Safe and Adaptive Decision-Making for Optimization of Safety-Critical Systems: The ARTEO Algorithm
arxiv(2023)
摘要
We consider the problem of decision-making under uncertainty in an environment with safety constraints. Many business and industrial applications rely on real-time optimization to improve key performance indicators. In the case of unknown characteristics, real-time optimization becomes challenging, particularly because of the satisfaction of safety constraints. We propose the ARTEO algorithm, where we cast multi-armed bandits as a mathematical programming problem subject to safety constraints and learn the unknown characteristics through exploration while optimizing the targets. We quantify the uncertainty in unknown characteristics by using Gaussian processes and incorporate it into the cost function as a contribution which drives exploration. We adaptively control the size of this contribution in accordance with the requirements of the environment. We guarantee the safety of our algorithm with a high probability through confidence bounds constructed under the regularity assumptions of Gaussian processes. We demonstrate the safety and efficiency of our approach with two case studies: optimization of electric motor current and real-time bidding problems. We further evaluate the performance of ARTEO compared to a safe variant of upper confidence bound based algorithms. ARTEO achieves less cumulative regret with accurate and safe decisions.
更多查看译文
关键词
optimization,algorithm,decision-making decision-making,safety-critical
AI 理解论文
溯源树
样例
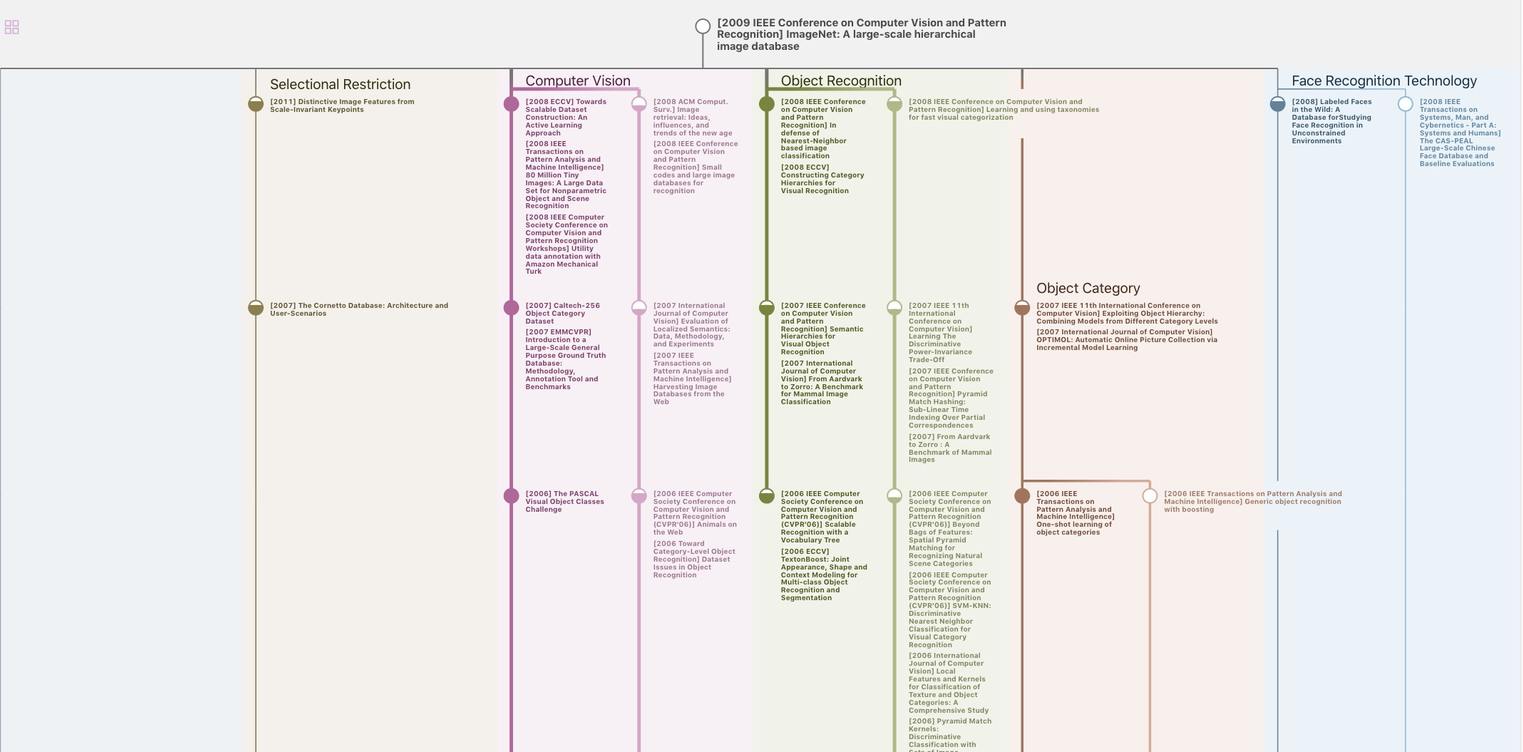
生成溯源树,研究论文发展脉络
Chat Paper
正在生成论文摘要