Stardust: Compiling Sparse Tensor Algebra to a Reconfigurable Dataflow Architecture
CoRR(2022)
Abstract
We introduce Stardust, a compiler that compiles sparse tensor algebra to reconfigurable dataflow architectures (RDAs). Stardust introduces new user-provided data representation and scheduling language constructs for mapping to resource-constrained accelerated architectures. Stardust uses the information provided by these constructs to determine on-chip memory placement and to lower to the Capstan RDA through a parallel-patterns rewrite system that targets the Spatial programming model. The Stardust compiler is implemented as a new compilation path inside the TACO open-source system. Using cycle-accurate simulation, we demonstrate that Stardust can generate more Capstan tensor operations than its authors had implemented and that it results in 138$\times$ better performance than generated CPU kernels and 41$\times$ better performance than generated GPU kernels.
MoreTranslated text
Key words
sparse tensor algebra,reconfigurable dataflow
AI Read Science
Must-Reading Tree
Example
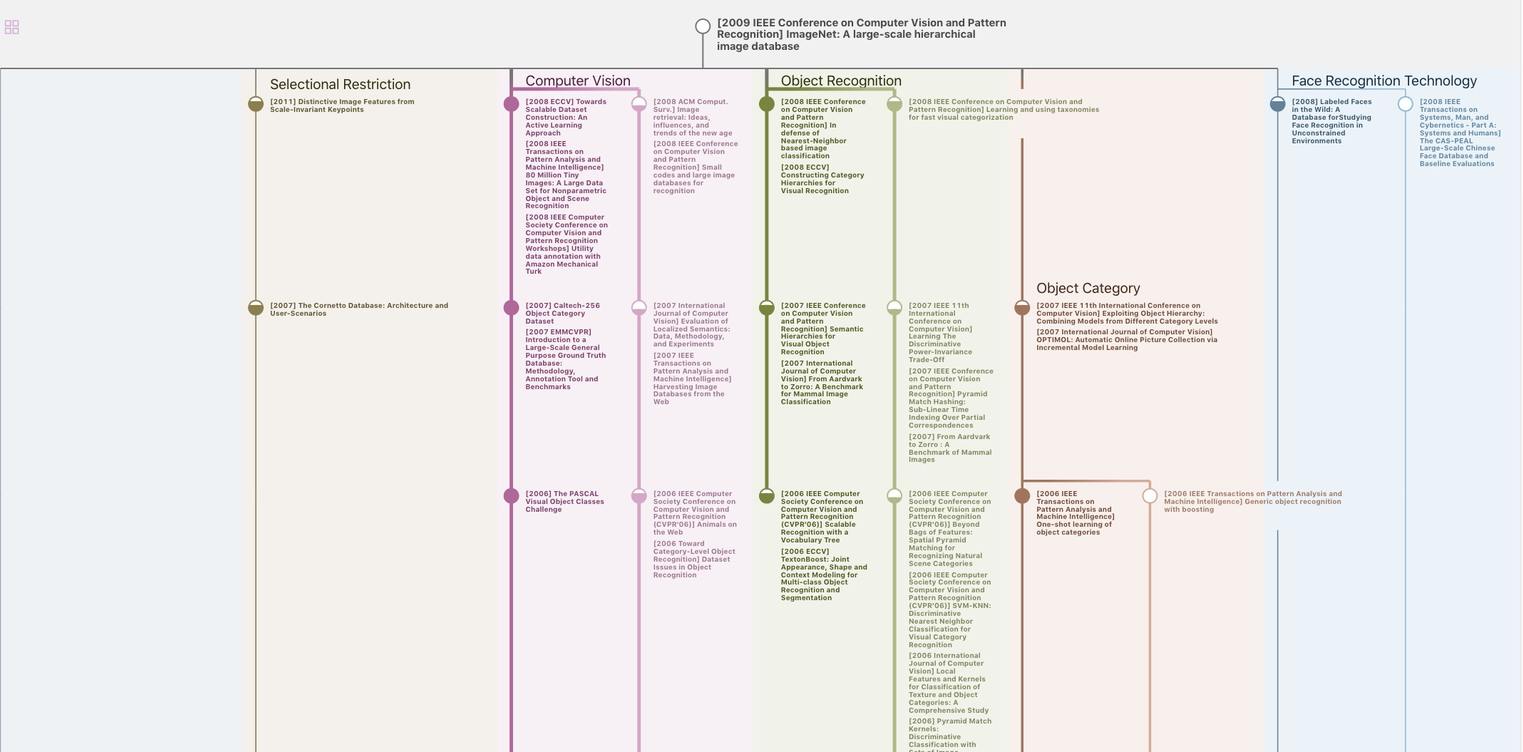
Generate MRT to find the research sequence of this paper
Chat Paper
Summary is being generated by the instructions you defined