FastPicker: Adaptive independent two-stage video-to-video summarization for efficient action recognition
Neurocomputing(2023)
摘要
Video datasets suffer from huge inter-frame redundancy, which prevents deep networks from learning effectively and increases computational costs. Therefore, several methods adopt random/uniform frame sampling or key-frame selection techniques. Unfortunately, most of the learnable frame selection methods are customized for specific models and lack generality, independence, and scalability. In this paper, we propose a novel two-stage video-to-video summarization method termed FastPicker, which can efficiently select the most discriminative and representative frames for better action recognition. Independently, the discriminative frames are selected in the first stage based on the inter-frame motion computation, whereas the representative frames are selected in the second stage using a novel Transformer-based model. Learnable frame embeddings are proposed to estimate each frame contribution to the final video classification certainty. Consequently, the frames with the largest contributions are the most representative. The proposed method is carefully evaluated by summarizing several action recognition datasets and using them to train various deep models with several backbones. The experimental results demonstrate a remarkable performance boost on Kinetics400, Something-Something-v2, ActivityNet-1.3, UCF-101, and HMDB51 datasets, e.g., FastPicker downsizes Kinetics400 by 78.7% of its size while improving the human activity recognition.
更多查看译文
关键词
Action recognition,Video-to-video summarization,Discriminative frame selection,Representative frame selection,Deep learning
AI 理解论文
溯源树
样例
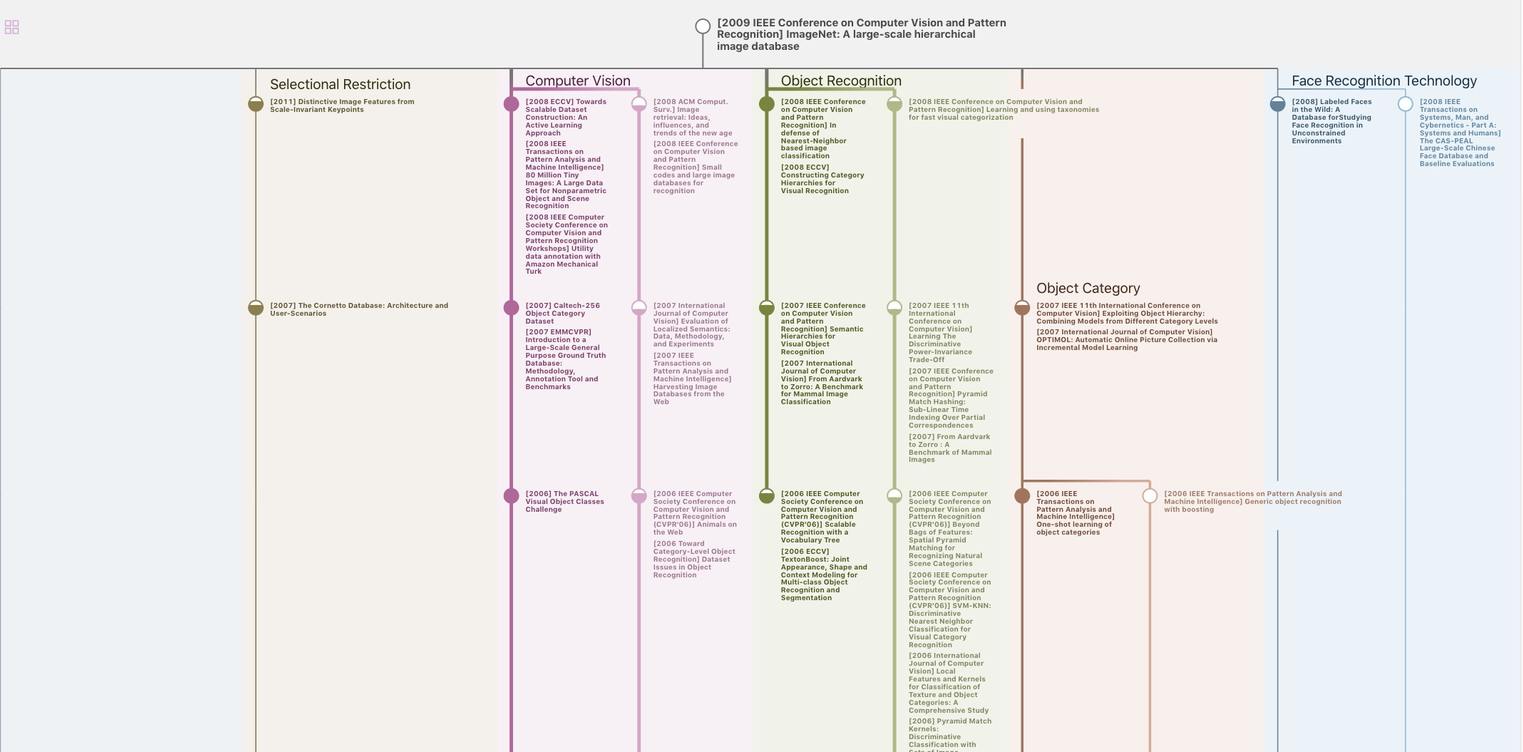
生成溯源树,研究论文发展脉络
Chat Paper
正在生成论文摘要