Defense Against Query-Based Black-Box Attack With Small Gaussian-Noise
2022 7th IEEE International Conference on Data Science in Cyberspace (DSC)(2022)
摘要
Although deep neural networks (DNNs) show un-precedented performance in various tasks, the vulnerability brought by adversarial samples to the models can incur security concerns, such as causing accidents by automatic driving, or in industrial manufacturing. Due to the discrete nature of textual data and the limitation of real-world access to the model, more and more attacks focus on iterative query attacks under black-box scenarios. The core idea is to query the models frequently to obtain the mapping relations between different input samples and the outputs, which guides the attack’s direction. Once we break down the input-output mapping relations, it will affect the attack’s query and local search process, which enables the defense against such attacks. With this motivation, we add tiny noise to the input samples to break the mapping relationship obtained by black-box attacks and we name the defense method as Gaussian Noise Perturbation Defence (GNPD). We analyze how the noise hinders the attack theoretically and demonstrate the effectiveness of the defense method on two datasets and three language models. The experimental results corroborate our analysis and our method has little impact to the performance of the original model.
更多查看译文
关键词
text categorization,adversarial defence,deep learning,query-based black-box attack
AI 理解论文
溯源树
样例
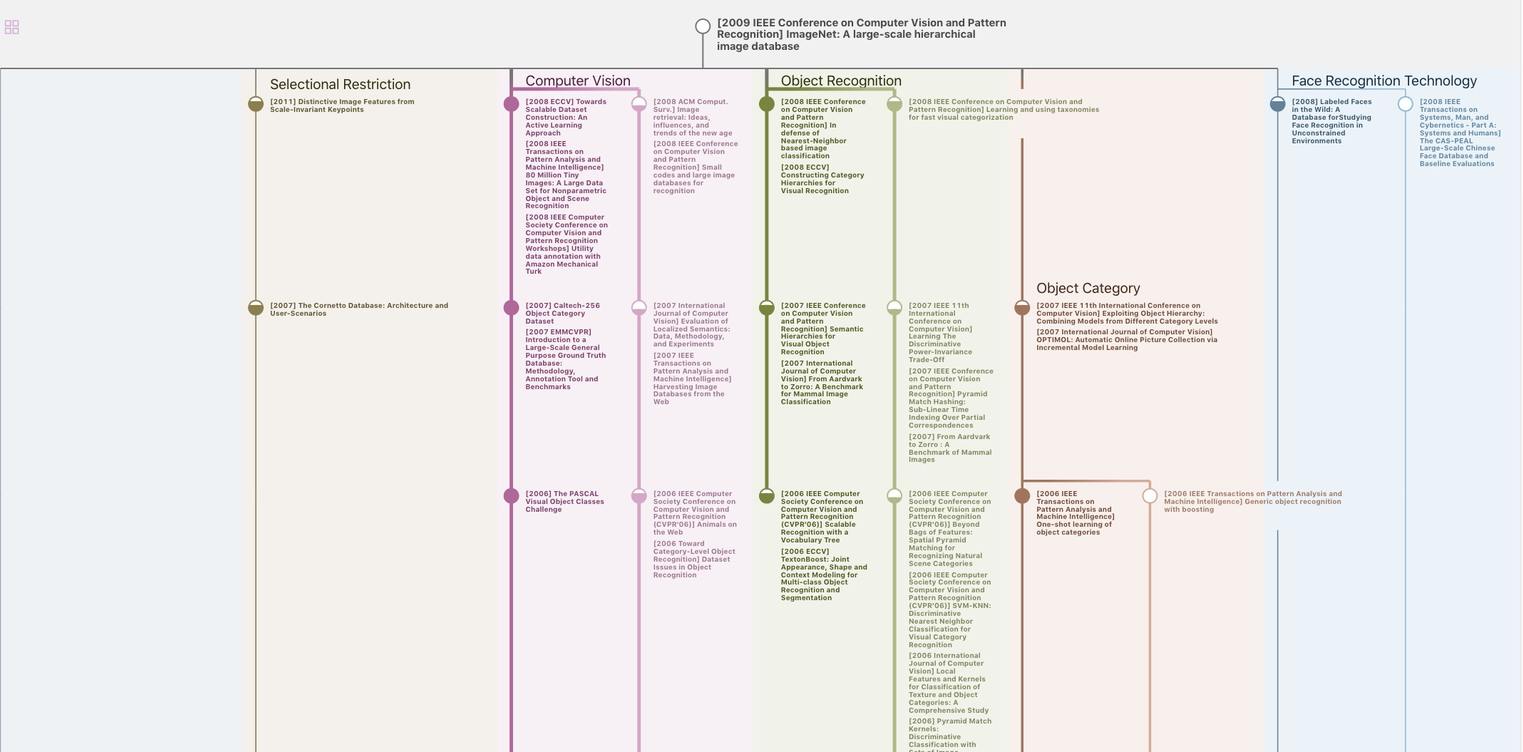
生成溯源树,研究论文发展脉络
Chat Paper
正在生成论文摘要