DistGNN-MB: Distributed Large-Scale Graph Neural Network Training on x86 via Minibatch Sampling
arxiv(2022)
摘要
Training Graph Neural Networks, on graphs containing billions of vertices and edges, at scale using minibatch sampling poses a key challenge: strong-scaling graphs and training examples results in lower compute and higher communication volume and potential performance loss. DistGNN-MB employs a novel Historical Embedding Cache combined with compute-communication overlap to address this challenge. On a 32-node (64-socket) cluster of $3^{rd}$ generation Intel Xeon Scalable Processors with 36 cores per socket, DistGNN-MB trains 3-layer GraphSAGE and GAT models on OGBN-Papers100M to convergence with epoch times of 2 seconds and 4.9 seconds, respectively, on 32 compute nodes. At this scale, DistGNN-MB trains GraphSAGE 5.2x faster than the widely-used DistDGL. DistGNN-MB trains GraphSAGE and GAT 10x and 17.2x faster, respectively, as compute nodes scale from 2 to 32.
更多查看译文
关键词
graph,neural network,training,large-scale
AI 理解论文
溯源树
样例
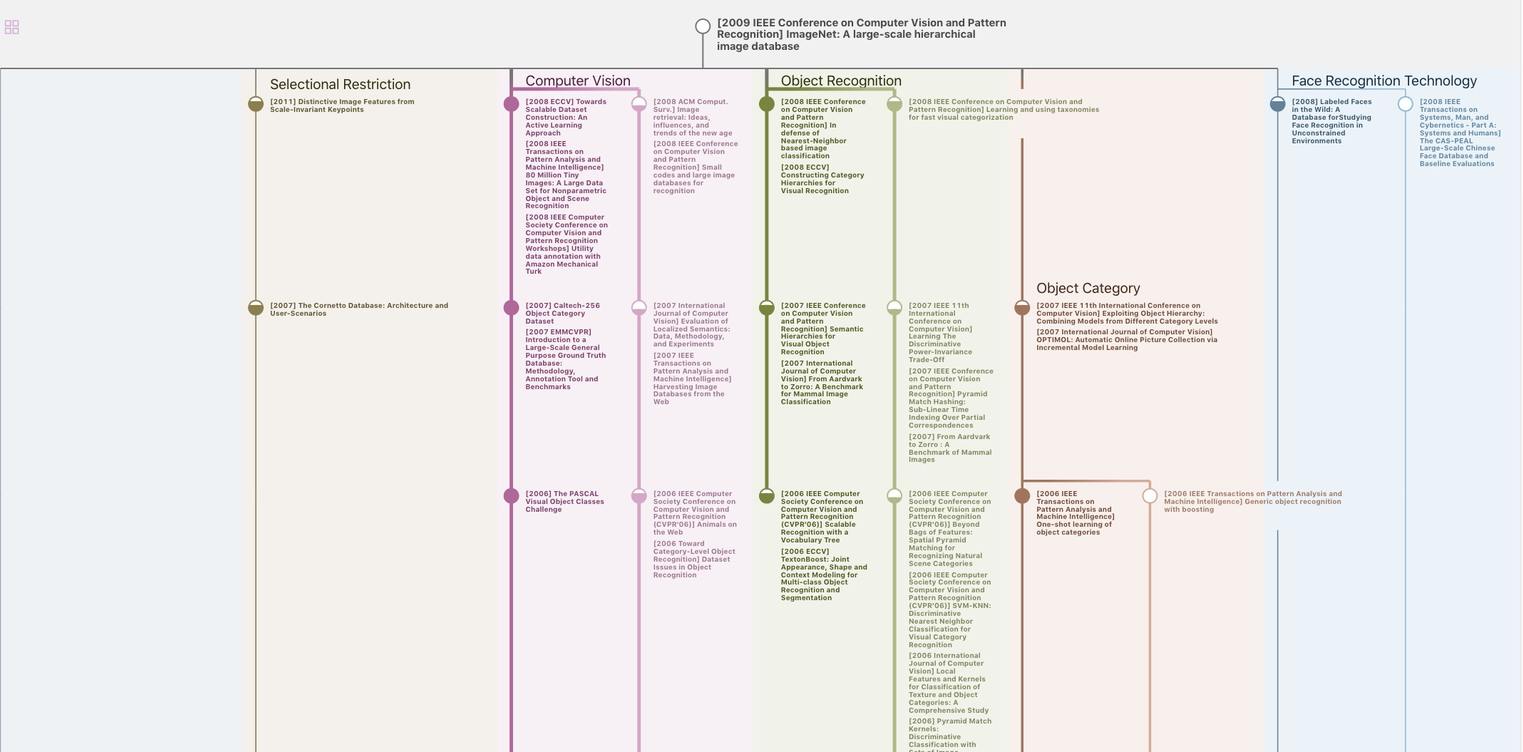
生成溯源树,研究论文发展脉络
Chat Paper
正在生成论文摘要