An Experimental Study on Hyper-parameter Optimization for Breast Cancer Risk Prediction using IBM Snap Machine Learning Techniques
2022 Second International Conference on Computer Science, Engineering and Applications (ICCSEA)(2022)
摘要
Worldwide approximately 1 in 6 women will succumb to Breast Cancer (BC), making it one of the most lethal illnesses in the female population. To avoid and receive adequate clinical diagnostic therapy, it is imperative that early detection be made possible. Medical applications rely heavily on Machine Learning (ML) for risk prediction, therapy recommendation, and critical decision assistance. With the Wisconsin BC diagnosis dataset from UCI (University of California Irvine) repository, we have evaluated two IBM Watson’s ML algorithms such as Snap Logistic Regression (LR) and Snap SVM Classifier. The algorithm’s goal is to identify the diseased cells as Benign (B) or Malignant (M) that is either non-cancerous or cancerous. Performance metrics such as Accuracy, AROC (Asymptotic Receiver Operating Characteristic), Precision, Recall, F1, Average Precision, and Log Loss are computed to measure the model outperformed the rest of the algorithm in all other measures, with a cross validation score of 98.2 percent.
更多查看译文
关键词
Breast Cancer Prediction,Snap Logistic Regression,Snap SVM Classifier,IBM Watson,Machine Learning
AI 理解论文
溯源树
样例
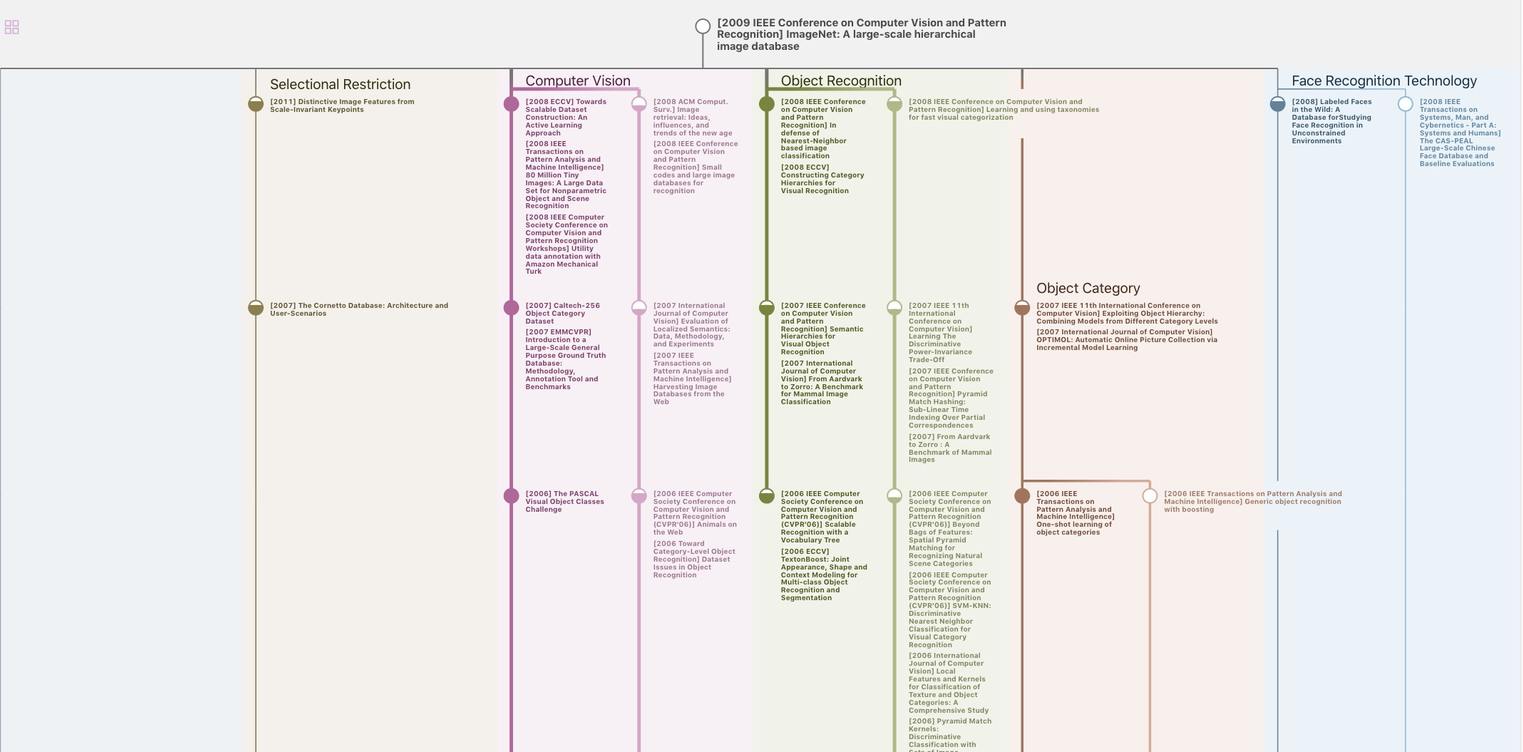
生成溯源树,研究论文发展脉络
Chat Paper
正在生成论文摘要