Estimating Multiclass Service Demand Distributions Using Markovian Arrival Processes
ACM Transactions on Modeling and Computer Simulation(2023)
摘要
Building performance models for software services in DevOps is costly and error-prone. Accurate service demand distribution estimation is critical to precisely modeling queueing behaviors and performance prediction. However, current estimation methods focus on capturing the mean service demand, disregarding higher-order moments of the distribution that still can largely affect prediction accuracy. To address this limitation, we propose to estimate higher moments of the service demand distribution for a microservice from monitoring traces. We first generate a closed queueing model to abstract software performance and use it to model the departure process of requests completed by the software service as a Markovian arrival process (MAP). This allows formulating the estimation of service demand into an optimization problem, which aims to find the first multiple moments of the service demand distribution that maximize the likelihood of the MAP using generated the measured inter-departure times. We then estimate the service demand distribution for different classes of service with a maximum likelihood algorithm and novel heuristics to mitigate the computational cost of the optimization process for scalability. We apply our method to real traces from a microservice-based application and demonstrate that its estimations lead to greater prediction accuracy than exponential distributions assumed in traditional service demand estimation approaches for software services.
更多查看译文
关键词
Service demand distribution,Markovian arrival process,maximum likelihood estimation,queueing models,performance
AI 理解论文
溯源树
样例
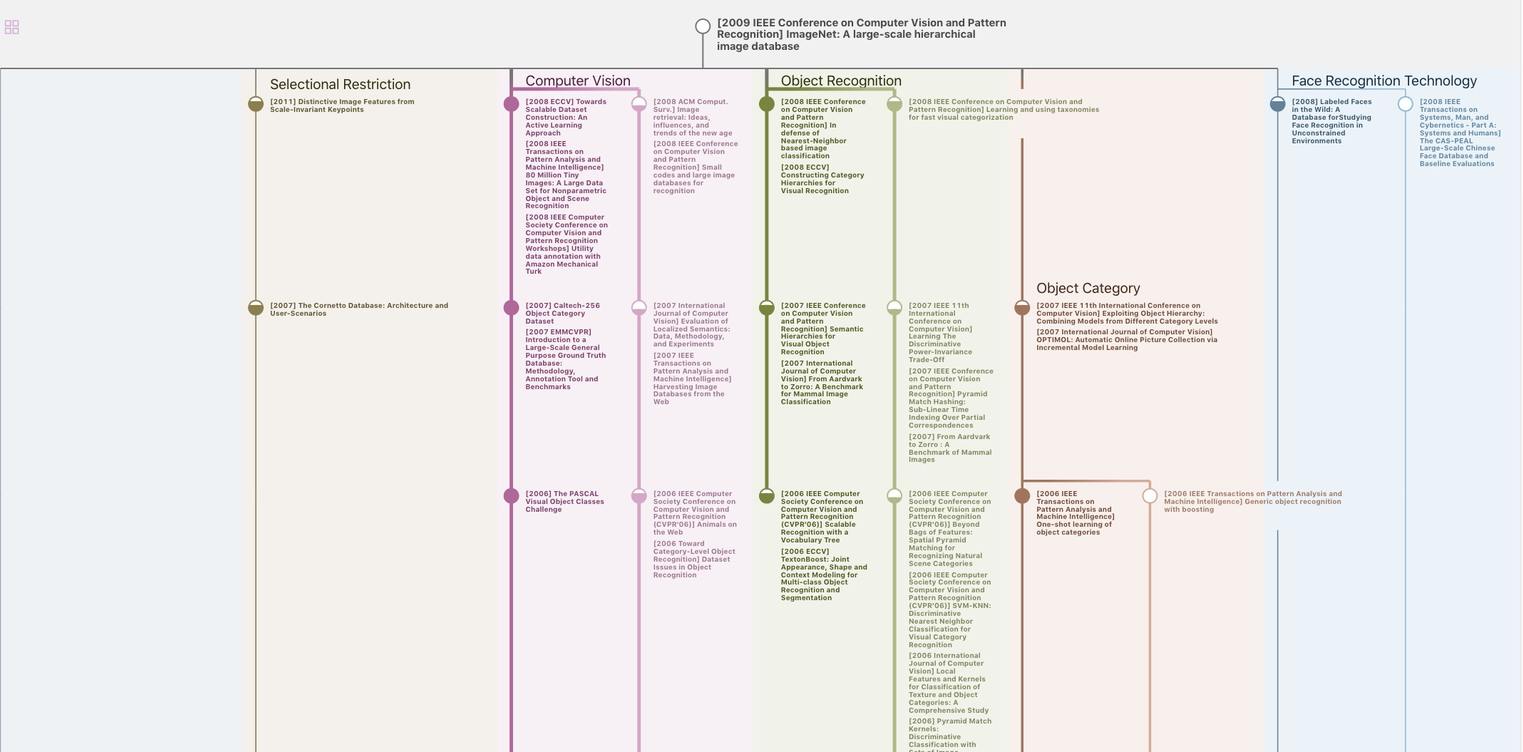
生成溯源树,研究论文发展脉络
Chat Paper
正在生成论文摘要