Combining Multi-Fidelity Modelling and Asynchronous Batch Bayesian Optimization
arxiv(2023)
摘要
Bayesian Optimization is a useful tool for experiment design. Unfortunately, the classical, sequential setting of Bayesian Optimization does not translate well into laboratory experiments, for instance battery design, where measurements may come from different sources and their evaluations may require significant waiting times. Multi-fidelity Bayesian Optimization addresses the setting with measurements from different sources. Asynchronous batch Bayesian Optimization provides a framework to select new experiments before the results of the prior experiments are revealed. This paper proposes an algorithm combining multi-fidelity and asynchronous batch methods. We empirically study the algorithm behavior, and show it can outperform single-fidelity batch methods and multi-fidelity sequential methods. As an application, we consider designing electrode materials for optimal performance in pouch cells using experiments with coin cells to approximate battery performance.
更多查看译文
关键词
Bayesian Optimization,Machine learning,Batch optimization,Asynchronous,Multi-fidelity
AI 理解论文
溯源树
样例
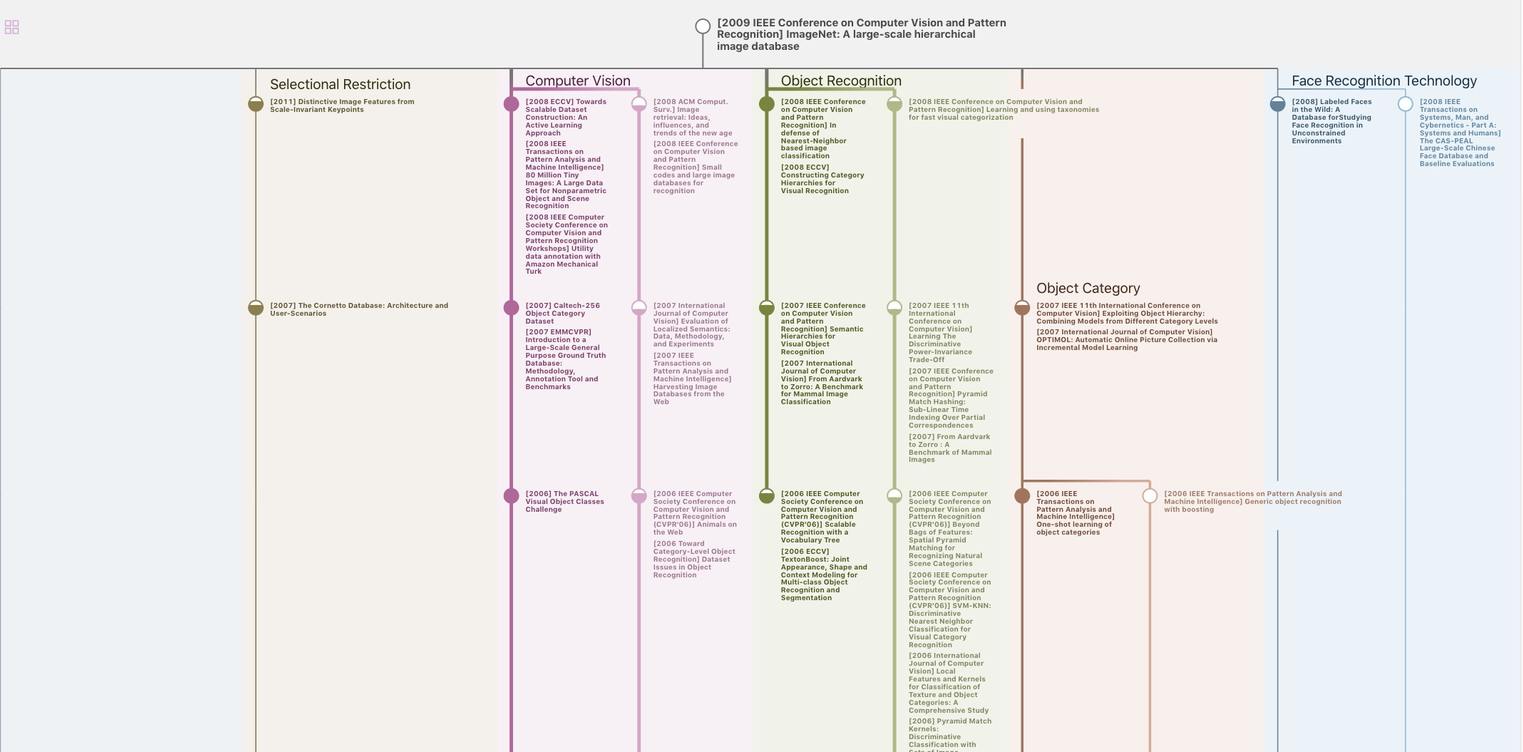
生成溯源树,研究论文发展脉络
Chat Paper
正在生成论文摘要