Online Nash Welfare Maximization Without Predictions
arxiv(2023)
摘要
The maximization of Nash welfare, which equals the geometric mean of agents' utilities, is widely studied because it balances efficiency and fairness in resource allocation problems. Banerjee, Gkatzelis, Gorokh, and Jin (2022) recently introduced the model of online Nash welfare maximization for $T$ divisible items and $N$ agents with additive utilities with predictions of each agent's utility for receiving all items. They gave online algorithms whose competitive ratios are logarithmic. We initiate the study of online Nash welfare maximization \emph{without predictions}, assuming either that the agents' utilities for receiving all items differ by a bounded ratio, or that their utilities for the Nash welfare maximizing allocation differ by a bounded ratio. We design online algorithms whose competitive ratios only depend on the logarithms of the aforementioned ratios of agents' utilities and the number of agents.
更多查看译文
AI 理解论文
溯源树
样例
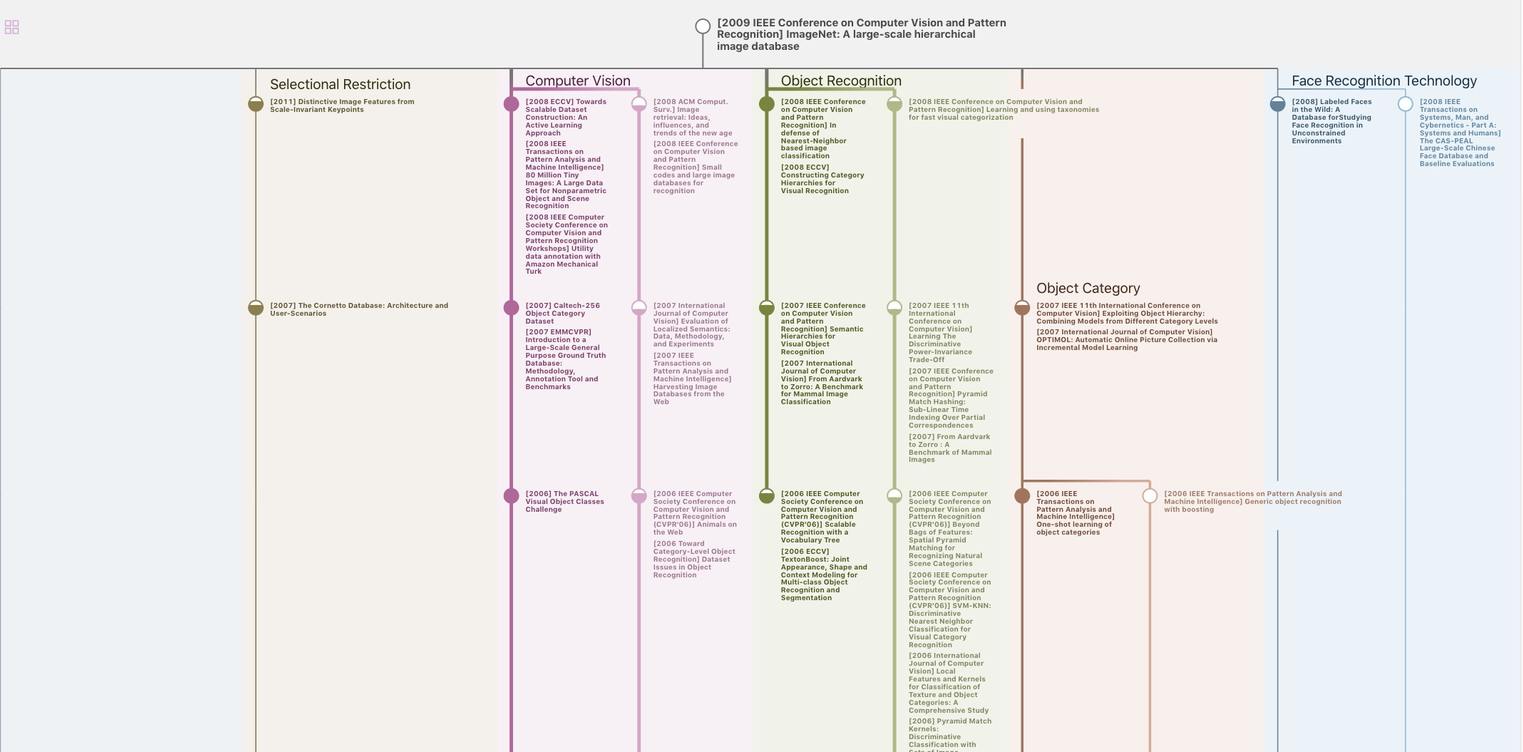
生成溯源树,研究论文发展脉络
Chat Paper
正在生成论文摘要