Fitting the BumpHunter test statistic distribution and global p-value estimation
arxiv(2022)
摘要
In high Energy Physics, it is common to look for a localized deviation in data with respect to a given reference. For this task, the well known BumpHunter algorithm allows for a model-independent deviation search with the advantage of estimating a global p-value to account for the Look Elsewhere Effect. However, this method relies on the generation and scan of thousands of pseudo-data histograms sampled from the reference background. Thus, accurately calculating a global significance of $5\sigma$ requires a lot of computing resources. In order to speed this process and improve the algorithm, we propose in this paper a solution to estimate the global p-value using a more reasonable number of pseudo-data histograms. This method uses a functional form inspired by similar statistical problems to fit the test statistic distribution. We have found that this alternative method allows to evaluate the global significance with a precision about 5% up to the $5\sigma$ discovery threshold.
更多查看译文
AI 理解论文
溯源树
样例
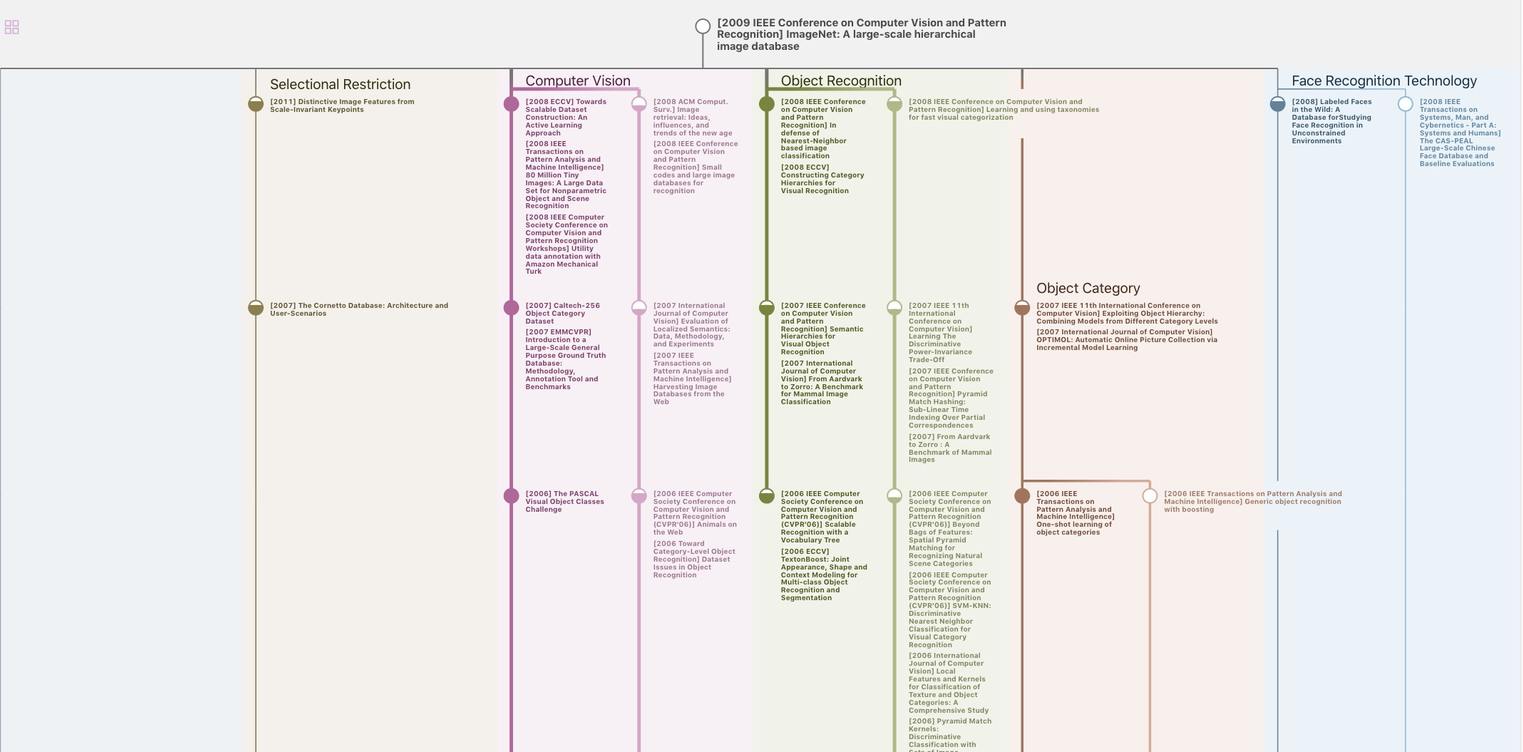
生成溯源树,研究论文发展脉络
Chat Paper
正在生成论文摘要