Concentration inequalities for leave-one-out cross validation
CoRR(2023)
摘要
In this article we prove that estimator stability is enough to show that leave-one-out cross validation is a sound procedure, by providing concentration bounds in a general framework. In particular, we provide concentration bounds beyond Lipschitz continuity assumptions on the loss or on the estimator. In order to obtain our results, we rely on random variables with distribution satisfying the logarithmic Sobolev inequality, providing us a relatively rich class of distributions. We illustrate our method by considering several interesting examples, including linear regression, kernel density estimation, and stabilized / truncated estimators such as stabilized kernel regression.
更多查看译文
关键词
validation,cross,leave-one-out
AI 理解论文
溯源树
样例
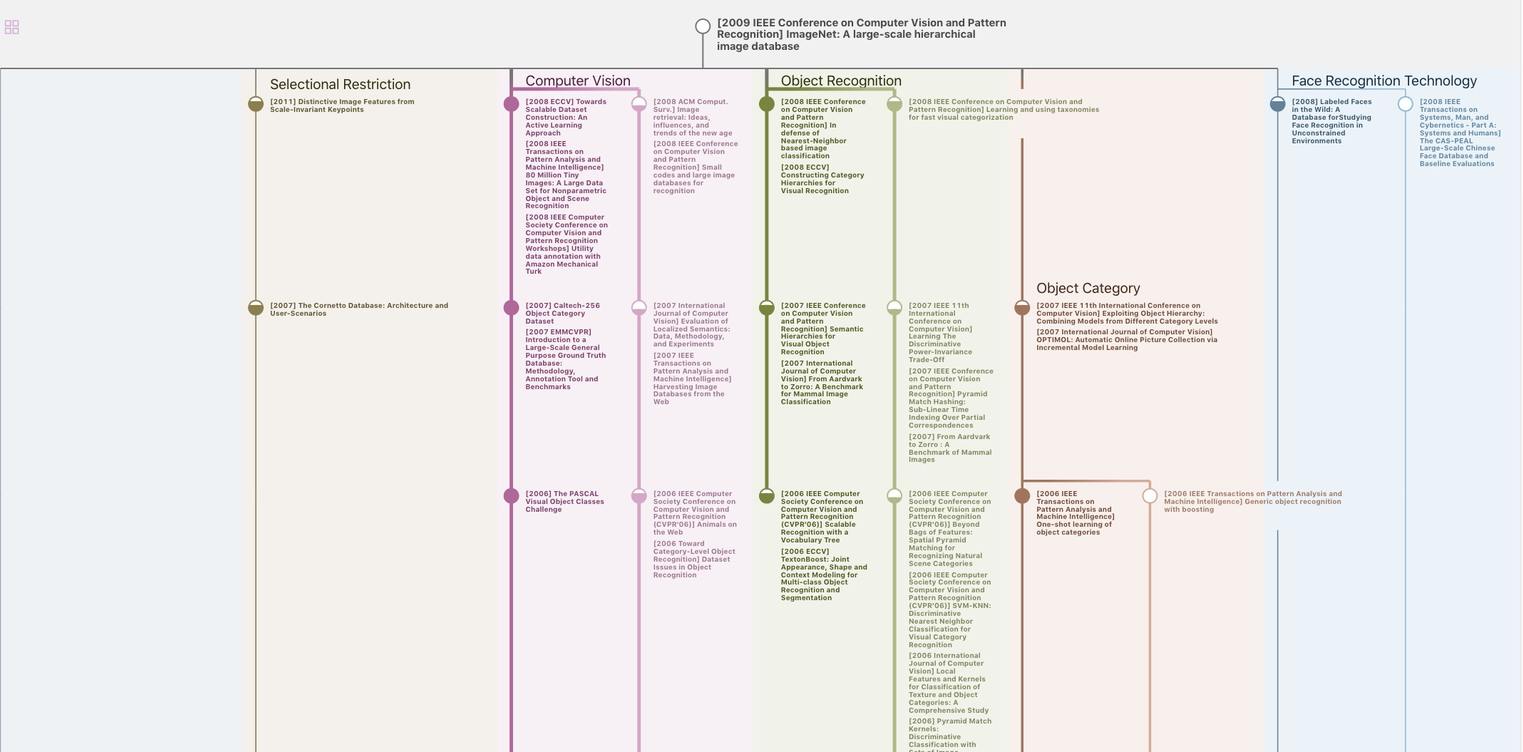
生成溯源树,研究论文发展脉络
Chat Paper
正在生成论文摘要