19th International Conference on Electrical Engineering/Electronics, Computer, Telecommunications and Information Technology (ECTI-CON)(2022)
摘要
Classification of data where the number of examples in each class differ significantly is not uncommon. Imbalance data can be found in various application domains including biomedical data analysis. The traditional supervised learning following the Empirical Risk Minimisation principle, which minimises the misclassification regardless of the types of error, often yields a classification model that generalises well only on the majority class but tends to perform poorly on the minority class. Interestingly, correct classification of the minority class is usually of greater importance. Cost-sensitive learning is one of the promising approaches to introducing inductive bias into the model for imbalance data classification. Nonetheless, there is currently a limited body of research on how to properly set the misclassification costs in imbalance settings. To elucidate that, in this paper, we studied three strategies for determining misclassification costs for an imbalance dataset and incorporated such costs into a cost-sensitive AdaBoost algorithm. The experimental results based on five imbalance biomedical testbeds suggested that the proposed distribution correction method is the most effective strategy in terms of imbalance-aware performance measures.
更多查看译文
关键词
cost-sensitive learning,imbalance data classification,cost-sensitive AdaBoost algorithm,misclassification cost assignment strategies,supervised learning,empirical risk minimisation principle,classification model
AI 理解论文
溯源树
样例
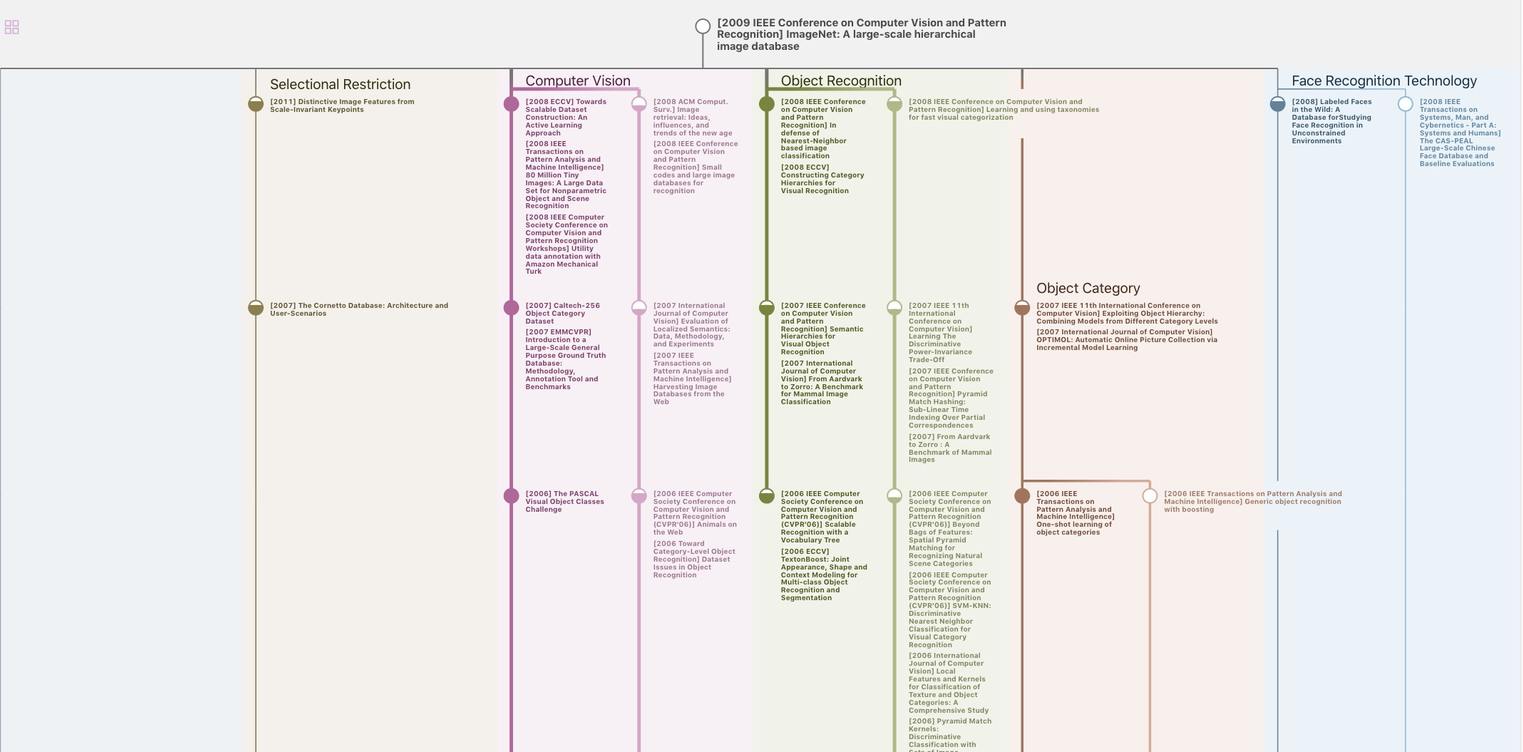
生成溯源树,研究论文发展脉络
Chat Paper
正在生成论文摘要