Geometry and convergence of natural policy gradient methods
Information Geometry(2023)
摘要
We study the convergence of several natural policy gradient (NPG) methods in infinite-horizon discounted Markov decision processes with regular policy parametrizations. For a variety of NPGs and reward functions we show that the trajectories in state-action space are solutions of gradient flows with respect to Hessian geometries, based on which we obtain global convergence guarantees and convergence rates. In particular, we show linear convergence for unregularized and regularized NPG flows with the metrics proposed by Kakade and Morimura and co-authors by observing that these arise from the Hessian geometries of conditional entropy and entropy respectively. Further, we obtain sublinear convergence rates for Hessian geometries arising from other convex functions like log-barriers. Finally, we interpret the discrete-time NPG methods with regularized rewards as inexact Newton methods if the NPG is defined with respect to the Hessian geometry of the regularizer. This yields local quadratic convergence rates of these methods for step size equal to the inverse penalization strength.
更多查看译文
关键词
natural policy gradient methods,convergence
AI 理解论文
溯源树
样例
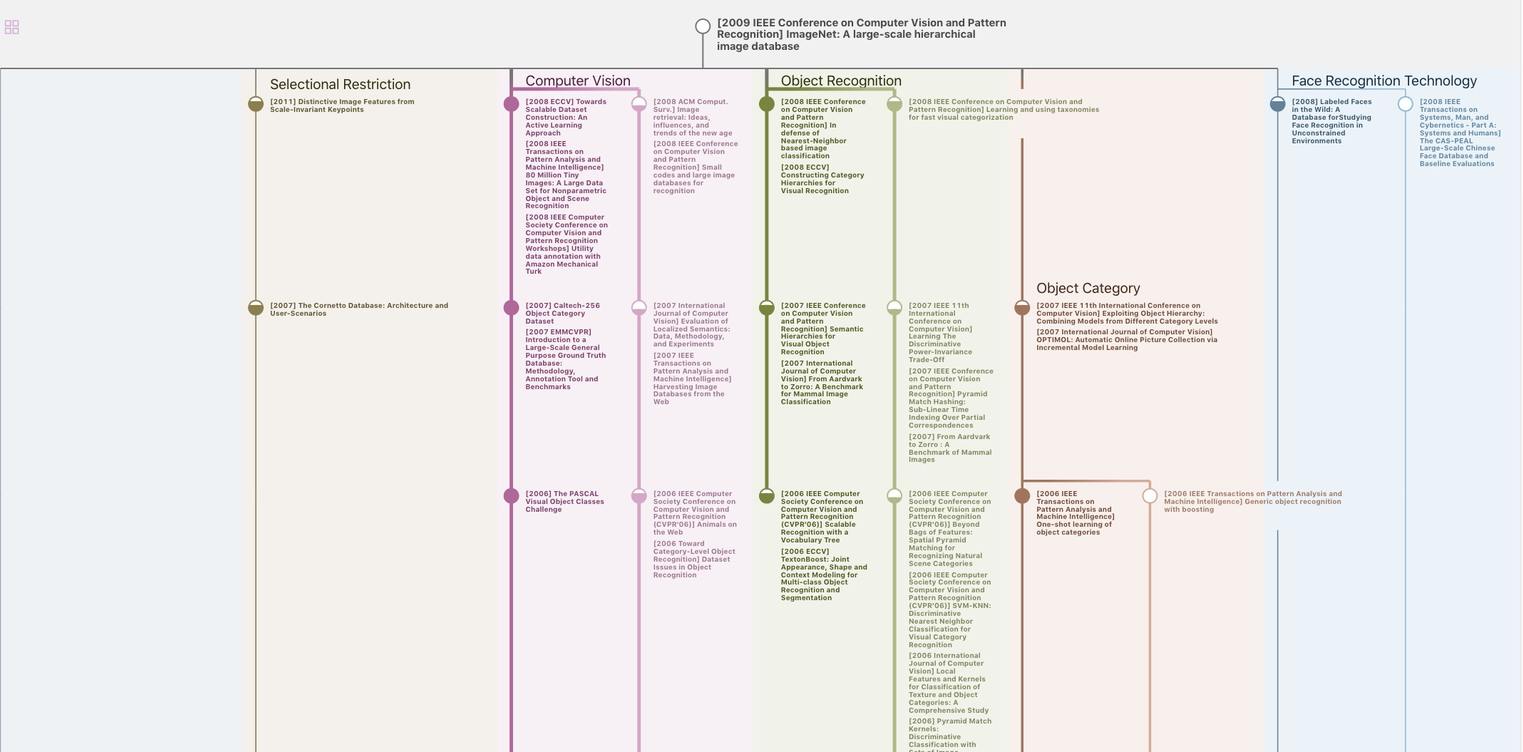
生成溯源树,研究论文发展脉络
Chat Paper
正在生成论文摘要