Few-shot Image Generation with Diffusion Models
arxiv(2023)
摘要
Denoising diffusion probabilistic models (DDPMs) have been proven capable of synthesizing high-quality images with remarkable diversity when trained on large amounts of data. However, to our knowledge, few-shot image generation tasks have yet to be studied with DDPM-based approaches. Modern approaches are mainly built on Generative Adversarial Networks (GANs) and adapt models pre-trained on large source domains to target domains using a few available samples. In this paper, we make the first attempt to study when do DDPMs overfit and suffer severe diversity degradation as training data become scarce. Then we fine-tune DDPMs pre-trained on large source domains to solve the overfitting problem when training data is limited. Although the directly fine-tuned models accelerate convergence and improve generation quality and diversity compared with training from scratch, they still fail to retain some diverse features and can only produce coarse images. Therefore, we design a DDPM pairwise adaptation (DDPM-PA) approach to optimize few-shot DDPM domain adaptation. DDPM-PA efficiently preserves information learned from source domains by keeping the relative pairwise distances between generated samples during adaptation. Besides, DDPM-PA enhances the learning of high-frequency details from source models and limited training data. DDPM-PA further improves generation quality and diversity and achieves results better than current state-of-the-art GAN-based approaches. We demonstrate the effectiveness of our approach on a series of few-shot image generation tasks qualitatively and quantitatively.
更多查看译文
AI 理解论文
溯源树
样例
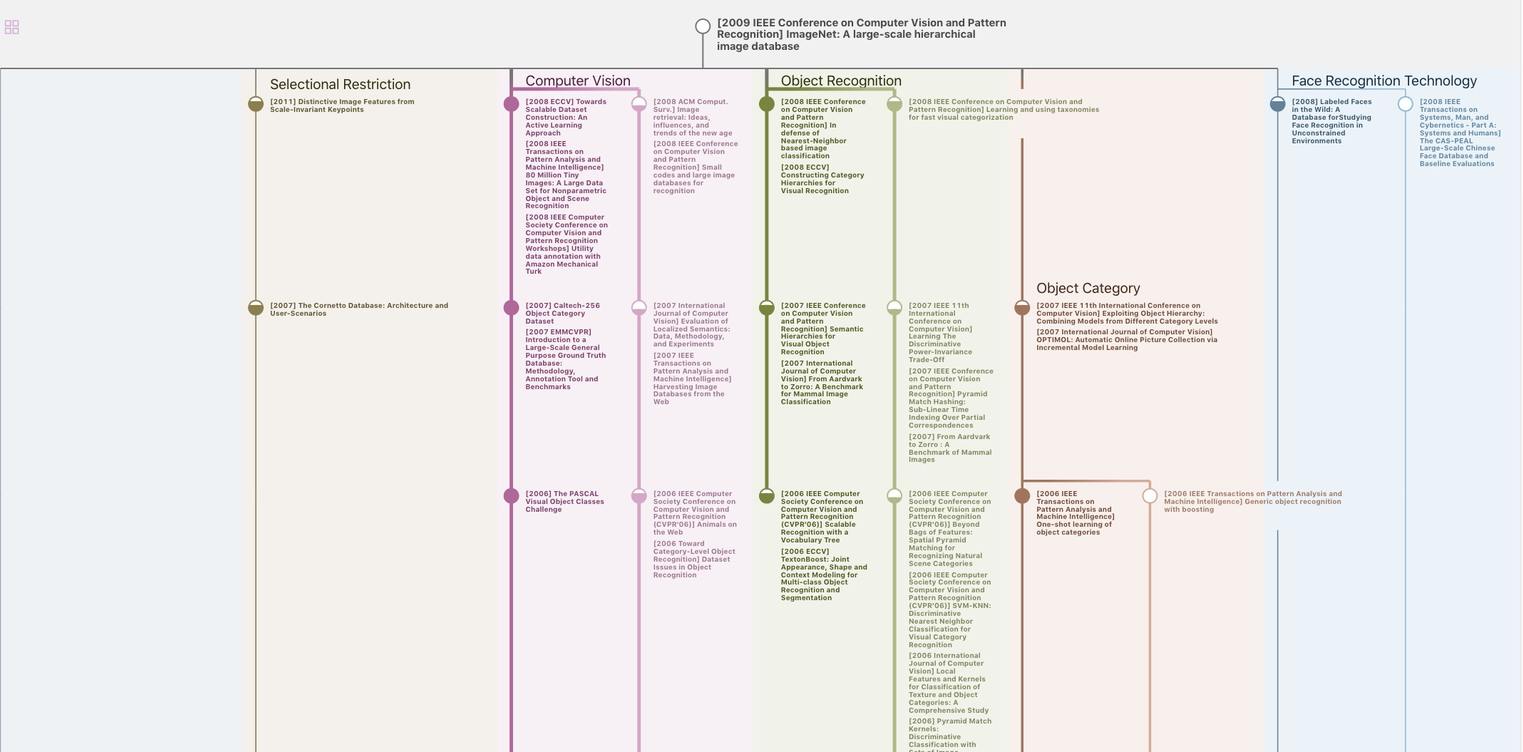
生成溯源树,研究论文发展脉络
Chat Paper
正在生成论文摘要