Provable Membership Inference Privacy
arxiv(2022)
摘要
In applications involving sensitive data, such as finance and healthcare, the necessity for preserving data privacy can be a significant barrier to machine learning model development. Differential privacy (DP) has emerged as one canonical standard for provable privacy. However, DP's strong theoretical guarantees often come at the cost of a large drop in its utility for machine learning, and DP guarantees themselves can be difficult to interpret. In this work, we propose a novel privacy notion, membership inference privacy (MIP), to address these challenges. We give a precise characterization of the relationship between MIP and DP, and show that MIP can be achieved using less amount of randomness compared to the amount required for guaranteeing DP, leading to a smaller drop in utility. MIP guarantees are also easily interpretable in terms of the success rate of membership inference attacks. Our theoretical results also give rise to a simple algorithm for guaranteeing MIP which can be used as a wrapper around any algorithm with a continuous output, including parametric model training.
更多查看译文
关键词
privacy
AI 理解论文
溯源树
样例
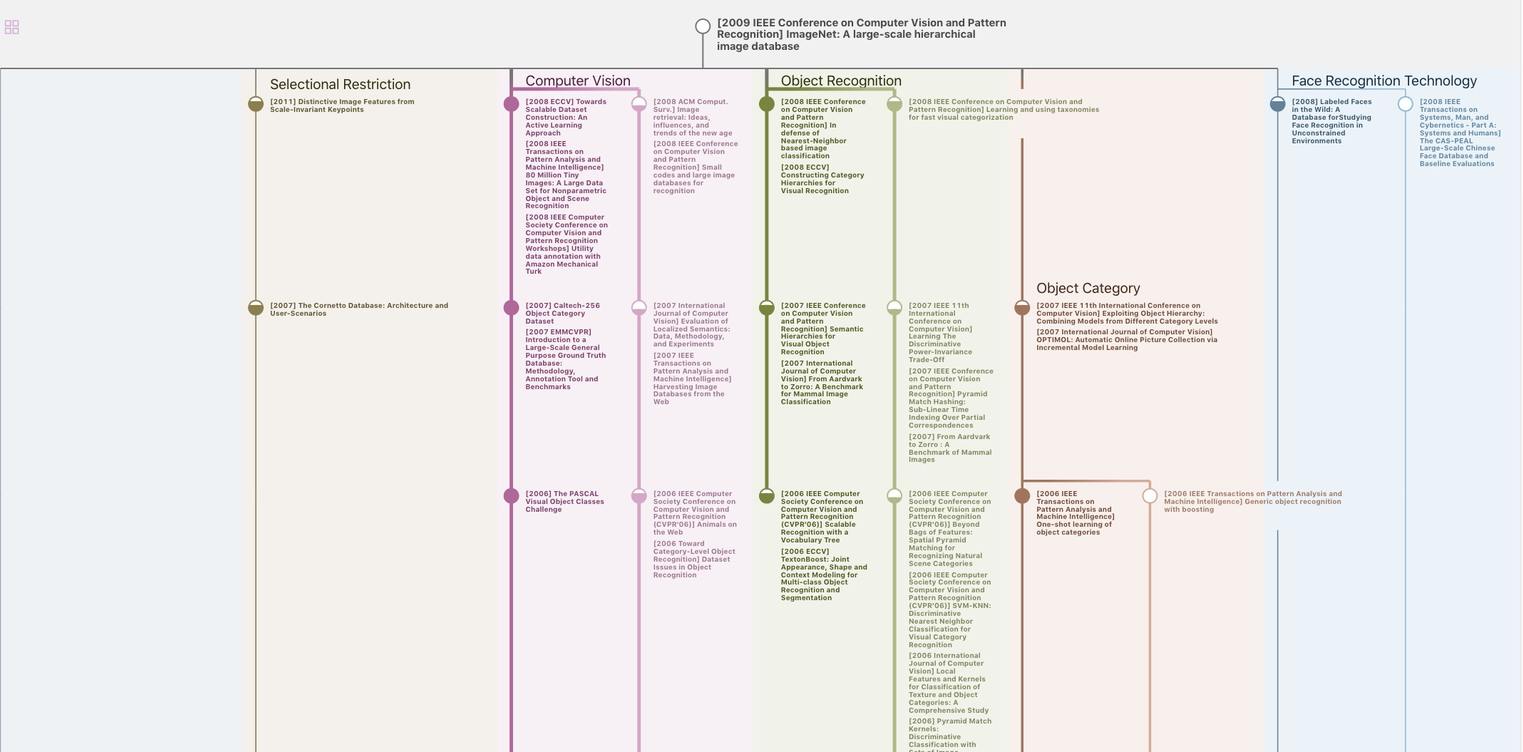
生成溯源树,研究论文发展脉络
Chat Paper
正在生成论文摘要