Predicting Treatment Adherence of Tuberculosis Patients at Scale
arxiv(2022)
摘要
Tuberculosis (TB), an infectious bacterial disease, is a significant cause of death, especially in low-income countries, with an estimated ten million new cases reported globally in $2020$. While TB is treatable, non-adherence to the medication regimen is a significant cause of morbidity and mortality. Thus, proactively identifying patients at risk of dropping off their medication regimen enables corrective measures to mitigate adverse outcomes. Using a proxy measure of extreme non-adherence and a dataset of nearly $700,000$ patients from four states in India, we formulate and solve the machine learning (ML) problem of early prediction of non-adherence based on a custom rank-based metric. We train ML models and evaluate against baselines, achieving a $\sim 100\%$ lift over rule-based baselines and $\sim 214\%$ over a random classifier, taking into account country-wide large-scale future deployment. We deal with various issues in the process, including data quality, high-cardinality categorical data, low target prevalence, distribution shift, variation across cohorts, algorithmic fairness, and the need for robustness and explainability. Our findings indicate that risk stratification of non-adherent patients is a viable, deployable-at-scale ML solution. As the official AI partner of India's Central TB Division, we are working on multiple city and state-level pilots with the goal of pan-India deployment.
更多查看译文
关键词
tuberculosis patients,treatment adherence
AI 理解论文
溯源树
样例
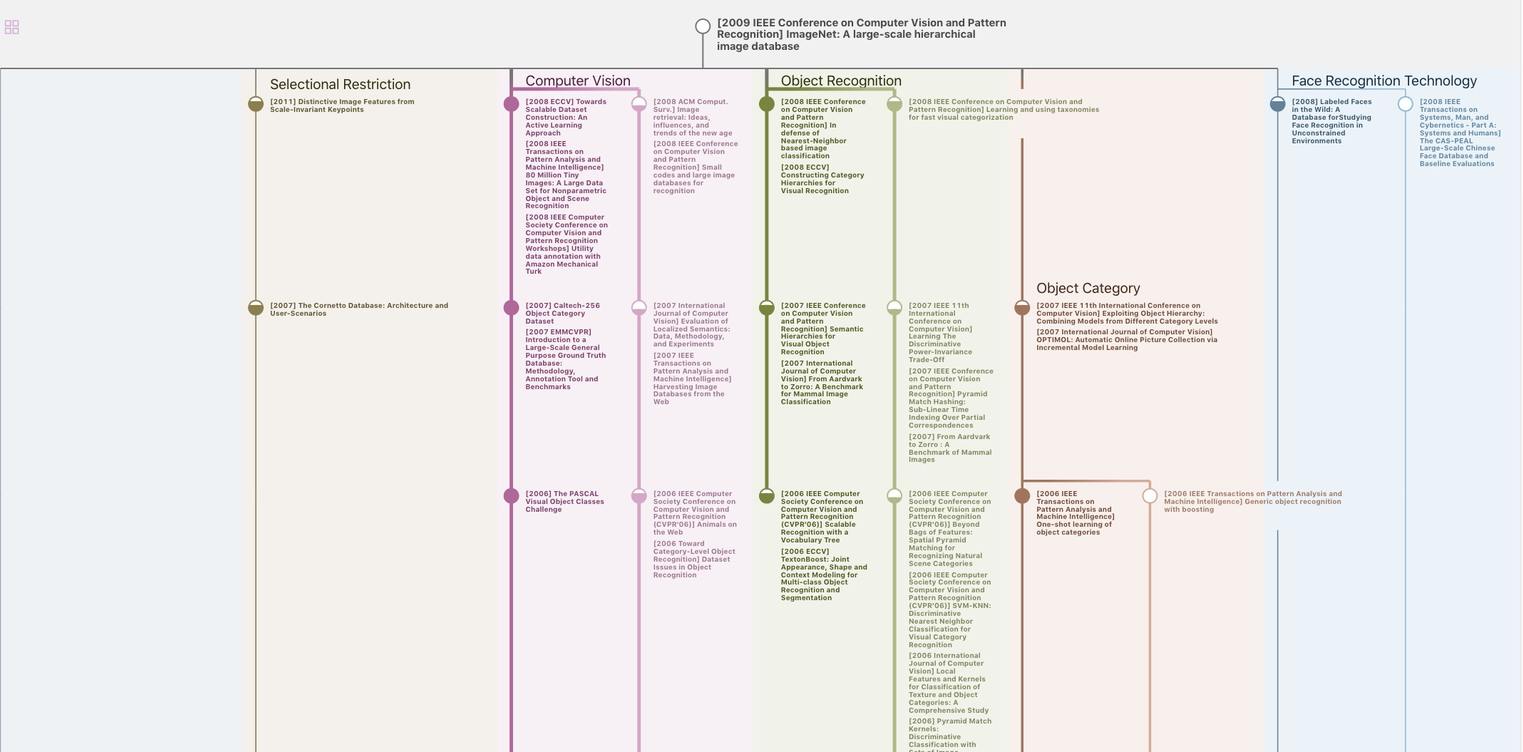
生成溯源树,研究论文发展脉络
Chat Paper
正在生成论文摘要