A Machine-Learning Approach Clarifies Interactions Between Contaminants of Emerging Concern
One earth(2022)
摘要
Humans and biotas are exposed to a cocktail of contaminants of emerging concern (CECs), but mixture regulation is lagging behind. This is largely attributed to inadequate experimental data of mixture risk; revealing intricate interactions among CECs in mixtures with random combinations remains a formidable challenge. Here, we propose a new framework comprised of 5,720 lab tests of mixture risk for 100 CECs with random combinations, extended prediction of mixture risk in any CEC combination via a new machine learning model, and validation in field sites. We identify a general concave-down relationship between CEC number and ecological risk of algae, invertebrates, and fish under different lab conditions and in more than 900 field sites worldwide. We propose a new “redundancy mechanism” to clarify interactions among CECs, suggesting implications in grouping CECs by action mode for developing mixture regulatory frameworks. Our framework provides a blueprint for addressing cocktail effects of multi-factors with random combinations in different disciplines.
更多查看译文
关键词
random selection test,neural network model,chemical cocktails,global mixture risk,biodiversity,primary production,carbon/nitrogen fixation,field validation
AI 理解论文
溯源树
样例
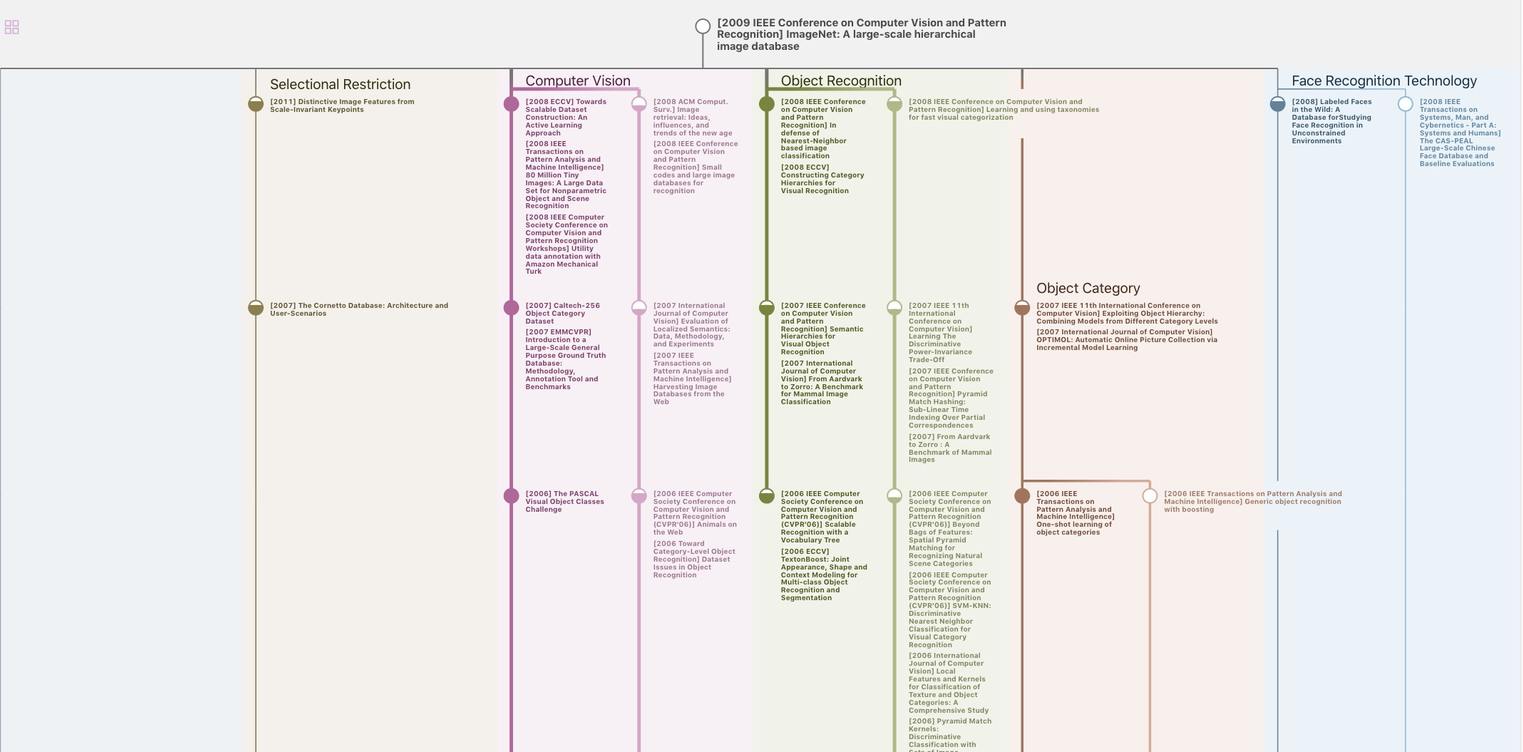
生成溯源树,研究论文发展脉络
Chat Paper
正在生成论文摘要