An attention-guided CNN framework for segmentation and grading of glioma using 3D MRI scans.
IEEE/ACM transactions on computational biology and bioinformatics(2022)
摘要
Glioma has emerged as the deadliest form of brain tumor for human beings. Timely diagnosis of these tumors is a major step towards effective oncological treatment. Magnetic Resonance Imaging (MRI) typically offers a non-invasive inspection of brain lesions. However, manual inspection of tumors from MRI scans requires a large amount of time and it is also an error-prone process. Therefore, automated diagnosis of tumors plays a crucial role in clinical management and surgical interventions of gliomas. In this study, we propose a Convolutional Neural Network (CNN)-based framework for non-invasive grading of tumors from 3D MRI scans. The proposed framework incorporates two novel CNN architectures. The first CNN architecture performs the segmentation of tumors from multimodel MRI volumes. The proposed segmentation network leverages the spatial and channel attention modules to recalibrate the feature maps across the layers. The second network utilizes the multi-task learning strategy to perform the classification based on the three glioma grading tasks which include characterization of tumor into low-grade or high-grade, identification of 1p19q, and Isocitrate Dehydrogenase (IDH) status. We have carried out several experiments to evaluate the performance of our method. Extensive experimental observations indicate that the proposed framework achieves better performance than several state-of-the-art methods. We have also executed Welch's- t test to show the statistical significance of grading results. The source code of this study is available at https://github.com/prasunc/Gliomanet.
更多查看译文
关键词
Attention,convolutional neural network,glioma grading,magnetic resonance imaging,multi-task learning,segmentation
AI 理解论文
溯源树
样例
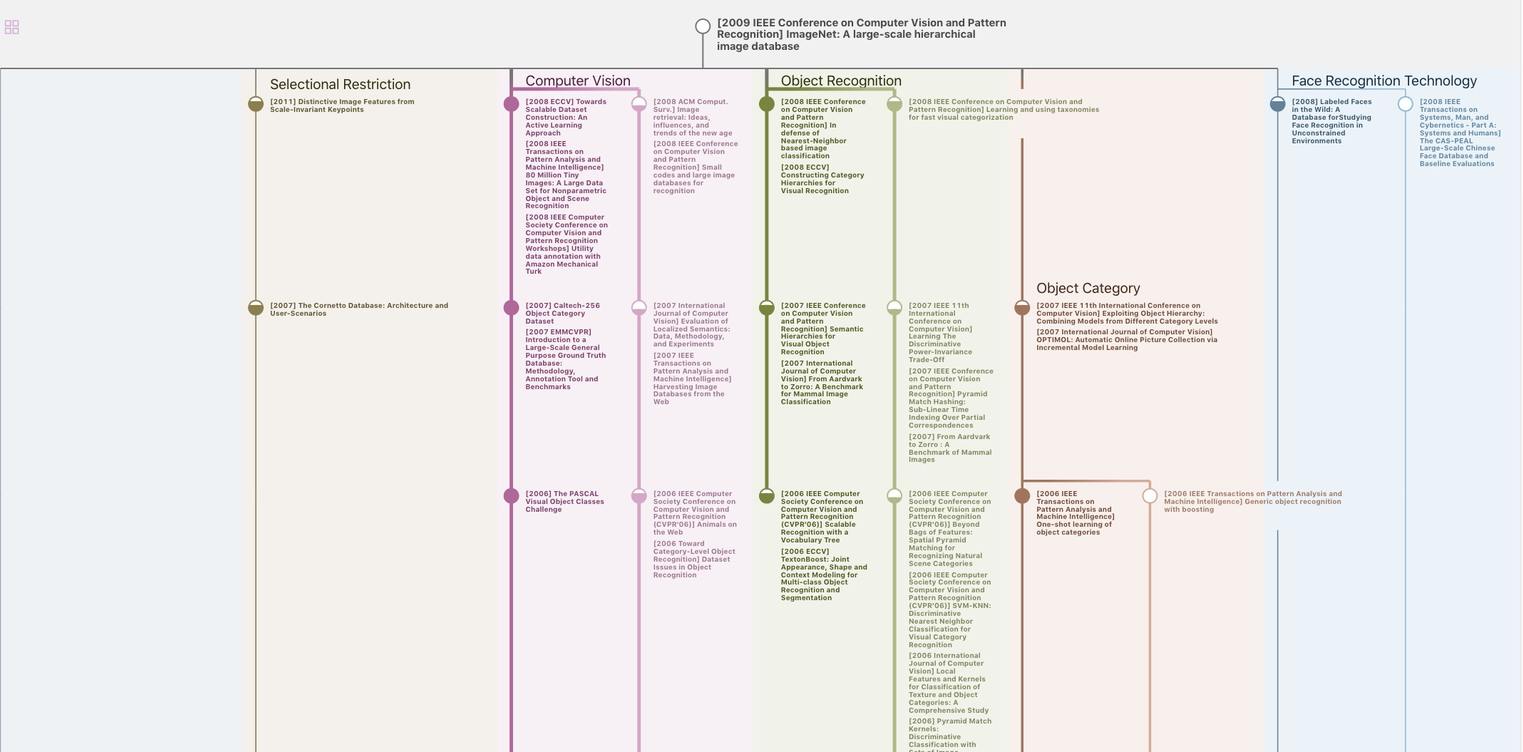
生成溯源树,研究论文发展脉络
Chat Paper
正在生成论文摘要