Targeted Maximum Likelihood Estimation for Causal Inference in Survival and Competing Risks Analysis
LIFETIME DATA ANALYSIS(2024)
摘要
Targeted maximum likelihood estimation (TMLE) provides a general methodology for estimation of causal parameters in presence of high-dimensional nuisance parameters. Generally, TMLE consists of a two-step procedure that combines data-adaptive nuisance parameter estimation with semiparametric efficiency and rigorous statistical inference obtained via a targeted update step. In this paper, we demonstrate the practical applicability of TMLE based causal inference in survival and competing risks settings where event times are not confined to take place on a discrete and finite grid. We focus on estimation of causal effects of time-fixed treatment decisions on survival and absolute risk probabilities, considering different univariate and multidimensional parameters. Besides providing a general guidance to using TMLE for survival and competing risks analysis, we further describe how the previous work can be extended with the use of loss-based cross-validated estimation, also known as super learning, of the conditional hazards. We illustrate the usage of the considered methods using publicly available data from a trial on adjuvant chemotherapy for colon cancer. R software code to implement all considered algorithms and to reproduce all analyses is available in an accompanying online appendix on Github.
更多查看译文
关键词
TMLE,Semiparametric efficiency,Survival analysis,Competing risks,Super learning,Highly adaptive lasso,Causal inference,Average treatment effects
AI 理解论文
溯源树
样例
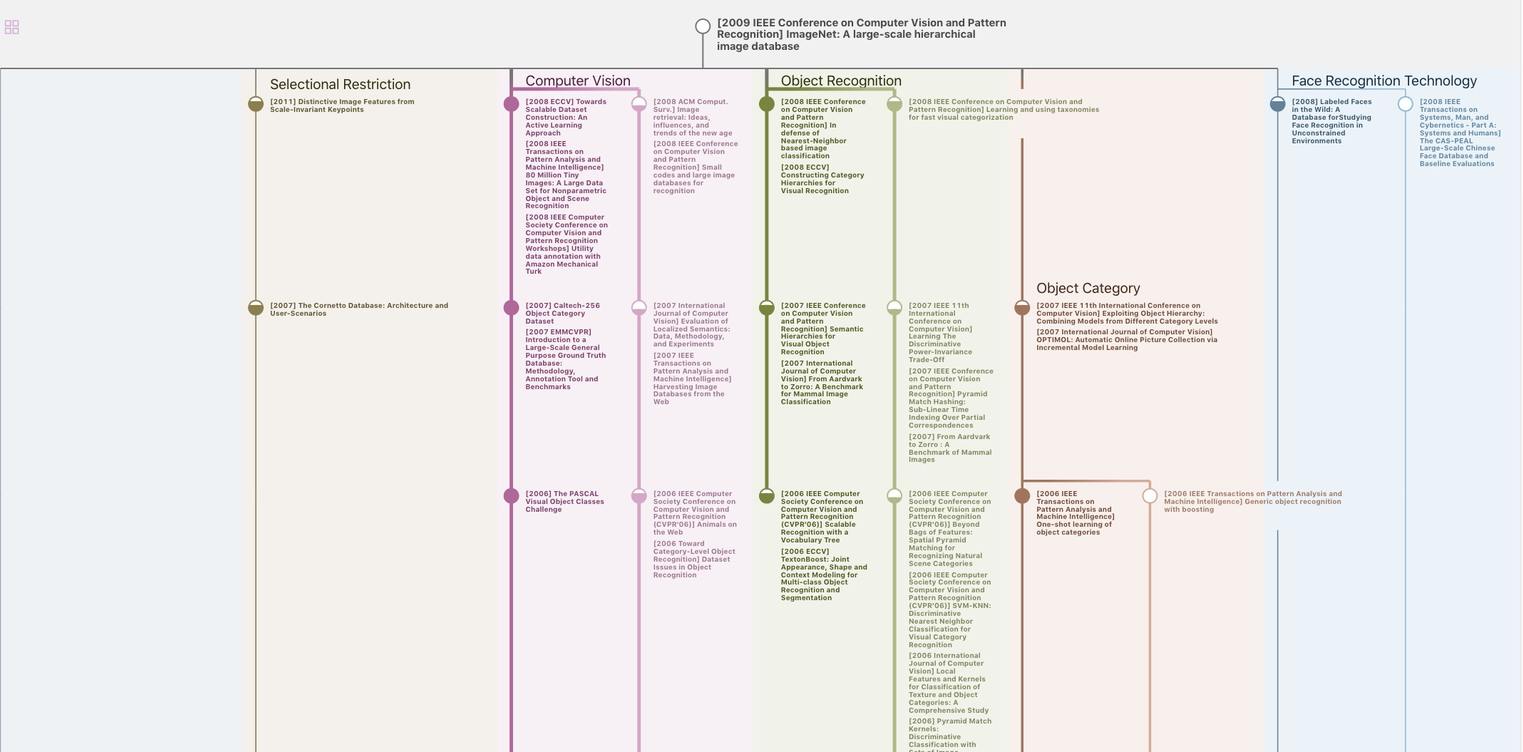
生成溯源树,研究论文发展脉络
Chat Paper
正在生成论文摘要