Variable Markov dynamics as a multi-focal lens to map multi-scale complex networks
arxiv(2022)
摘要
From traffic flows on road networks to electrical signals in brain networks, many real-world networks contain modular structures of different sizes and densities. In the networks where modular structures emerge due to coupling between nodes with similar dynamical functions, we can identify them using flow-based community detection methods. However, these methods implicitly assume that communities are dense or clique-like which can shatter sparse communities due to a field-of-view limit inherent in one-step dynamics. Taking multiple steps with shorter or longer Markov time enables us to effectively zoom in or out to capture small or long-range communities. However, zooming out to avoid the field-of-view limit comes at the expense of introducing or increasing a lower resolution limit. Here we relax the constant Markov time constraint and introduce variable Markov dynamics as a multi-focal lens to capture functional communities in networks with a higher range of scales. With variable Markov time, a random walker can keep one-step dynamics in dense areas to avoid the resolution limit and move faster in sparse areas to detect long-range modular structures and prevent the field-of-view limit. We analyze the performance of variable Markov time using the flow-based community detection method called the map equation. We have implemented the map equation with variable Markov time in the search algorithm Infomap without any complexity overhead and tested its performance on synthetic and real-world networks from different domains. Results show that it outperforms the standard map equation in networks with constrained structures and locally sparse regions. In addition, the method estimates the optimal Markov time and avoids parameter tuning.
更多查看译文
关键词
complex networks,markov,dynamics,multi-focal,multi-scale
AI 理解论文
溯源树
样例
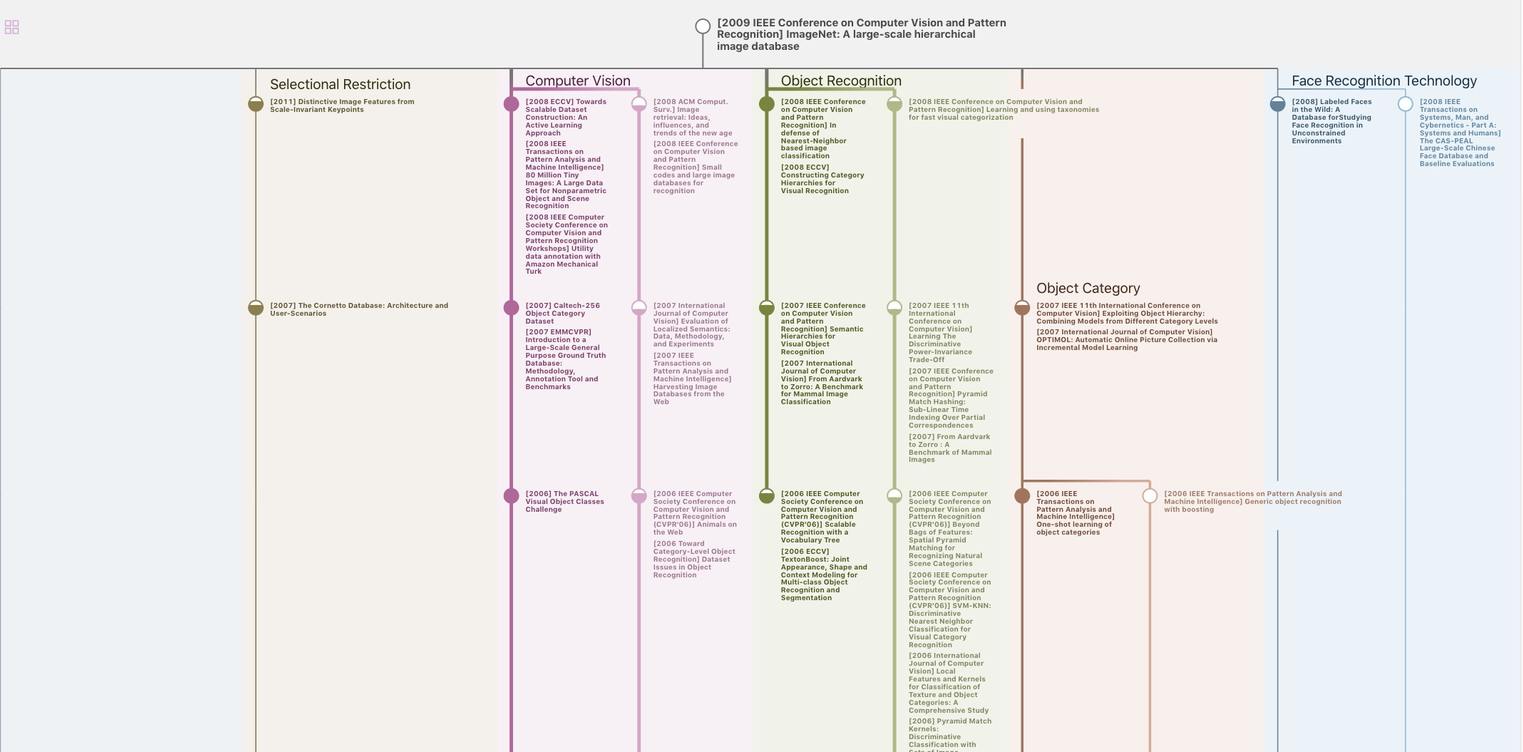
生成溯源树,研究论文发展脉络
Chat Paper
正在生成论文摘要