Machine learning based uncertainty quantification of turbulence model for airfoils
arxiv(2022)
摘要
Reynolds-averaged Navier-Stokes (RANS)-based transition modeling is widely used in aerospace applications but suffers inaccuracies due to the Boussinesq turbulent viscosity hypothesis. The eigenspace perturbation method can estimate the accuracy of a RANS model by injecting perturbations to its predicted Reynolds stresses. However, there lacks a reliable method for choosing the strength of the injected perturbation, while existing machine learning models are often complex and data craving. We examined two light-weighted machine learning models to help select the strength of the injected perturbation for estimating the RANS uncertainty of flows undergoing the transition to turbulence over a Selig-Donovan 7003 airfoil. On the one hand, we examined polynomial regression to construct a marker function augmented with eigenvalue perturbations to estimate the uncertainty bound for the predicted skin friction coefficient. On the other hand, we trained a convolutional neural network (CNN) to predict high-fidelity turbulence kinetic energy. The trained CNN acts as a marker function that can be integrated into the eigenspace perturbation method to quantify the RANS uncertainty. Our findings suggest that the light-weighted machine learning models are effective in constructing an appropriate marker function that is promising to enrich the existing eigenspace perturbation method to quantify the RANS uncertainty more precisely.
更多查看译文
关键词
turbulence model
AI 理解论文
溯源树
样例
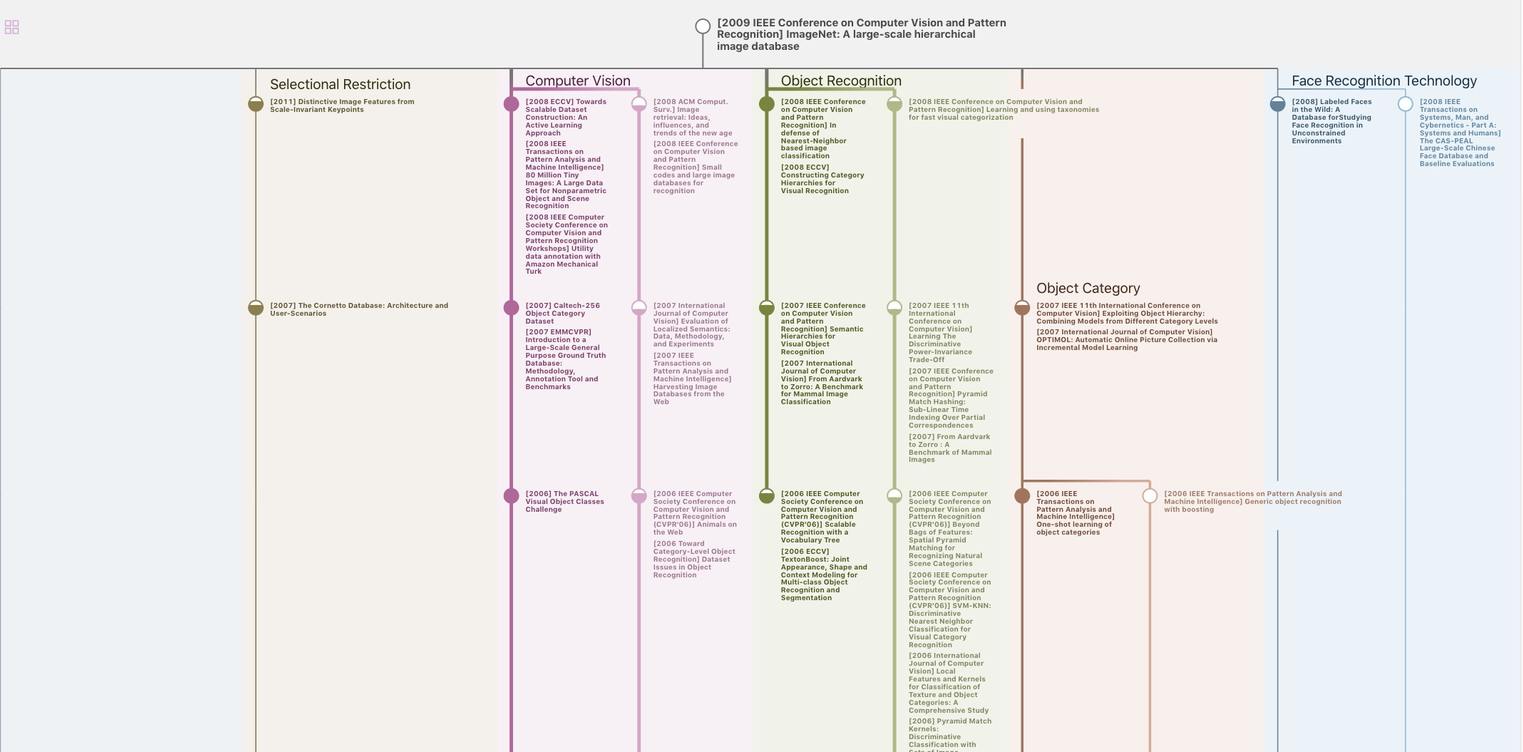
生成溯源树,研究论文发展脉络
Chat Paper
正在生成论文摘要