Group-Based Dimensionality Reduction and Estimation for Heterogeneous Large-Scale Traffic Networks
2022 European Control Conference (ECC)(2022)
摘要
State estimation for traffic networks is a particu-larly challenging problem in view of their large dimensionality, and since models are often inaccurate and the interaction pat-terns unpredictable. In this article, we approach the problem by mixing aggregation-based complexity reduction and nonlinear filtering. We subdivide vehicles into groups and derive a lower-dimensional approximate model where vehicles belonging to the same group are represented by a unique random variable matching their average characteristics. Then, we propose a procedure to estimate the statistical properties of the group variables from partial measurements. Connections to car-following models are discussed, and the developed methodology is illustrated through numerical simulations.
更多查看译文
关键词
aggregation-based complexity reduction,nonlinear filtering,lower-dimensional approximate model,unique random variable,group variables,group-based dimensionality reduction,heterogeneous large-scale traffic networks,numerical simulations
AI 理解论文
溯源树
样例
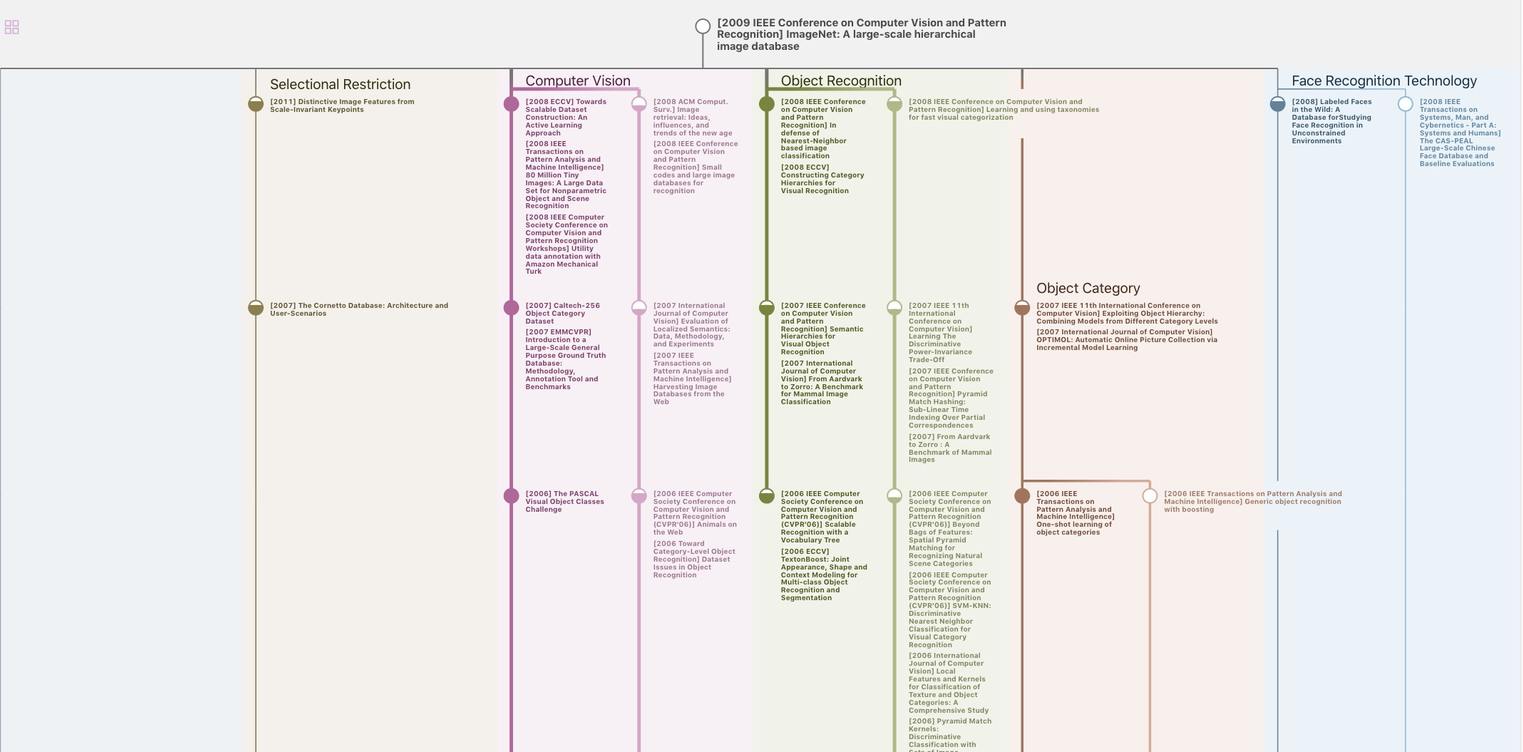
生成溯源树,研究论文发展脉络
Chat Paper
正在生成论文摘要