Resolution enhancement of one-dimensional molecular wavefunctions in plane-wave basis via quantum machine learning
arxiv(2022)
摘要
Super-resolution is a machine-learning technique in image processing which generates high-resolution images from low-resolution images. Inspired by this approach, we perform a numerical experiment of quantum machine learning, which takes low-resolution (low plane-wave energy cutoff) one-particle molecular wavefunctions in plane-wave basis as input and generates high-resolution (high plane-wave energy cutoff) wavefunctions in fictitious one-dimensional systems, and study the performance of different learning models. We show that the trained models can generate wavefunctions having higher fidelity values with respect to the ground-truth wavefunctions than a simple linear interpolation, and the results can be improved both qualitatively and quantitatively by including data-dependent information in the ansatz. On the other hand, the accuracy of the current approach deteriorates for wavefunctions calculated in electronic configurations not included in the training dataset. We also discuss the generalization of this approach to many-body electron wavefunctions.
更多查看译文
关键词
quantum machine learning,resolution,one-dimensional,plane-wave
AI 理解论文
溯源树
样例
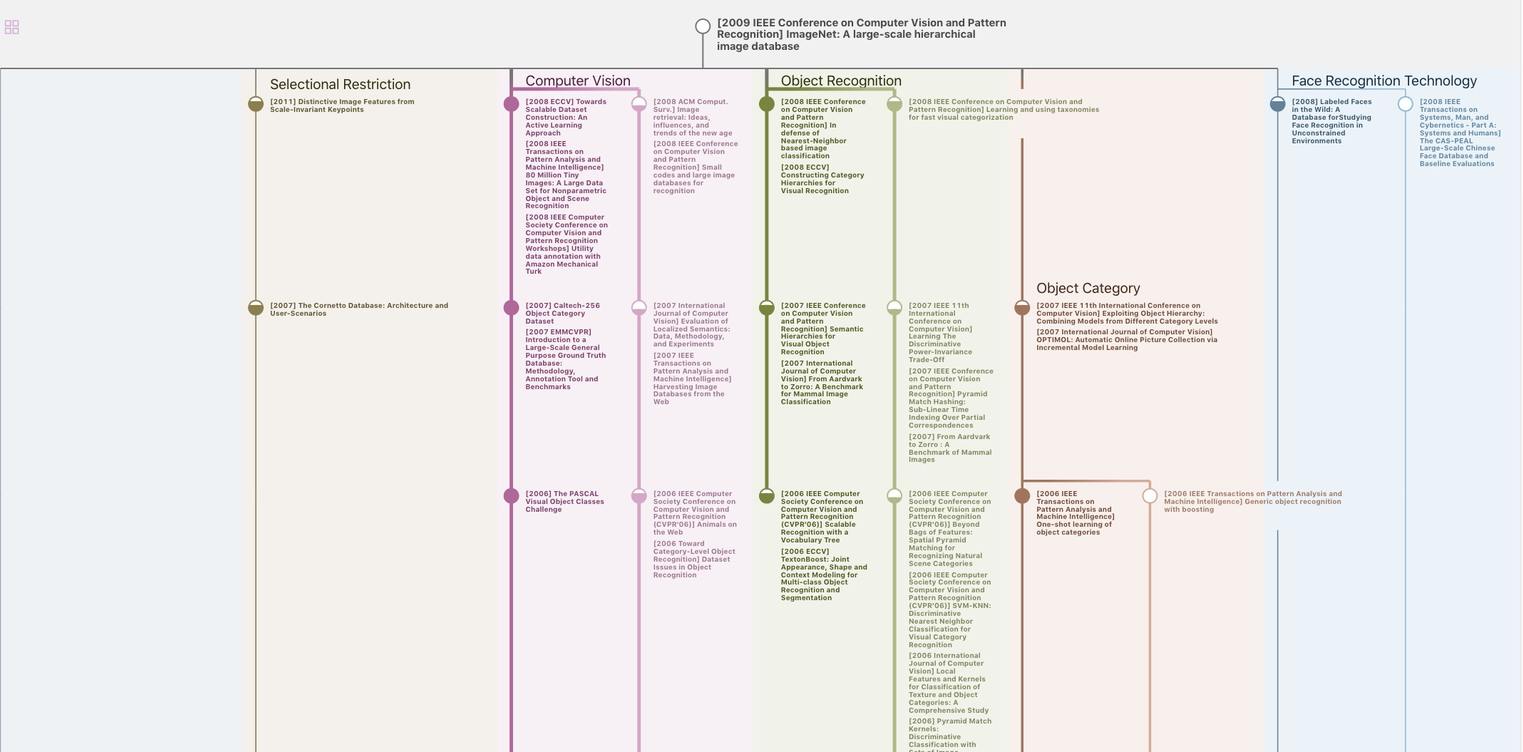
生成溯源树,研究论文发展脉络
Chat Paper
正在生成论文摘要