PhotoRedshift-MML: A Multimodal Machine Learning Method for Estimating Photometric Redshifts of Quasars
Monthly Notices of the Royal Astronomical Society(2022)
摘要
We propose a Multimodal Machine Learning method for estimating the Photometric Redshifts of quasars (PhotoRedshift-MML for short), which has long been the subject of many investigations. Our method includes two main models, i.e. the feature transformation model by multimodal representation learning, and the photometric redshift estimation model by multimodal transfer learning. The prediction accuracy of the photometric redshift was significantly improved owing to the large amount of information offered by the generated spectral features learned from photometric data via the MML. A total of 415 930 quasars from Sloan Digital Sky Survey (SDSS) Data Release 17, with redshifts between 1 and 5, were screened for our experiments. We used |Delta z| = |(z(phot) - z(spec))/(1 + z(spec))| to evaluate the redshift prediction and demonstrated a $4.04{{\ \rm per\ cent}}$ increase in accuracy. With the help of the generated spectral features, the proportion of data with |Delta z| < 0.1 can reach $84.45{{\ \rm per\ cent}}$ of the total test samples, whereas it reaches $80.41{{\ \rm per\ cent}}$ for single-modal photometric data. Moreover, the Root Mean Square (RMS) of |Delta z| is shown to decrease from 0.1332 to 0.1235. Our method has the potential to be generalized to other astronomical data analyses such as galaxy classification and redshift prediction.
更多查看译文
关键词
methods: data analysis,methods: statistical,techniques: photometric,surveys
AI 理解论文
溯源树
样例
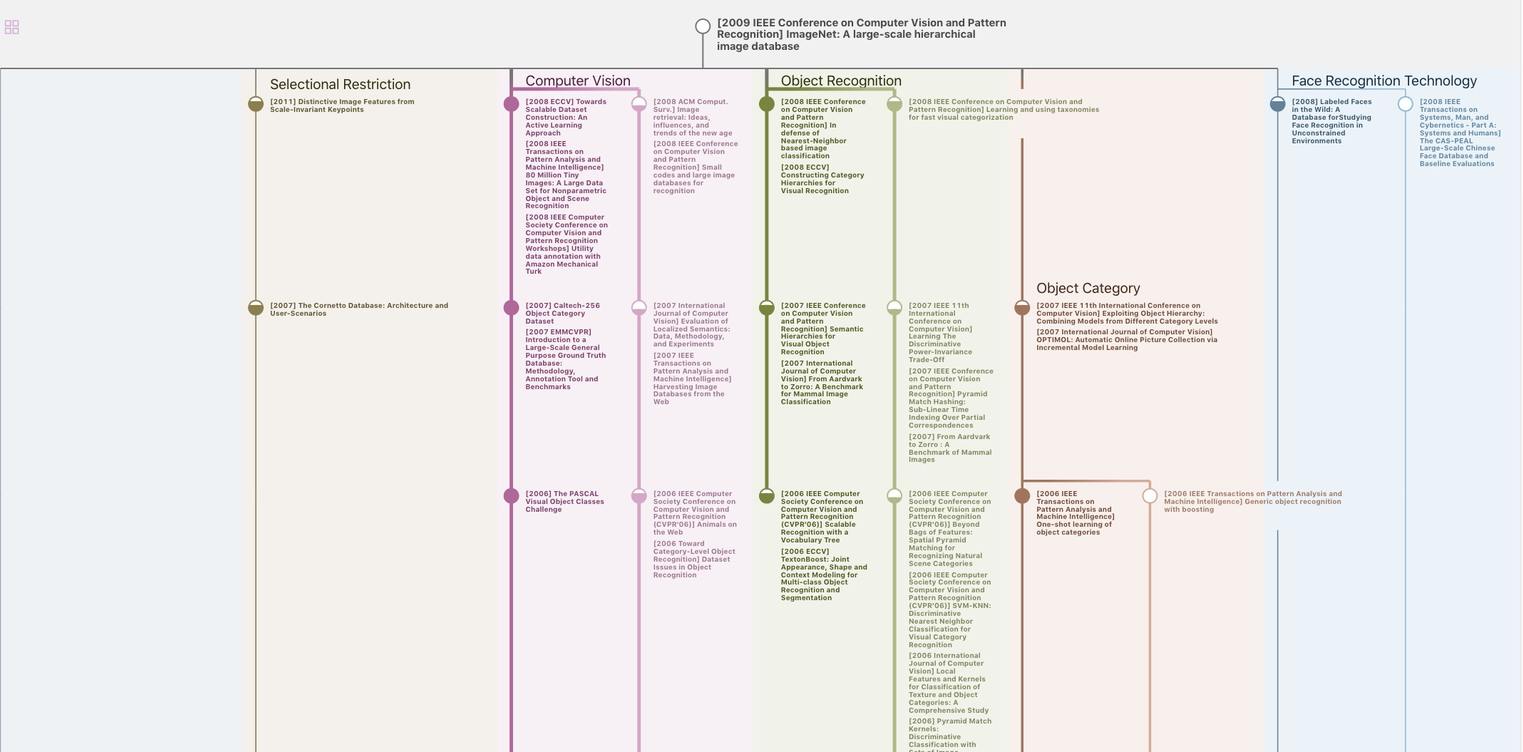
生成溯源树,研究论文发展脉络
Chat Paper
正在生成论文摘要