A New Graph Node Classification Benchmark: Learning Structure from Histology Cell Graphs
arxiv(2022)
摘要
We introduce a new benchmark dataset, Placenta, for node classification in an underexplored domain: predicting microanatomical tissue structures from cell graphs in placenta histology whole slide images. This problem is uniquely challenging for graph learning for a few reasons. Cell graphs are large (>1 million nodes per image), node features are varied (64-dimensions of 11 types of cells), class labels are imbalanced (9 classes ranging from 0.21% of the data to 40.0%), and cellular communities cluster into heterogeneously distributed tissues of widely varying sizes (from 11 nodes to 44,671 nodes for a single structure). Here, we release a dataset consisting of two cell graphs from two placenta histology images totalling 2,395,747 nodes, 799,745 of which have ground truth labels. We present inductive benchmark results for 7 scalable models and show how the unique qualities of cell graphs can help drive the development of novel graph neural network architectures.
更多查看译文
关键词
graph,node,classification,cell
AI 理解论文
溯源树
样例
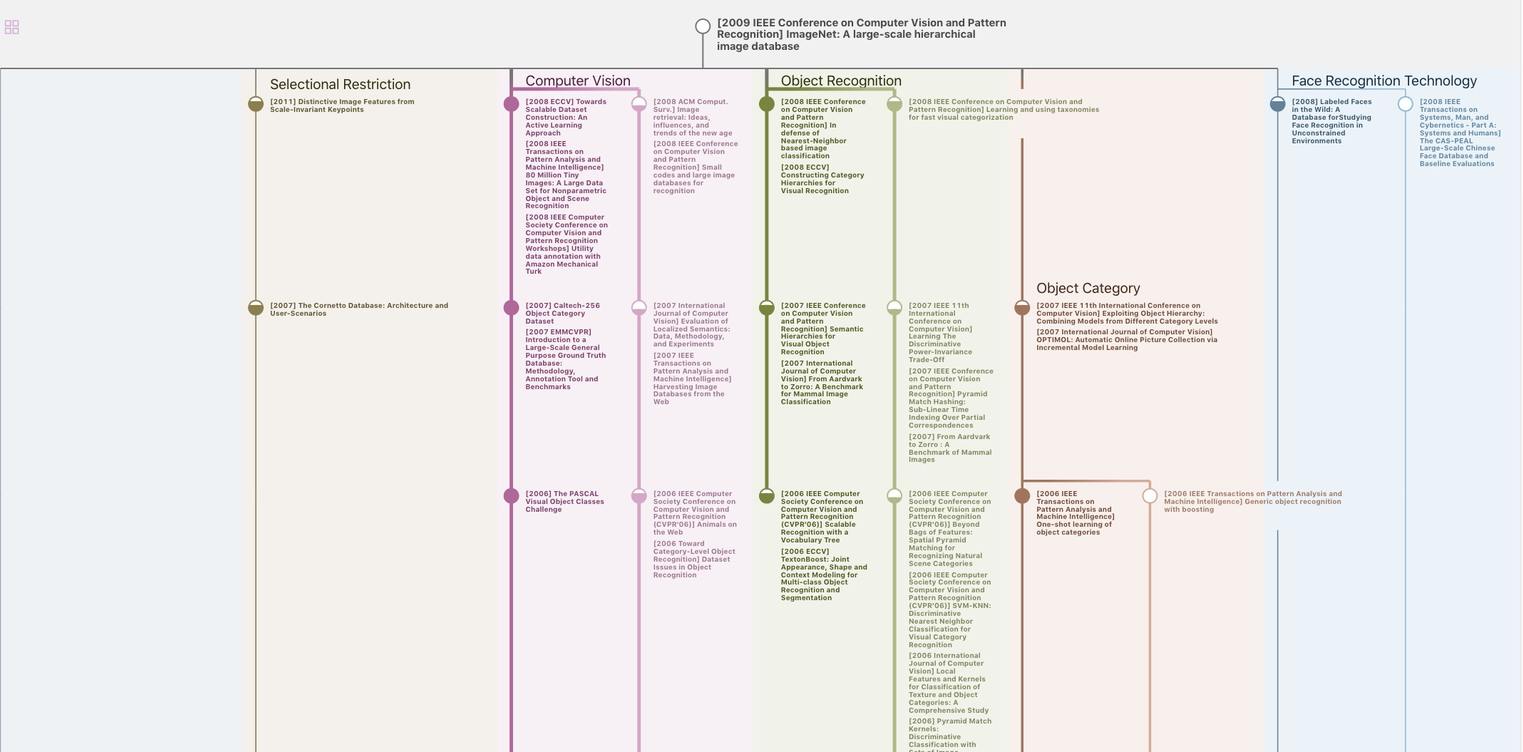
生成溯源树,研究论文发展脉络
Chat Paper
正在生成论文摘要