A Spatiotemporal Graph Attention Network Based on Synchronization for Epileptic Seizure Prediction
IEEE Journal of Biomedical and Health Informatics(2023)
摘要
Accurate early prediction of epileptic seizures can provide timely treatment for patients. Previous studies have mainly focused on a single temporal or spatial dimension, making it difficult to take both relationships into account. Therefore, the effective properties of electroencephalograms (EEGs) may not be fully evaluated. To solve this problem, we propose a spatiotemporal graph attention network (STGAT) based on synchronization. The spatial and functional connectivity information between EEG channels was extracted by using the phase locking values (PLVs) first, which allowed multichannel EEG signals to be modeled as graph signals. Afterward, the STGAT model was used to dynamically learn the temporal correlation properties of EEG sequences and explore the spatial topological structure information of multiple channels. Experimental results demonstrated that the STGAT model was able to obtain spatiotemporal correlations and achieve good results on two benchmark datasets. The accuracy, specificity and sensitivity were 98.74%, 99.21% and 98.87%, respectively, on the CHB-MIT dataset. Moreover, all evaluation indices of the private dataset had reached more than 98.8%, with the area under the curve (AUC) reaching 99.96%. The proposed method is superior or comparable to the state-of-the-art models. Extensive experiments demonstrate that our end-to-end automatic seizure prediction model can be extended to design clinical assistant decision systems.
更多查看译文
关键词
Epilepsy,graph attention network,seizure prediction,spatiotemporal correlation,synchronization
AI 理解论文
溯源树
样例
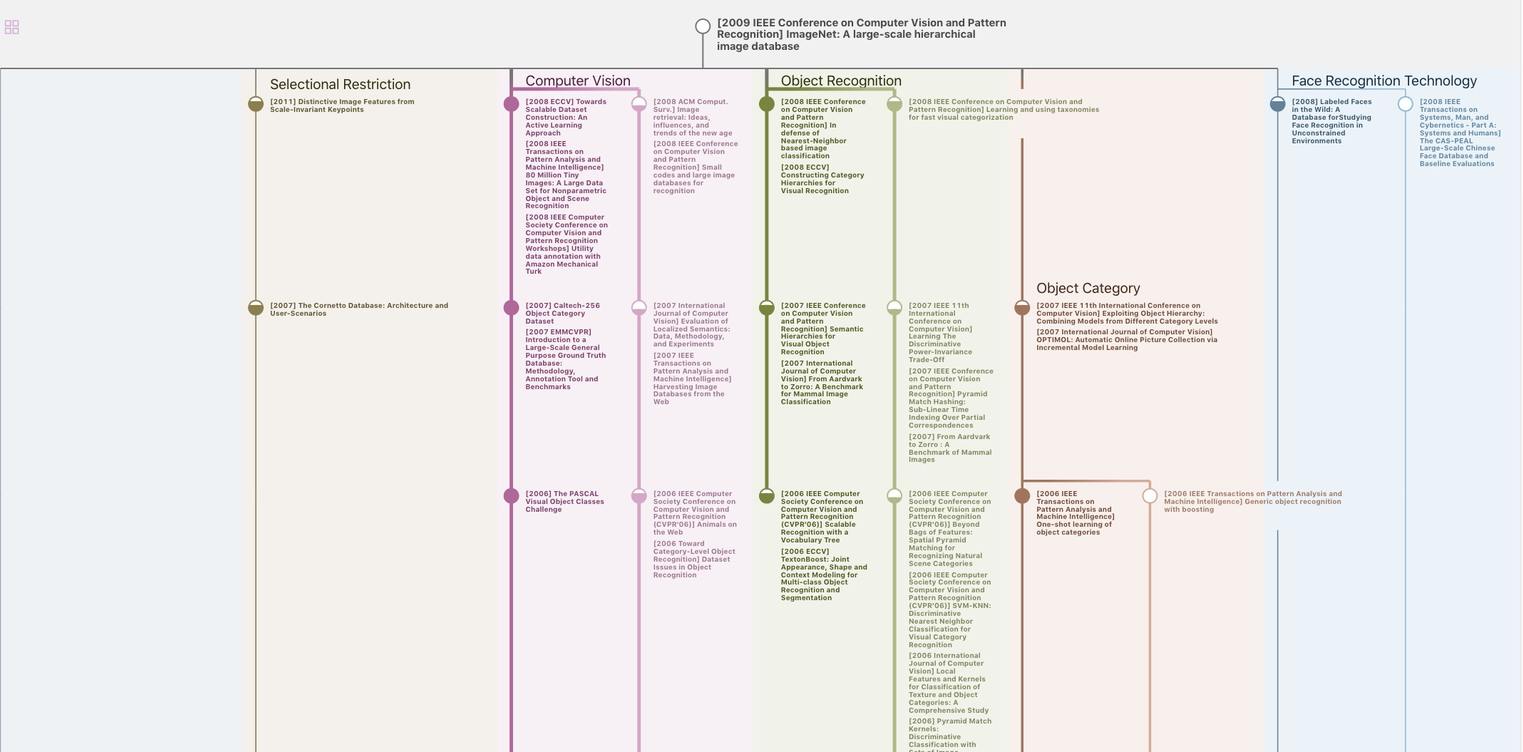
生成溯源树,研究论文发展脉络
Chat Paper
正在生成论文摘要