Inconsistency identification in network meta-analysis via stochastic search variable selection
arxiv(2022)
摘要
The reliability of the results of network meta-analysis (NMA) lies in the plausibility of key assumption of transitivity. This assumption implies that the effect modifiers' distribution is similar across treatment comparisons. Transitivity is statistically manifested through the consistency assumption which suggests that direct and indirect evidence are in agreement. Several methods have been suggested to evaluate consistency. A popular approach suggests adding inconsistency factors to the NMA model. We follow a different direction by describing each inconsistency factor with a candidate covariate whose choice relies on variable selection techniques. Our proposed method, Stochastic Search Inconsistency Factor Selection (SSIFS), evaluates the consistency assumption both locally and globally, by applying the stochastic search variable selection method to determine whether the inconsistency factors should be included in the model. The posterior inclusion probability of each inconsistency factor quantifies how likely is a specific comparison to be inconsistent. We use posterior model odds or the median probability model to decide on the importance of inconsistency factors. Differences between direct and indirect evidence can be incorporated into the inconsistency detection process. A key point of our proposed approach is the construction of a reasonable "informative" prior concerning network consistency. The prior is based on the elicitation of information derived historical data from 201 published network meta-analyses. The performance of our proposed method is evaluated in two published network meta-analyses. The proposed methodology is publicly available in an R package called ssifs, developed and maintained by the authors of this work.
更多查看译文
关键词
selection,network,search,meta-analysis
AI 理解论文
溯源树
样例
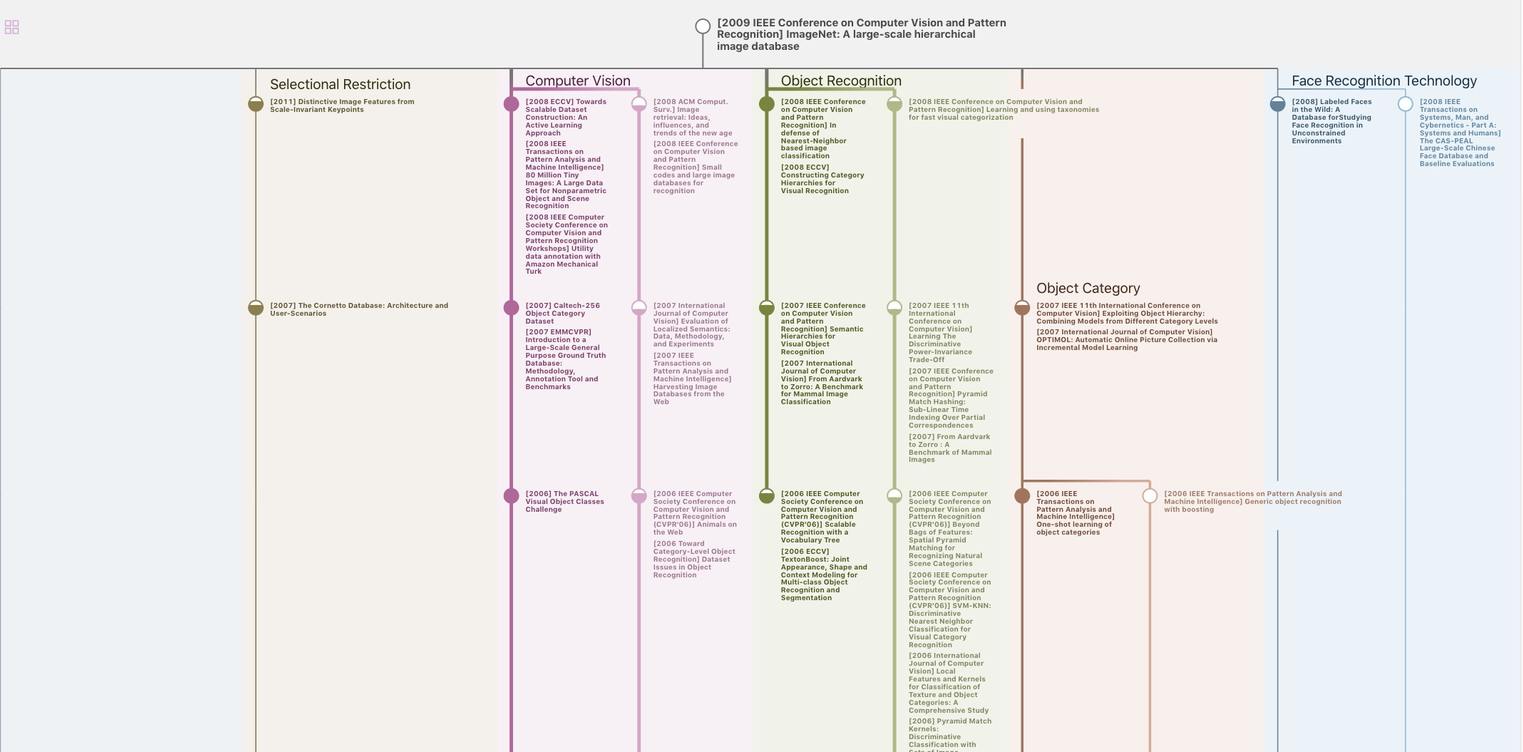
生成溯源树,研究论文发展脉络
Chat Paper
正在生成论文摘要