Deep reinforcement learning for flow control exploits different physics for increasing Reynolds-number regimes
arxiv(2022)
摘要
Deep artificial neural networks (ANNs) used together with deep reinforcement learning (DRL) are receiving growing attention due to their capabilities to control complex problems. This technique has been recently used to solve problems related to flow control. In this work, an ANN trained through a DRL agent is used to perform active flow control. Two-dimensional simulations of the flow around a cylinder are conducted and an active control based on two jets located on the walls of the cylinder is considered. By gathering information from the flow surrounding the cylinder, the ANN agent is able to learn effective control strategies for the jets, leading to a significant drag reduction. In the present work, a Reynolds-number range beyond those previously considered is studied and compared with results obtained using classical flow-control methods. Significantly different nature in the control strategies is identified by the DRL as the Reynolds number Re increases. For Re <= 1000 the classical control strategy based on an opposition control relative to the wake oscillation is obtained. For Re = 2000 the new strategy consists of an energisation of the boundary layers and the separation area, which modulate the flow separation and reduce drag in a fashion similar to that of the drag crisis, through a high frequency actuation. A cross-application of agents is performed for a flow at Re = 2000, obtaining similar results in terms of drag reduction with the agents trained at Re = 1000 and 2000. The fact that two different strategies yield the same performance make us question whether this Reynolds number regime (Re = 2000) belongs to a transition towards a nature-different flow which would only admit a high-frequency actuation strategy to obtain drag reduction. This finding allows the application of ANNs trained at lower Reynolds numbers but comparable in nature, saving computational resources.
更多查看译文
关键词
numerical simulation,wake dynamics,flow control,machine learning,deep reinforcement learning
AI 理解论文
溯源树
样例
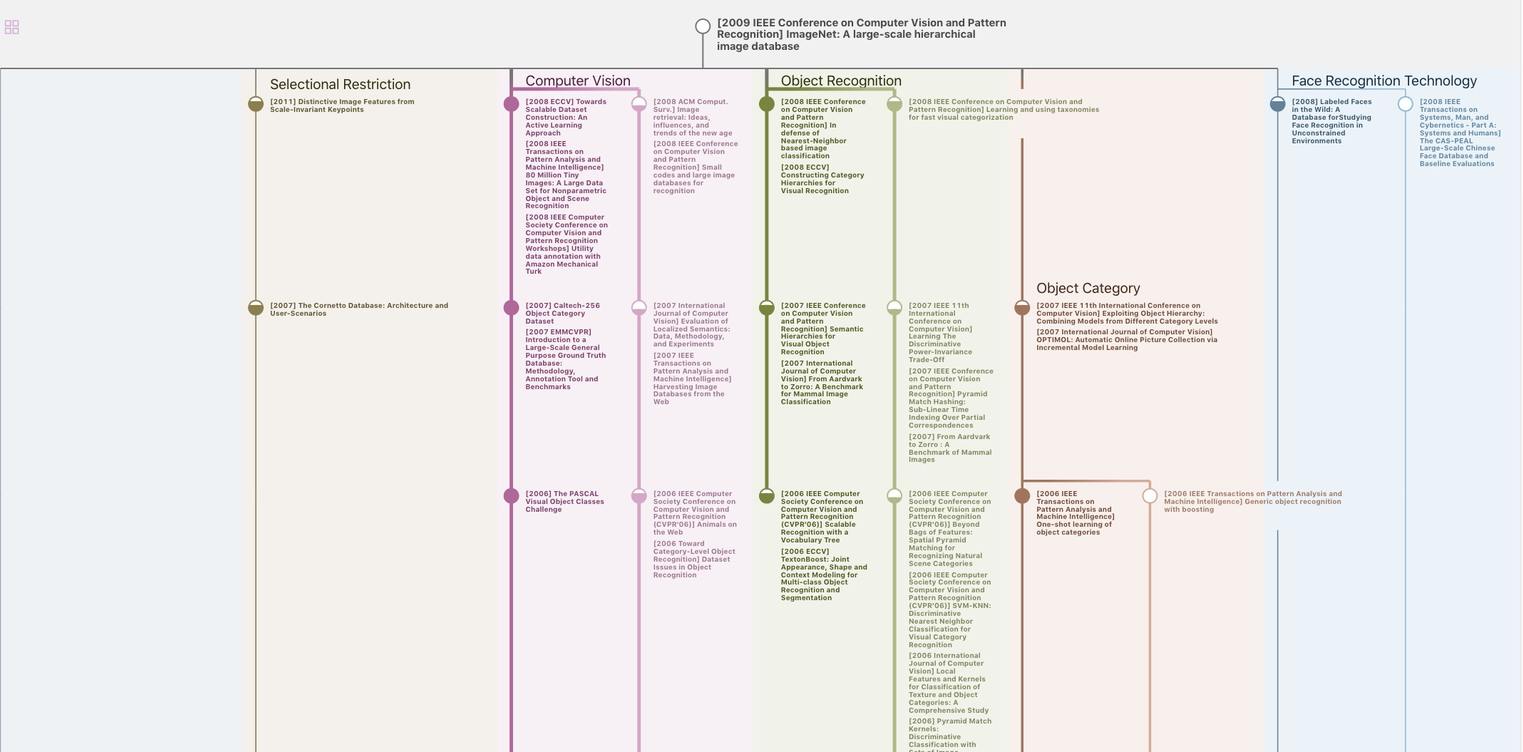
生成溯源树,研究论文发展脉络
Chat Paper
正在生成论文摘要