MicroISP: Processing 32MP Photos on Mobile Devices with Deep Learning
arxiv(2022)
摘要
While neural networks-based photo processing solutions can provide a better image quality compared to the traditional ISP systems, their application to mobile devices is still very limited due to their very high computational complexity. In this paper, we present a novel MicroISP model designed specifically for edge devices, taking into account their computational and memory limitations. The proposed solution is capable of processing up to 32MP photos on recent smartphones using the standard mobile ML libraries and requiring less than 1 second to perform the inference, while for FullHD images it achieves real-time performance. The architecture of the model is flexible, allowing to adjust its complexity to devices of different computational power. To evaluate the performance of the model, we collected a novel Fujifilm UltraISP dataset consisting of thousands of paired photos captured with a normal mobile camera sensor and a professional 102MP medium-format FujiFilm GFX100 camera. The experiments demonstrated that, despite its compact size, the MicroISP model is able to provide comparable or better visual results than the traditional mobile ISP systems, while outperforming the previously proposed efficient deep learning based solutions. Finally, this model is also compatible with the latest mobile AI accelerators, achieving good runtime and low power consumption on smartphone NPUs and APUs. The code, dataset and pre-trained models are available on the project website: https://people.ee.ethz.ch/~ihnatova/microisp.html
更多查看译文
关键词
Learned ISP, Computational photography, Mobile cameras, RAW Image Processing, Photo enhancement, Mobile AI, Deep learning, Efficient computer vision
AI 理解论文
溯源树
样例
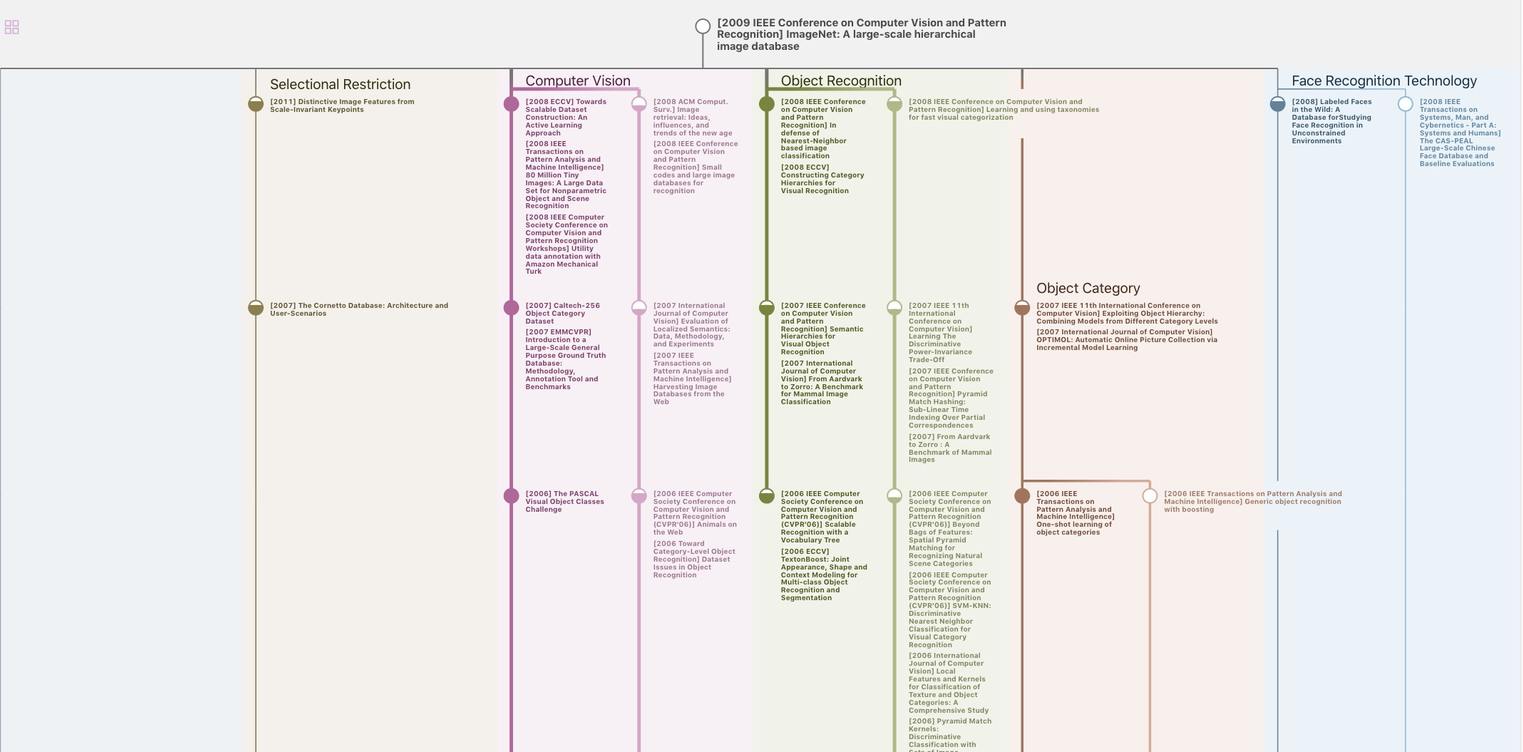
生成溯源树,研究论文发展脉络
Chat Paper
正在生成论文摘要