Enhancing and Adversarial: Improve ASR with Speaker Labels
arxiv(2023)
摘要
ASR can be improved by multi-task learning (MTL) with domain enhancing or domain adversarial training, which are two opposite objectives with the aim to increase/decrease domain variance towards domain-aware/agnostic ASR, respectively. In this work, we study how to best apply these two opposite objectives with speaker labels to improve conformer-based ASR. We also propose a novel adaptive gradient reversal layer for stable and effective adversarial training without tuning effort. Detailed analysis and experimental verification are conducted to show the optimal positions in the ASR neural network (NN) to apply speaker enhancing and adversarial training. We also explore their combination for further improvement, achieving the same performance as i-vectors plus adversarial training. Our best speaker-based MTL achieves 7\% relative improvement on the Switchboard Hub5'00 set. We also investigate the effect of such speaker-based MTL w.r.t. cleaner dataset and weaker ASR NN.
更多查看译文
关键词
asr,improve
AI 理解论文
溯源树
样例
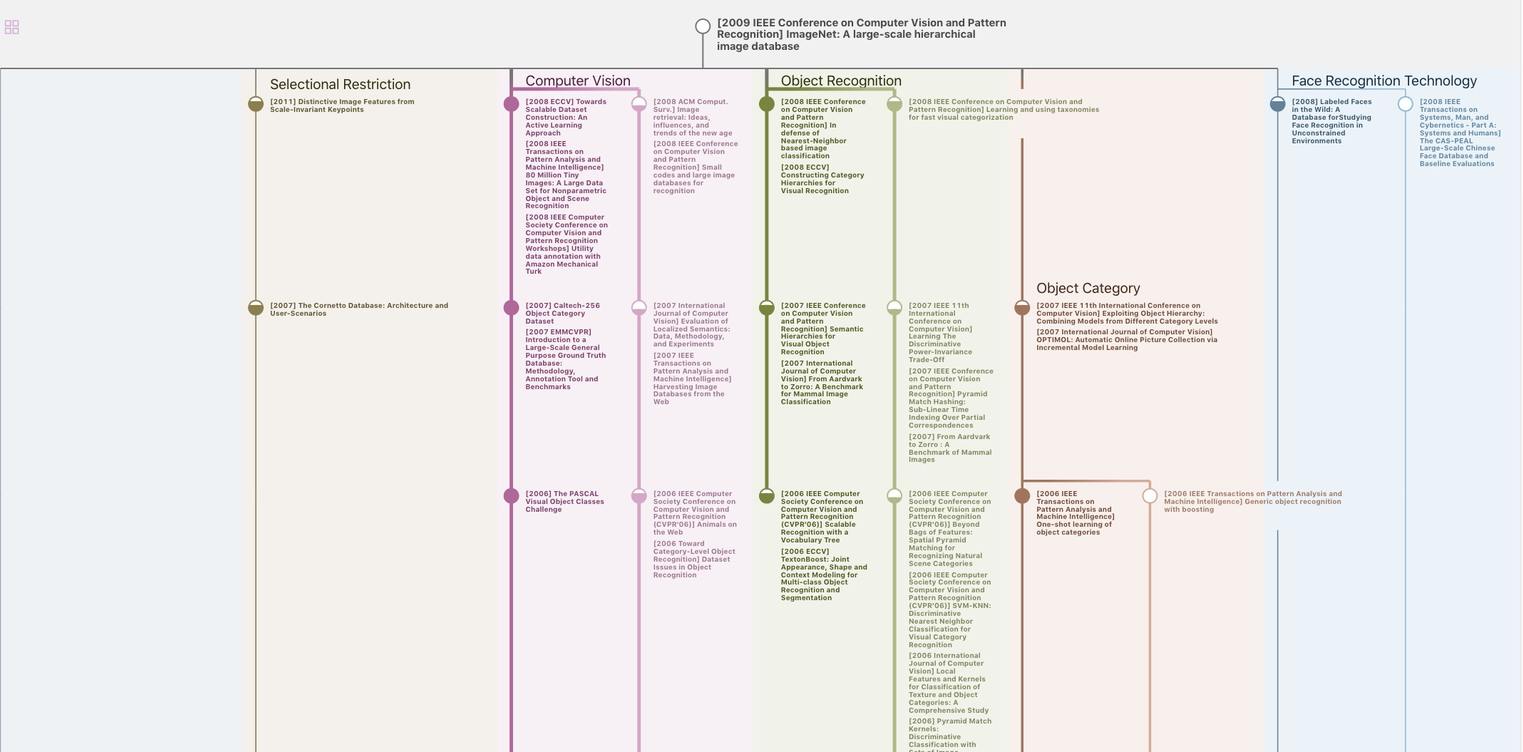
生成溯源树,研究论文发展脉络
Chat Paper
正在生成论文摘要