MILCDock: Machine Learning Enhanced Consensus Docking for Virtual Screening in Drug Discovery
JOURNAL OF CHEMICAL INFORMATION AND MODELING(2022)
摘要
Molecular docking tools are regularly used to computationally identify new molecules in virtual screening for drug discovery. However, docking tools suffer from inaccurate scoring functions with widely varying performance on different proteins. To enable more accurate ranking of active over inactive ligands in virtual screening, we created a machine learning consensus docking tool, MILCDock, that uses predictions from five traditional molecular docking tools to predict the probability a ligand binds to a protein. MILCDock was trained and tested on data from both the DUD-E and LIT-PCBA docking datasets and shows improved performance over traditional molecular docking tools and other consensus docking methods on the DUD-E dataset. LIT-PCBA targets proved to be difficult for all methods tested. We also find that DUD-E data, although biased, can be effective in training machine learning tools if care is taken to avoid DUD-E's biases during training.
更多查看译文
关键词
virtual screening,discovery,drug
AI 理解论文
溯源树
样例
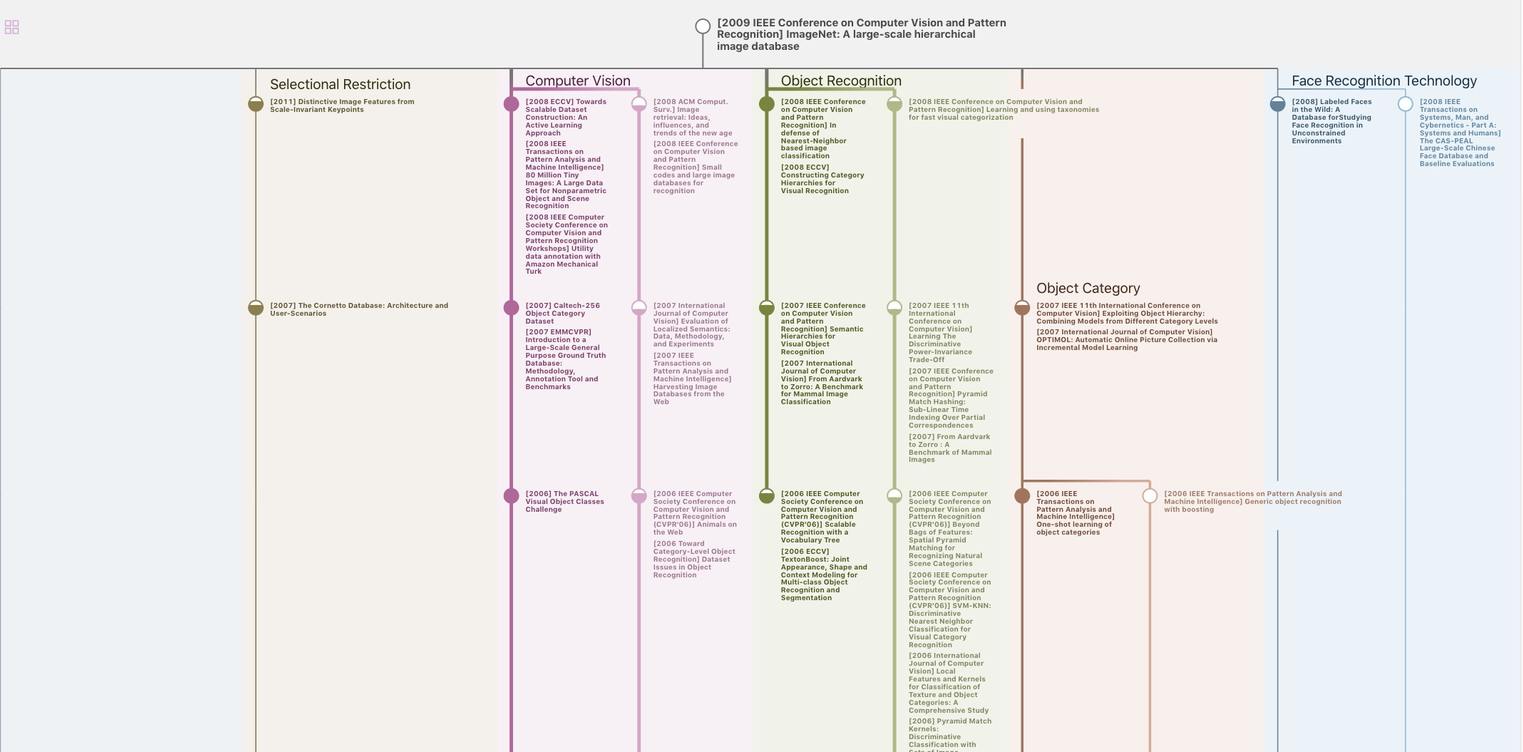
生成溯源树,研究论文发展脉络
Chat Paper
正在生成论文摘要