Climbing four tops with graph networks, transformers and pairwise features
arxiv(2022)
摘要
The ATLAS and CMS collaborations at CERN aim to measure the production of four top quarks in a single LHC collision. The sensitivity to this process is heavily reliant on the classification accuracy of these events in relation to the vast background from other Standard Model processes. The four top process is thus a prime candidate for the implementation and comparison of more advanced classification techniques based on deep learning, rather than the commonplace traditional methods. In this paper, we present a data set and comparison of a variety of methods that have recently emerged. We find that a transformer architecture (Particle Transformer) and a graph neural network (Particle Net), with the inclusion of pairwise features, perform best. Compared to the performance of a baseline boosted decision tree, the improvement in signal efficiency equates to a gain of $30-50\%$ in integrated luminosity.
更多查看译文
关键词
graph networks,pairwise
AI 理解论文
溯源树
样例
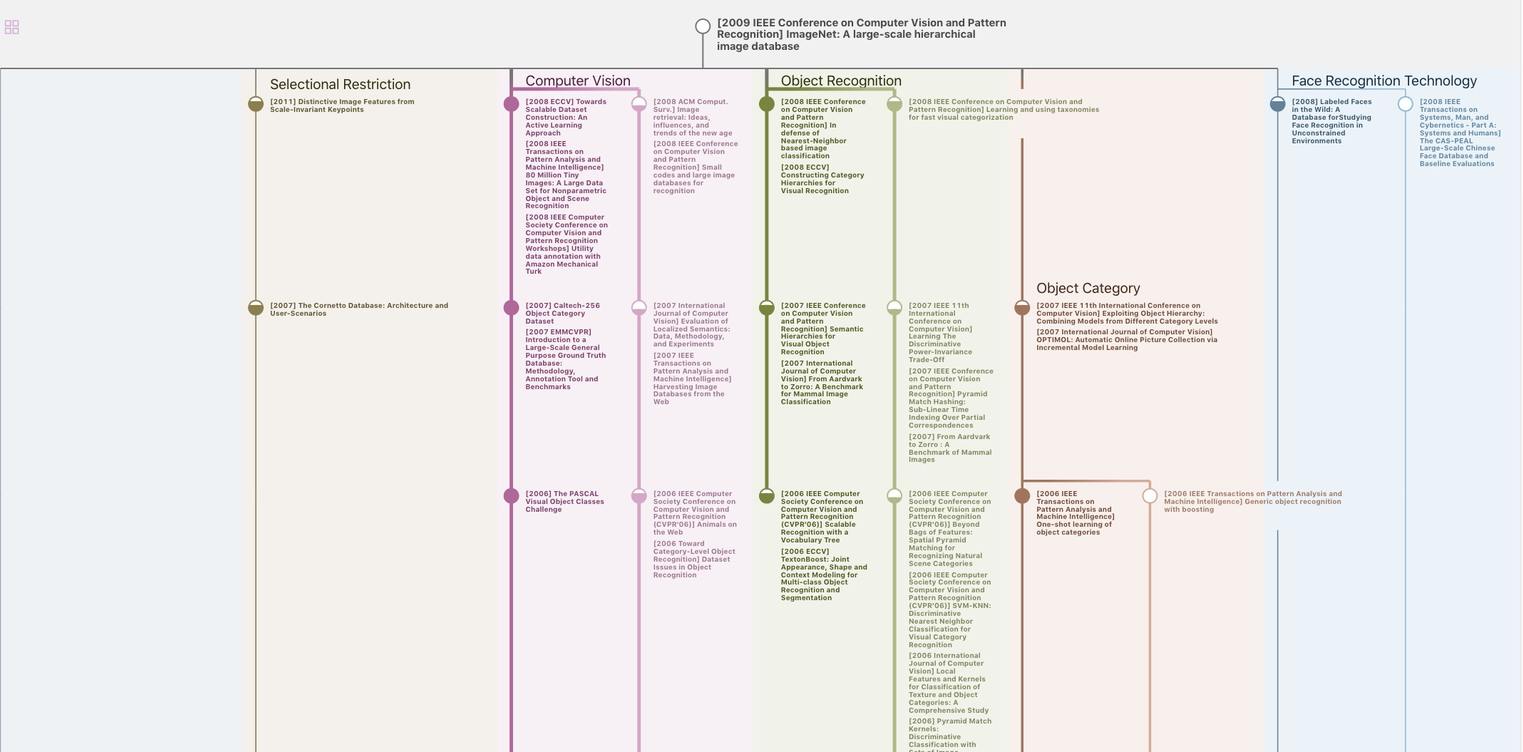
生成溯源树,研究论文发展脉络
Chat Paper
正在生成论文摘要