Improving Semi-supervised Deep Learning by Using Automatic Thresholding to Deal with out of Distribution Data for COVID-19 Detection Using Chest X-ray Images
openalex
摘要
Semi-supervised learning (SSL) leverages both labeled and unlabeled data for training models when the labeled data is limited and the unlabeled data is vast. Frequently, the unlabeled data is more widely available than the labeled data, hence this data is used to improve the level of generalization of a model when the labeled data is scarce. However, in real-world settings unlabeled data might depict a different distribution than the labeled dataset distribution. This is known as distribution mismatch. Such problem generally occurs when the source of unlabeled data is different from the labeled data. For instance, in the medical imaging domain, when training a COVID-19 detector using chest X-ray images, different unlabeled datasets sampled from different hospitals might be used. In this work, we propose an automatic thresholding method to filter out-of-distribution data in the unlabeled dataset. We use the Mahalanobis distance between the labeled and unlabeled datasets using the feature space built by a pre-trained Image-net Feature Extractor (FE) to score each unlabeled observation. We test two simple automatic thresholding methods in the context of training a COVID-19 detector using chest X-ray images. The tested methods provide an automatic manner to define what unlabeled data to preserve when training a semi-supervised deep learning architecture.
更多查看译文
关键词
Semi-Supervised Learning,Unsupervised Learning,Transfer Learning,Meta-Learning,Deep Learning
AI 理解论文
溯源树
样例
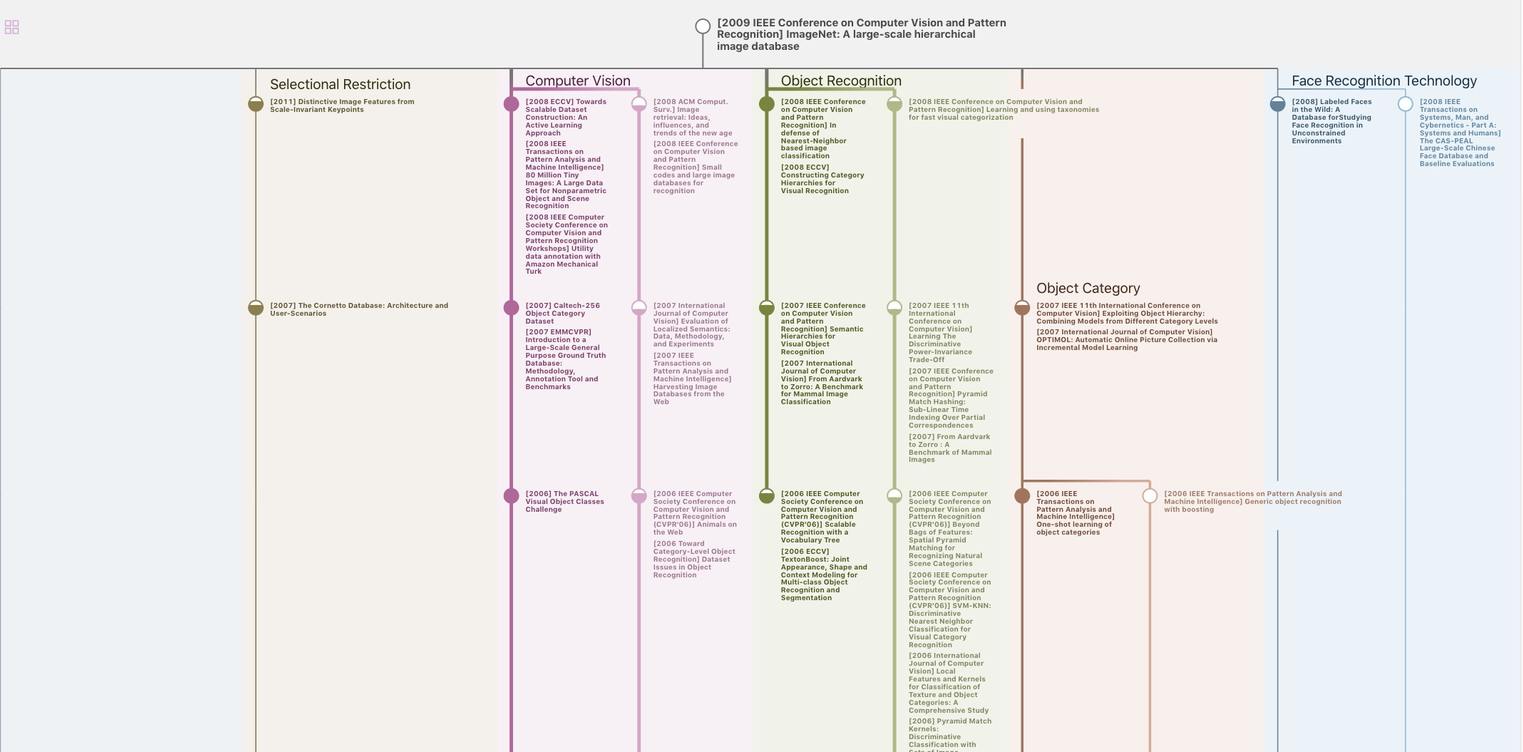
生成溯源树,研究论文发展脉络
Chat Paper
正在生成论文摘要